Smart Chemistry: Exploring AI Applications in Chemicals
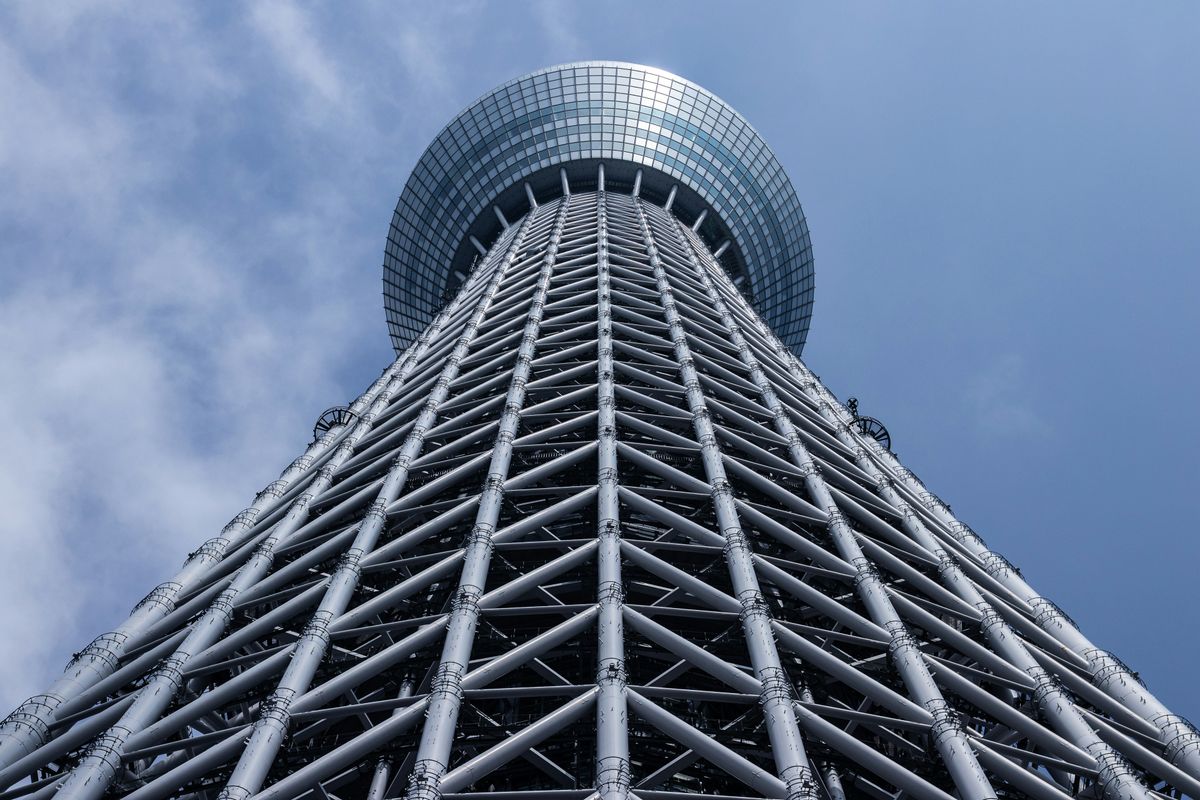
In the ever-evolving landscape of scientific research, the infusion of artificial intelligence (AI) stands out as a transformative force, particularly within the realm of chemistry. As technology progresses, AI applications are reshaping conventional approaches to chemical research, introducing novel avenues and hastening the pace of discovery. This article explores the profound influence of AI on chemistry research and delves into the exciting developments that are shaping the future of this field.
Key Takeaways
- AI is revolutionizing drug discovery by accelerating the identification of new compounds and enhancing predictive models for drug efficacy, leading to more efficient clinical trials.
- Innovations in materials science are being driven by AI, with the development of smart and sustainable materials and the customization of their properties through machine learning.
- Chemical manufacturing processes are being optimized using AI to improve efficiency, implement predictive maintenance, and enhance quality control and assurance.
- AI applications are addressing environmental and safety concerns by predicting chemical hazards, reducing environmental impact, and enhancing lab safety with monitoring systems.
- The future of chemical research is being shaped by generative AI, which is aiding in novel chemical synthesis, the design of next-generation catalysts, and altering industry dynamics.
