Unveiling the Potential of Machine Learning in Modern Technologies
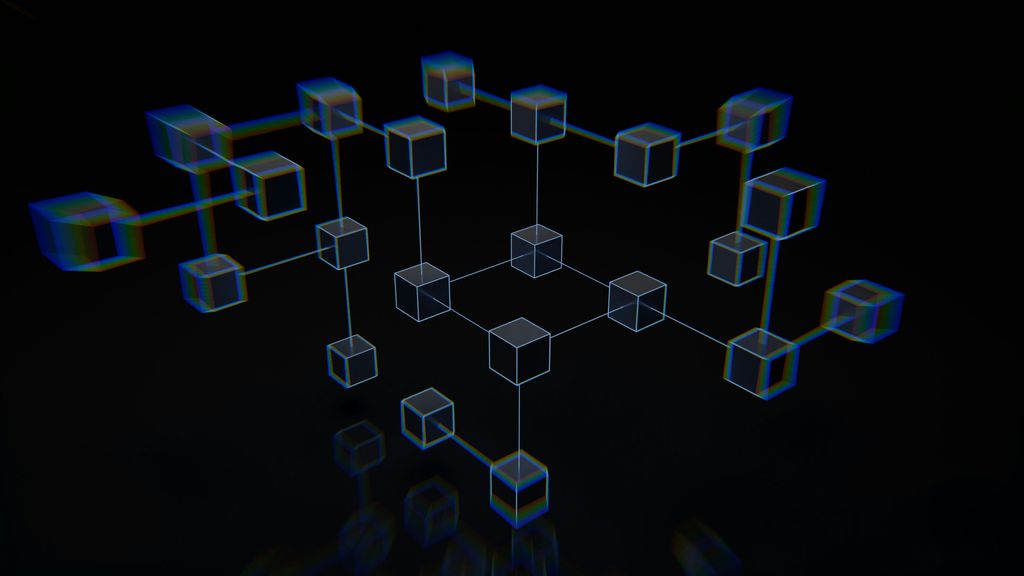
In today's rapidly advancing technological landscape, machine learning has emerged as one of the most prominent fields. From the early days when it was met with skepticism, machine learning has now become a powerful tool in various domains. This article aims to explore the intricacies of machine learning, with a specific focus on the role of possibility in developing AI systems. By understanding the significance of representation and reasoning, as well as the challenges posed by experience in machine learning, we can appreciate the potential of learning possibility in creating more advanced and efficient learning systems.
Key Takeaways
- Explore advancements in natural language processing, robotics, and quantum computing
- Understand the significance of representation and reasoning in machine learning
- Address the challenges posed by experience in machine learning
- Recognize the potential of learning possibility in creating advanced learning systems
- Appreciate the transformative power of intelligent machines
Unveiling the Impact of AI and ML Technologies
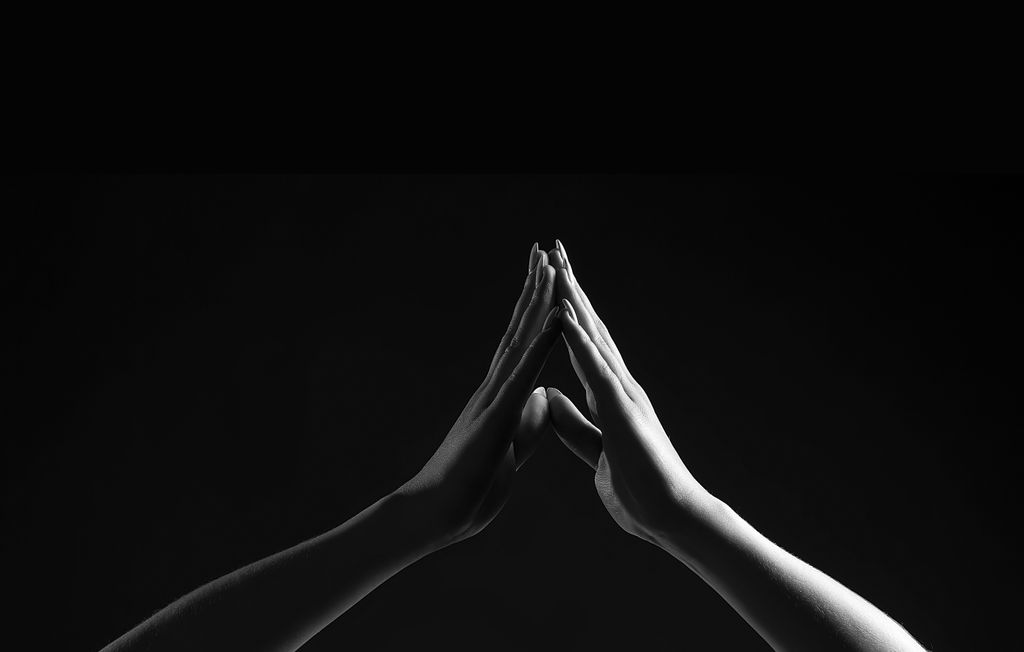
Exploring Advancements in Natural Language Processing
The field of Natural Language Processing (NLP) has seen transformative advancements, largely due to the integration of machine learning techniques. These innovations have significantly enhanced the ability of machines to understand and interpret human language, opening up new possibilities in how we interact with technology. For instance, the development of models like Google's BERT has been a game-changer, enabling machines to comprehend context and nuances in language with unprecedented accuracy.
Advancements in NLP are not just technical feats; they are reshaping the way we live and work. Here's a glimpse into the impact of these advancements:
- Improved language translation services
- More accurate sentiment analysis tools
- Personalized chatbots for enhanced user engagement
- In-depth analysis of text data for marketing and customer insights
The continuous refinement of NLP algorithms will further our ability to create machines that can engage in meaningful conversations and understand complex human emotions and intentions.
One of the key aspects of these advancements is the process of trial and error learning, where machines leverage feedback from previous actions and experiences to identify the best outcomes. Such an approach is crucial for the evolution of NLP, as it allows for the gradual improvement of algorithms over time.
Revolutionizing Robotics with AI and ML
Machine learning and artificial intelligence (AI) have revolutionized the field of robotics, just as robotics is poised to revolutionize the digital landscape. The synergy between AI and ML is amplifying the potential for groundbreaking advancements, transforming operations with a focus on efficiency and productivity.
Predictive maintenance, driven by AI and the Internet of Things (IoT), is a prime example of this transformation. It enhances equipment reliability and minimizes downtime, leading to significant improvements in manufacturing and other industrial domains.
The integration of AI and ML in robotics heralds a new era of innovation, where intelligent machines can learn, adapt, and work alongside humans to tackle complex tasks.
The economic implications are vast, with AI and ML contributing to the evolution of industries and everyday life. As we continue to explore the transformative impact of these technologies, it is clear that they are not just shaping the future of robotics but also redefining the boundaries of what is technologically possible.
Quantum Computing: Redefining Technological Boundaries
Quantum computing stands at the forefront of technological innovation, promising to revolutionize the way we solve complex problems. By leveraging the principles of quantum mechanics, these computers perform calculations at speeds unattainable by classical systems. The potential of quantum computing in enhancing machine learning algorithms is immense, offering unprecedented computational power to process vast datasets and perform intricate optimizations.
Quantum machine learning combines the best of both worlds, using quantum algorithms to accelerate the learning process. This synergy could lead to breakthroughs in various fields, from drug discovery to financial modeling. Here are some key areas where quantum computing could have a significant impact:
- Drug Discovery: Accelerating the identification of molecular structures and potential treatments.
- Climate Modeling: Providing more accurate simulations to predict climate change effects.
- Financial Modeling: Enabling complex risk assessments and market predictions with greater precision.