Revolutionizing Drug Discovery with AI
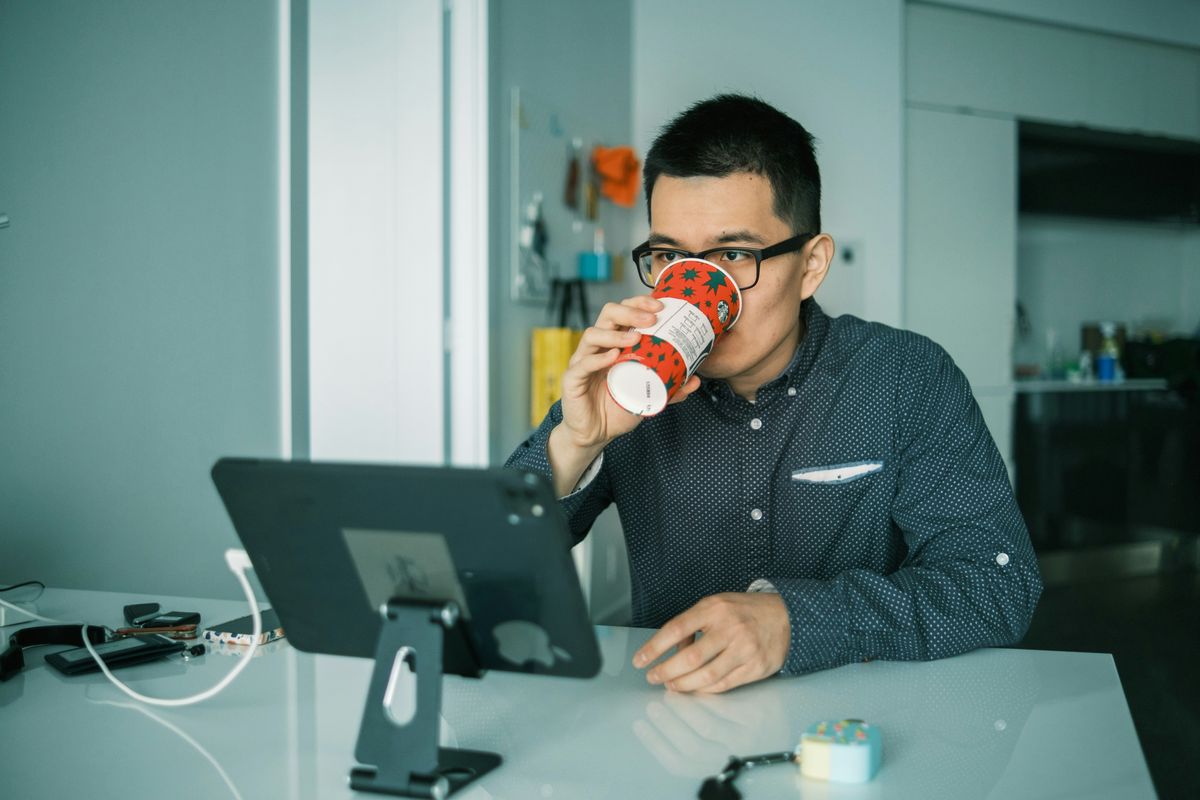
Accelerating the Identification of New Compounds
The advent of artificial intelligence (AI) has brought a paradigm shift in the pharmaceutical industry, particularly in the identification of new compounds. AI-driven approaches are significantly reducing the time and resources required to discover potential drug candidates. Traditional methods, such as high-throughput screening, are known for being laborious and time-consuming. AI, on the other hand, can rapidly analyze vast chemical libraries, pinpointing molecules with desired properties more efficiently.
Predictive analytics play a crucial role in this process, enabling researchers to forecast bioactivity and optimize compound activity without the need for extensive physical testing. This not only accelerates the discovery phase but also enhances the accuracy of the results. The integration of AI in drug discovery pipelines is a testament to how technology is revolutionizing the field, addressing both the challenges of speed and precision.
AI revolutionizes drug discovery and clinical trials in healthcare, enhancing personalized treatments and predictive analytics.