The integration of quantum computing with machine learning not only pushes the boundaries of what's computationally possible but also opens new avenues for innovation and problem-solving.
As we continue to explore the capabilities of quantum computing, it is crucial to address the challenges that come with it, such as the need for specialized hardware and the development of new algorithms. The journey towards fully harnessing the power of quantum computing is just beginning, and its convergence with machine learning is set to redefine our technological limits.
The Evolution of Machine Learning
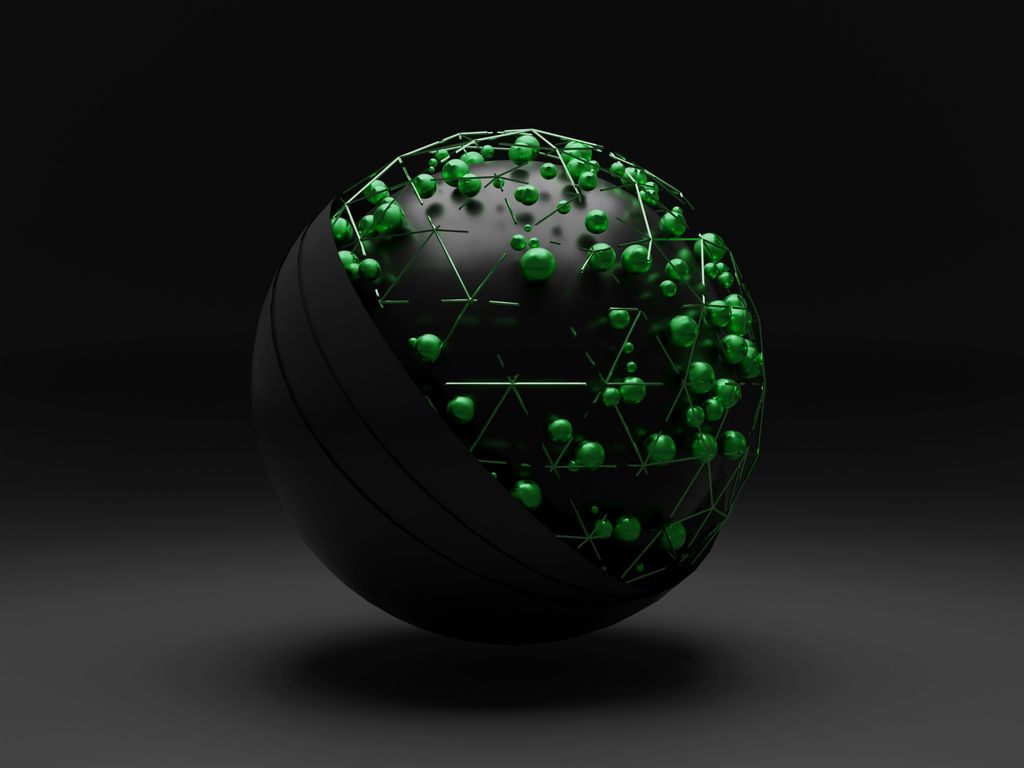
From Skepticism to Power: A Historical Perspective
The journey of machine learning from a concept met with skepticism to a powerful force in technology is a testament to human ingenuity. Machine learning has evolved to impact society in ways we are just beginning to fathom. This evolution is marked by key milestones that reflect the growth of computing power and the availability of data.
- The inception of the idea in the mid-20th century
- The first algorithms and their practical applications
- The AI winter and subsequent resurgence
- The explosion of data and computational resources in the 21st century
Machine learning is not a stagnant concept but a self-evolving system that adapts and contributes to our technological landscape.
As we continue to navigate the ongoing evolution of AI and ML, it's clear that their transformative impact on society goes beyond industries, influencing everything from transportation to ethics. The balanced perspective we now have allows us to appreciate the full spectrum of machine learning's capabilities.