Despite the clear advantages, the industry faces hurdles such as ethical considerations and resistance to AI adoption. It is essential to navigate these challenges thoughtfully to fully harness AI's potential in drug discovery.
Enhancing Predictive Models for Drug Efficacy
The integration of AI in pharmacology has led to significant advancements in predictive modeling for drug efficacy. Machine learning algorithms have shown promise in identifying patterns and correlations within vast datasets, leading to more accurate predictions of a drug's performance. For instance, studies utilizing sources like DrugBank have achieved an average of 79% accuracy for predicting safe versus risky drugs, indicating the potential of AI in enhancing drug safety profiles.
Predictive analytics in pharmacokinetics and pharmacodynamics are becoming increasingly sophisticated. Researchers are harnessing machine learning to anticipate drug-drug interactions and to forecast pharmacokinetic parameters with notable success. This not only accelerates the drug development process but also improves the precision of therapeutic interventions.
The ability to predict drug efficacy accurately is crucial for reducing the time and cost associated with bringing new medications to market.
The table below illustrates the predictive accuracy achieved in recent studies:
Study Reference | Prediction Focus | Accuracy |
---|---|---|
Webel et al. | Cytotoxicity | >70% |
Keutzer et al. | Pharmacokinetics | 79% |
Cheng et al. | Drug Interactions | 76% |
These advancements underscore the transformative impact of AI on drug discovery, paving the way for more effective and safer medications.
Streamlining Clinical Trials with AI Integration
The integration of AI into clinical trials is transforming the landscape of drug development. Artificial intelligence is streamlining the entire clinical trial process, from patient recruitment to data analysis. By leveraging AI algorithms, researchers can now more efficiently identify eligible patients, which in turn saves testing expenses and accelerates therapy approval. This is particularly evident in the use of genetic profiles, biomarkers, and electronic health information to tailor clinical trials to individual patient needs.
AI's capability to process massive volumes of data is crucial in addressing complicated clinical issues. With tools like IBM Watson for oncology and PathAI for pathology diagnosis, AI is enhancing the precision of clinical trials. Moreover, the adoption of wearable devices and sensors facilitates the collection of real-world data, which AI algorithms can analyze to provide customized treatment programs and improve patient adherence.
The potential of AI in medicine extends beyond clinical trials, offering innovative solutions for drug discovery and patient care.
The impact of AI on clinical trials is quantifiable. McKinsey Global Institute predicts a fundamental change in society due to AI-driven automation, which includes the reduction of time, cost, and failure rates in clinical trials. The following list highlights the key benefits of AI integration in clinical trials:
- Accelerated patient recruitment and selection
- Enhanced design of clinical trials through insightful data analysis
- Improved treatment customization and patient adherence
- Reduced time and cost of research
- Lower failure rates of clinical trials
AI-Driven Innovations in Materials Science
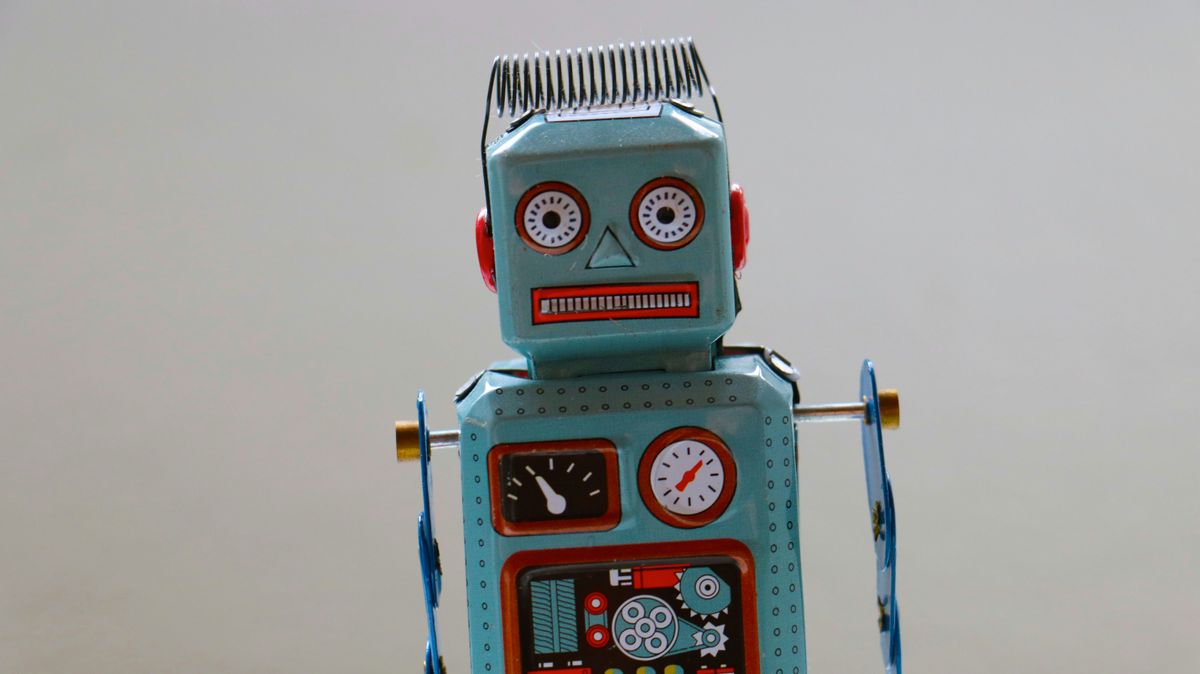
Advancing Smart Materials with AI Algorithms
The integration of AI in materials science has led to significant strides in the design and development of smart materials. AI algorithms are now essential tools in predicting and optimizing the properties of new materials, which is crucial for applications ranging from energy storage to environmental sustainability. For instance, machine learning models are adept at analyzing the complex relationships between material structures and their performance, guiding researchers to engineer materials with desired functionalities.
The potential of AI in this field is vast, with implications for both industry and environmental stewardship. As AI revolutionizes mining with smarter extraction and exploration, similar advancements in AI-driven geological analysis and smart mining technologies are being mirrored in the materials sector to optimize operations and promote sustainability.
By harnessing the power of AI, the scope of challenges that scientists can address is expanding, allowing for more targeted and efficient material innovation.
Here are some of the benefits that AI brings to the table in materials science:
- Sourcing optimized material compositions for enhanced product performance.
- Accelerating the discovery of novel materials for applications like carbon capture.
- Reducing equipment downtime through predictive maintenance strategies.
AI in the Development of Sustainable Materials
The integration of AI in materials science is not just about innovation; it's about sustainability. AI-driven systems optimize fertilizer application, revolutionize recycling with advanced sorting, and enhance energy management for efficiency and sustainability in agriculture and recycling sectors. By harnessing the power of AI, scientists are able to design materials that are not only high-performing but also environmentally friendly.
One of the most promising areas is the development of metal-organic framework (MOF) materials. These versatile compounds are being explored for a range of applications, from catalysts to energy converters, with AI playing a pivotal role in identifying new MOFs suitable for carbon capture. This is crucial in the fight against climate change, as these materials can significantly reduce greenhouse gas emissions.
The potential of AI to transform the materials industry is immense, offering a path to more sustainable and efficient material design and usage.
The table below outlines some of the key benefits of AI in sustainable material development:
Benefit | Description |
---|---|
Enhanced Product Performance | AI sources optimized material compositions. |
Predictive Maintenance | Reduces equipment downtime through AI strategies. |
Eco-friendly Manufacturing | AI fosters sustainable processes and novel material discovery. |
Customizing Properties of Materials Using Machine Learning
The advent of AI in materials science has ushered in a new era of optimizing material design. Machine learning models are pivotal in understanding the intricate relationship between material structures and their performance. This understanding is crucial for developing materials with desired properties, which has significant implications for industries like energy storage and catalysis.
The process of employing machine learning in materials research involves several key steps:
- Data preparation, including cleaning and imputing missing values.
- Feature extraction and selection of key attributes.
- Algorithm selection and model development.
- Evaluation and refinement of the predictive model.
By harnessing machine learning, researchers can drastically reduce the time and resources needed to develop high-performance materials. This not only accelerates the discovery process but also contributes to tackling global challenges such as climate change through improved materials for gas separation and energy conversion.
The scope of machine learning in materials research is vast, with reviews indicating that it reshapes our comprehension and manipulation of materials. It enables the tailored design of materials through property prediction, leading to breakthroughs in various applications.