Machine Learning's Role in Various Domains
The versatility of machine learning (ML) is evident across a spectrum of industries, proving its capacity to not only enhance existing technologies but also to pioneer new ones. Machine learning helps devices program themselves, adapting to new conditions with a level of autonomy that was once the realm of science fiction. From robotics to autonomous vehicles, and from social networks to predictive analytics, ML's role is pivotal in shaping the future of technology.
- Robotics: Managing uncertainty and improving adaptability in dynamic environments.
- Autonomous Vehicles: Enabling self-driving cars to make intelligent decisions.
- Social Networks: Analyzing relationships and preferences to tailor user experiences.
- Predictive Analytics: Shaping business strategies through data-driven insights.
The synergy between artificial intelligence (AI) and ML is unleashing unprecedented possibilities, where AI provides the overarching intelligence, and ML refines its capabilities through continuous learning. This symbiosis is not only fueling innovation but also streamlining decision-making processes across various sectors.
The impact of ML on society and everyday life is profound, with applications that touch upon nearly every aspect of our daily routines. The potential of learning possibility in developing AI systems is a testament to the transformative power of ML, as it continues to redefine the boundaries of what is technologically achievable.
Advancements in Computing Power and Dataset Availability
The landscape of machine learning has been transformed by significant advancements in computing power and the proliferation of large datasets. These two factors have synergistically fueled the capabilities of machine learning algorithms, enabling them to perform complex tasks with remarkable accuracy and efficiency.
Computing power has seen exponential growth, allowing for the training of more sophisticated neural networks that can process and analyze vast amounts of data. This has been pivotal in the development of technologies that can learn and adapt with minimal human intervention.
The availability of extensive datasets has been equally crucial. Historical and real-time data provide the raw material for machine learning models to learn and improve. The following list highlights the importance of data in machine learning:
- Data is the foundation upon which machine learning models are built.
- Quality and quantity of data directly influence the accuracy of predictions.
- Diverse datasets ensure that models are robust and less biased.
- Continuous data flow allows for real-time learning and adaptation.
The symbiosis of advanced computing power and extensive datasets has not only accelerated the pace of innovation but also democratized the use of machine learning across various domains. The future of technology hinges on our ability to harness and interpret data, making these advancements not just valuable, but essential for progress.
Empowering AI Systems with Machine Learning
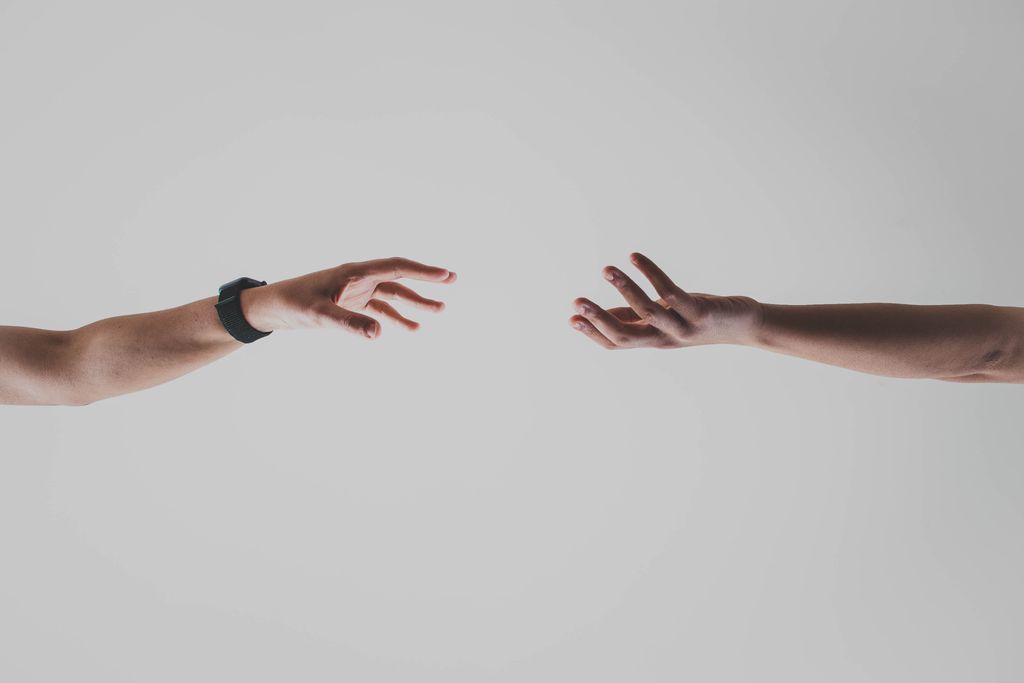
Training Agents for Decision-Making
In the realm of artificial intelligence, the ability to train agents for effective decision-making stands as a cornerstone of progress. Machine learning algorithms empower these agents to learn from data, adapt to new situations, and make informed decisions. This process is often achieved through reinforcement learning, where an AI agent learns through trial and error, honing its strategy over time.