Optimizing Chemical Manufacturing with AI
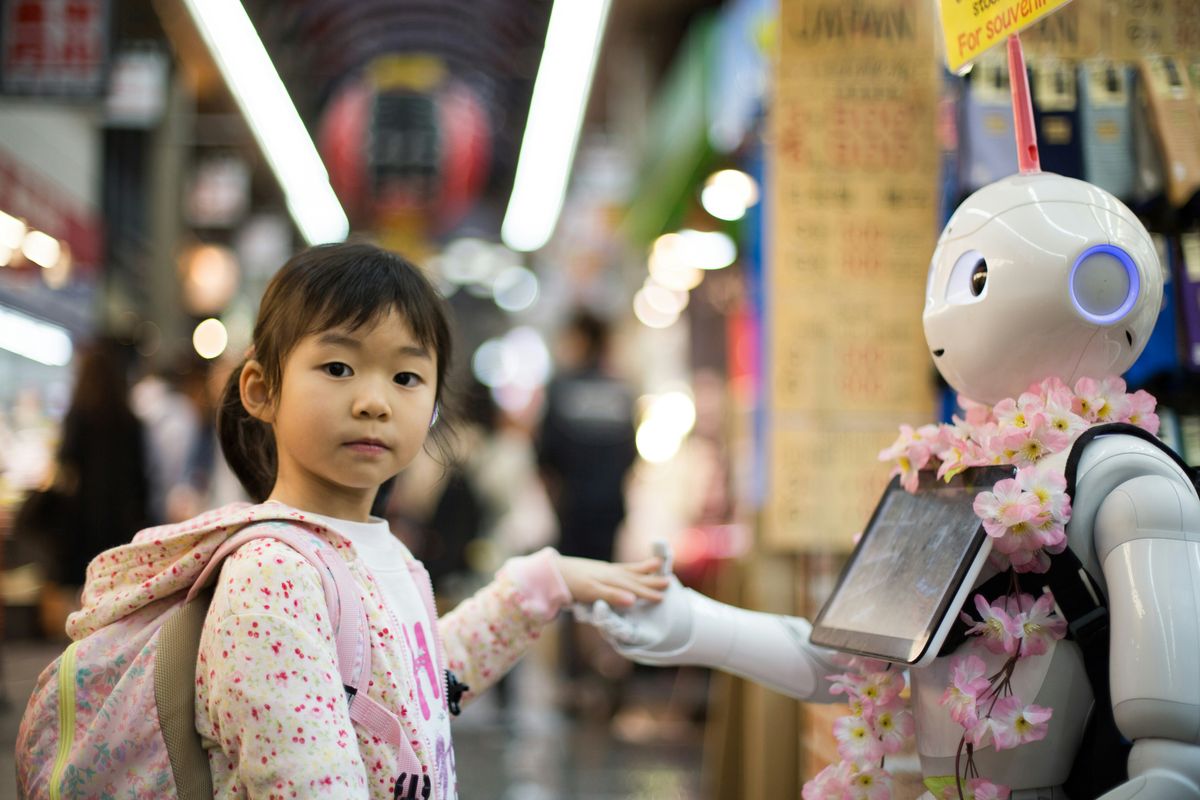
Improving Process Efficiency Through AI Automation
The integration of AI into pharmaceutical operations is a game-changer, optimizing data usage and enhancing decision-making. By automating processes and employing real-time analytics, companies are witnessing a significant boost in productivity and quality. This shift is not only reshaping industry operations but also ensuring higher resource utilization and cost-effectiveness.
AI in pharmaceutical digitalization is pivotal for maintaining a competitive edge. It streamlines the production process, reducing time to market and improving product safety. However, the journey to full-scale implementation comes with its challenges, as the industry navigates the complexities of integrating AI with existing systems.
The promise of AI automation lies in its ability to transform every facet of pharmaceutical manufacturing, from the lab to the market.
The impact of AI on the workforce is also noteworthy. As AI systems take over repetitive tasks, there is a growing need for skilled workers to guide these advanced processes, potentially leading to an increase in job opportunities. Smart lab instruments and robotics, enhanced with AI, are setting new standards for efficiency in research and development, making laboratories smarter and more productive.
Predictive Maintenance in Chemical Plants
Predictive maintenance in chemical plants is a game-changer for the industry, offering a proactive approach to equipment management. By utilizing advanced sensors and IoT solutions, plants can monitor the condition of machinery in real-time, anticipating failures before they occur. This not only enhances the reliability of operations but also significantly reduces unplanned downtime.
Predictive maintenance emerges as a transformative solution, addressing key issues manufacturers face with complex processes and the potential for equipment disruptions. The integration of AI into maintenance strategies allows for the continuous analysis of data, leading to more accurate predictions and timely interventions.
The strategic implementation of predictive maintenance can lead to substantial cost savings and improved operational efficiency. It is a critical component in the modernization of chemical manufacturing.
Key benefits of predictive maintenance include:
- Extended equipment lifespan
- Optimized maintenance schedules
- Reduction in maintenance costs
- Increased safety and reduced risk of accidents
- Enhanced production quality
AI in Quality Control and Assurance
In the realm of chemical manufacturing, quality control and assurance are critical for ensuring product consistency and safety. AI systems are transforming this domain by introducing advanced analytics and machine learning models that can predict and detect quality deviations in real-time. This proactive approach not only reduces waste but also minimizes the risk of releasing substandard products.
AI-driven tools are adept at analyzing complex datasets, which are often beyond the scope of traditional statistical methods. By leveraging historical and real-time data, AI can identify patterns and anomalies that might indicate potential quality issues. For instance, AI-driven production yield prediction is a game-changer, allowing manufacturers to anticipate and rectify problems before they affect the final product.
The integration of AI in quality control processes is not just about preventing defects; it's about striving for excellence in every batch produced.
The benefits of AI in this field are manifold, including improved accuracy, faster response times, and enhanced traceability. Here's a brief overview of how AI contributes to quality assurance:
- Automated visual inspections: AI algorithms can analyze images from cameras on the production line to detect defects that are imperceptible to the human eye.
- Predictive analytics: By forecasting potential quality issues, AI helps in taking preemptive measures to avoid them.
- Process optimization: Machine learning models can suggest adjustments to the manufacturing process to maintain high-quality standards.
- Data-driven decision-making: AI provides actionable insights that lead to more informed decisions regarding quality control.
AI in Environmental and Safety Applications
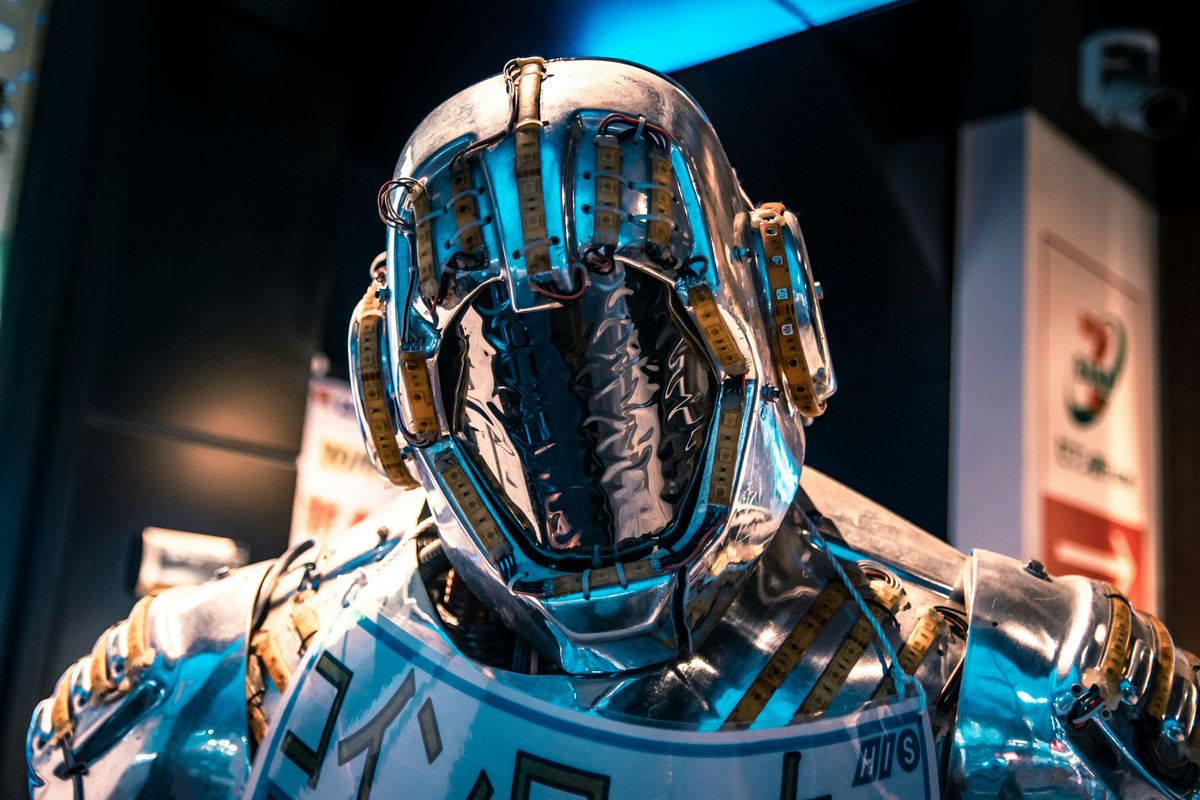
AI for Predicting Chemical Hazards
The integration of AI in chemical research has marked a significant advancement in predicting chemical hazards. AI's predictive capabilities are crucial for anticipating potential risks and formulating preemptive measures. By analyzing vast datasets, AI algorithms can identify patterns and correlations that human experts may overlook, leading to more accurate hazard assessments.