Reinforcement learning is particularly significant in areas such as finance and content creation. For instance, in trading, ML algorithms can analyze historical data to develop and refine trading strategies, adapting to market changes to optimize performance. Similarly, in content creation, algorithms can adjust based on user engagement, striving to maximize conversions.
The iterative nature of machine learning in decision-making allows for continuous improvement, as agents learn to navigate complex environments and achieve specific goals.
The impact of machine learning on decision-making is not limited to a single domain but spans various industries:
- Finance: Enhancing trading strategies and risk assessment.
- Content Creation: Tailoring content to user preferences and feedback.
- Customer Service: Automating responses and personalizing interactions.
By leveraging machine learning, organizations can equip their AI systems to act more autonomously and efficiently, paving the way for innovative solutions and competitive advantages.
Addressing Ethical Considerations
The integration of AI and ML into our digital ecosystem has raised significant ethical questions. Ethics and effectiveness are undeniably linked to the data quality they process. To navigate these waters with precision and responsibility, implementing strict data governance practices is a start. Furthermore, collaboration between regulatory bodies, tech companies, and other stakeholders is crucial.
Ensuring data accuracy is another pivotal aspect. The risk of misinformation or outdated information can skew analytics and predictions, leading to flawed decision-making. Advanced algorithms and machine learning models have evolved to become more discerning and nuanced, ensuring that data adheres to privacy and ethical standards.
It is about balancing the scale where technological innovations address the gaps and challenges associated with harnessing public web data effectively and ethically.
In conclusion, fostering trust and reliability in AI and ML applications is essential. Crafting universal standards and regulations that cater to the dynamic nature of data, technology, and privacy can pave the way for a balanced and sustainable progression.
Ensuring Equitable Applications of AI and ML
The integration of Artificial Intelligence (AI) and Machine Learning (ML) into various sectors has the potential to streamline workflows and revolutionize industries. However, ensuring equitable applications of these technologies is a multifaceted challenge that requires careful consideration of ethical norms and the socio-economic landscape.
Ethical considerations are paramount when addressing the challenges of bias in AI algorithms. It is crucial to implement strict data governance practices to maintain the integrity and fairness of AI systems. A collaborative approach involving regulatory bodies, tech companies, and stakeholders is essential to craft universal standards and regulations.
The methodologies employed to collect, process, and utilize data are under scrutiny. Ensuring that data extraction adheres to ethical norms is essential to fostering trust and reliability in AI and ML applications.
To further illustrate the importance of equitable AI, consider the economic implications of these technologies:
- Automation in industries may lead to efficiency gains but also societal challenges.
- The creation of new job sectors must be balanced with the potential displacement of traditional jobs.
- Navigating the balance between technological advancement and societal impact is key to sustainable progression.
The Future of Machine Learning and Possibility
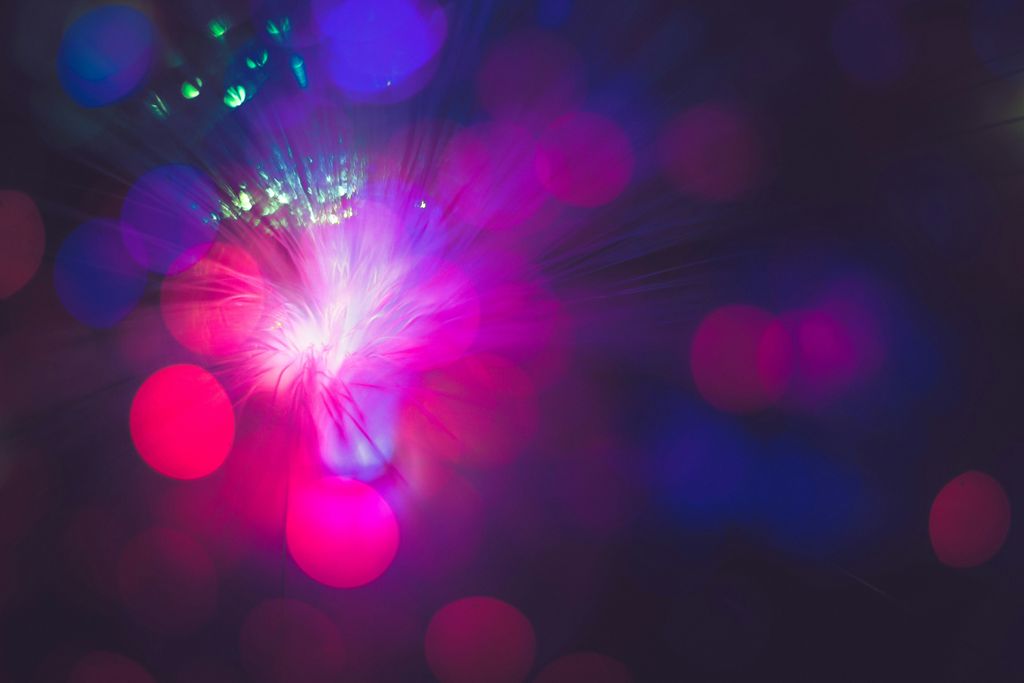
Representation and Reasoning in Machine Learning
At the core of machine learning is the ability to represent and reason with data. Representation refers to how information is encoded and stored, allowing machines to interpret and make sense of it. Reasoning, on the other hand, involves applying logical and probabilistic rules to draw conclusions or make predictions based on the available data. Effective representation and reasoning are crucial for machine learning systems to accurately process information and make informed decisions.
Incorporating rules and reasoning in machine learning systems is essential to ensure consistency and logical coherence. Rule-based systems allow machines to encode domain-specific knowledge and constraints, guiding their decision-making process. By combining rules with learning algorithms, we can create hybrid systems that are capable of both learning from data and reasoning with logical rules. These systems provide a powerful framework for handling complex tasks that require both data-driven learning and knowledge-based reasoning.
Modern machine learning approaches can be categorized into two main types: Supervised learning and unsupervised learning. In supervised learning, an algorithm is trained on a labeled dataset, learning to make predictions or decisions based on that data. Unsupervised learning, however, deals with finding patterns and relationships in unlabeled data.
The future of machine learning also hinges on the ability to deal with uncertainty and flexibility in decision-making. Possibility plays a fundamental role in this aspect, enhancing the adaptability and robustness of intelligent systems. As we continue to develop and refine possibility-based learning algorithms, we can expect machine learning systems to become more adept at handling the inherent uncertainties of the real world.
Modern Approaches in Machine Learning
The landscape of machine learning is continually evolving, with modern approaches significantly enhancing the capabilities of AI systems. Supervised and unsupervised learning remain foundational techniques, but the emergence of reinforcement learning has opened new avenues for machines to learn from interaction with their environment. This approach, akin to learning through trial and error, is pivotal for systems requiring autonomous decision-making.
Modern machine learning is not just about algorithms, but also about the ability to adapt and learn from new experiences. Transfer learning and meta-learning are methods addressing the 'problem of experience' by enabling models to apply knowledge gained in one domain to another, thus accelerating the learning process.
Collaboration among industry leaders, such as the partnership between Boeing and other companies, exemplifies the cooperative spirit driving innovation. This synergy is crucial for tackling complex challenges and pushing the boundaries of what machine learning can achieve.
- Representation and reasoning in machine learning
- Consistency with rules and reasoning
- The potential of learning possibility in AI development
As we look to the future, the integration of machine learning with other emerging technologies like quantum computing could redefine our technological limits, offering unprecedented possibilities for advancement.
Overcoming the Problem of Experience
Machine learning systems thrive on vast amounts of data, but acquiring this data can be a significant hurdle. The challenge lies in not just collecting data, but in ensuring its quality and relevance. To address this, researchers are exploring innovative methods to reduce reliance on extensive historical data.
One approach is the use of synthetic data, which can be generated to simulate real-world scenarios. This allows ML models to learn from a wider range of experiences without the need for large datasets from the real world. Another strategy is transfer learning, where a model trained on one task is adapted to perform another related task, effectively leveraging prior knowledge.
The goal is to create systems that can learn more efficiently from less data, making machine learning more accessible and less resource-intensive.
The following list outlines key strategies to overcome the experience problem in machine learning:
- Utilizing synthetic data to augment real datasets
- Applying transfer learning to share knowledge across tasks
- Implementing few-shot learning techniques for rapid adaptation
- Exploring unsupervised and semi-supervised learning methods
- Encouraging the development of algorithms that require less data for training
By adopting these strategies, the field of machine learning can progress towards models that are not only powerful but also practical for a wider range of applications.
Cooperation for Innovation and Advancement
In the realm of machine learning, cooperation is not just beneficial; it's essential. Companies like Boeing exemplify this by leading collaborations that harness the collective power of AI to drive innovation in the aerospace industry. Sharing data, expertise, and resources, these partnerships are pivotal in creating AI systems that enhance safety and efficiency.
Collaboration is the cornerstone of machine learning research, with its ability to transcend industry boundaries and foster transformative advancements. The synergy between different organizations facilitates a melting pot of ideas, leading to breakthroughs that might not be possible in isolation.
- Predictive maintenance with AI and IoT
- Enhancing equipment reliability
- Minimizing operational downtime
The collaborative nature of machine learning is a testament to its potential in revolutionizing not just the aerospace sector, but various industries, paving the way for a future where AI-driven solutions are the norm.
Conclusion
In conclusion, the integration of Artificial Intelligence and Machine Learning heralds a new era in the modern age. As we decode the intricate layers of these technologies, their impact on industries, society, and the future becomes increasingly apparent. The journey into tomorrow is shaped by the relentless pursuit of innovation and the transformative power of intelligent machines.
Frequently Asked Questions
What is the potential impact of AI and ML technologies in modern technologies?
AI and ML technologies have the potential to revolutionize industries and society, reshaping the future with advanced capabilities.
How has machine learning evolved over time?
Machine learning has transitioned from skepticism to power, becoming a crucial tool in various domains with advancements in computing power and dataset availability.
How can AI systems be empowered with machine learning?
AI systems can be empowered through training agents for decision-making, addressing ethical considerations, and ensuring equitable applications of AI and ML.
What is the future outlook for machine learning and its possibilities?
The future of machine learning involves representation and reasoning, modern approaches, overcoming the problem of experience, and cooperation for innovation and advancement.
What are the key advancements in machine learning for smarter solutions?
Advancements in machine learning for smarter solutions include training agents for decision-making, addressing ethical considerations, and ensuring equitable applications of AI and ML.
How can machine learning contribute to improving accuracy in AI content detectors?
Machine learning plays a crucial role in enhancing the accuracy of AI content detectors by utilizing advanced algorithms and statistical models.