Toxicity prediction is a prime example of AI's impact in this domain. Utilizing deep learning techniques, such as DeepTox, AI systems can evaluate the safety profile of substances with remarkable precision. This foresight is instrumental in preventing adverse effects during the development of new drugs and chemicals.
- Predictive modeling for reaction outcomes
- AI-based toxicity prediction
- Mitigating risk through AI
AI's role in mitigating environmental and safety concerns is pivotal. It not only aids in reducing the environmental impact of chemical processes but also enhances laboratory safety by recommending safer alternatives.
Reducing Environmental Impact with AI-Optimized Processes
The integration of Artificial Intelligence (AI) in chemical engineering is transforming the way environmental impacts are mitigated. AI algorithms are instrumental in optimizing processes to reduce waste and emissions, ensuring a greener approach to chemical manufacturing. By leveraging machine learning, AI systems can predict and adjust operational parameters in real-time, leading to more sustainable outcomes.
- AI-driven process optimization minimizes resource consumption.
- Machine learning models facilitate the reduction of hazardous byproducts.
- Intelligent algorithms enhance energy efficiency across operations.
AI's capability to analyze vast amounts of data and recommend eco-friendly alternatives is crucial for sustainable chemical engineering practices.
While AI's role in optimizing processes for reduced environmental impact is clear, the practical application of these technologies is still evolving. The industry is witnessing a gradual shift towards more AI-integrated systems, which promise to deliver not only environmental benefits but also economic advantages through improved efficiency and resource utilization.
Enhancing Laboratory Safety with AI Monitoring Systems
The integration of AI into laboratory safety protocols marks a significant advancement in chemical research. Smart lab instruments and robotics, equipped with AI, are transforming laboratories into more efficient and safer environments. These instruments automate repetitive tasks, analyze experimental data in real-time, and suggest next steps, thereby reducing the risk of human error and exposure to hazardous substances.
Toxicity prediction is another area where AI is making strides. By leveraging vast datasets, AI can predict the side effects and in vivo safety of chemicals before they are manufactured. This preemptive approach to safety is revolutionizing how researchers handle toxic substances, ensuring that safety information is verified early in the drug development process.
AI's role in addressing environmental and safety concerns is pivotal. It not only predicts potential hazards but also recommends safer alternatives, aiding researchers in making informed decisions.
The following points highlight the impact of AI on laboratory safety:
- AI optimizes mining processes for sustainability and efficiency.
- Focus on safety, risk management, predictive maintenance, and real-time monitoring.
- Revolutionizing project management and reducing operational costs.
The Future of Chemical Research with Generative AI
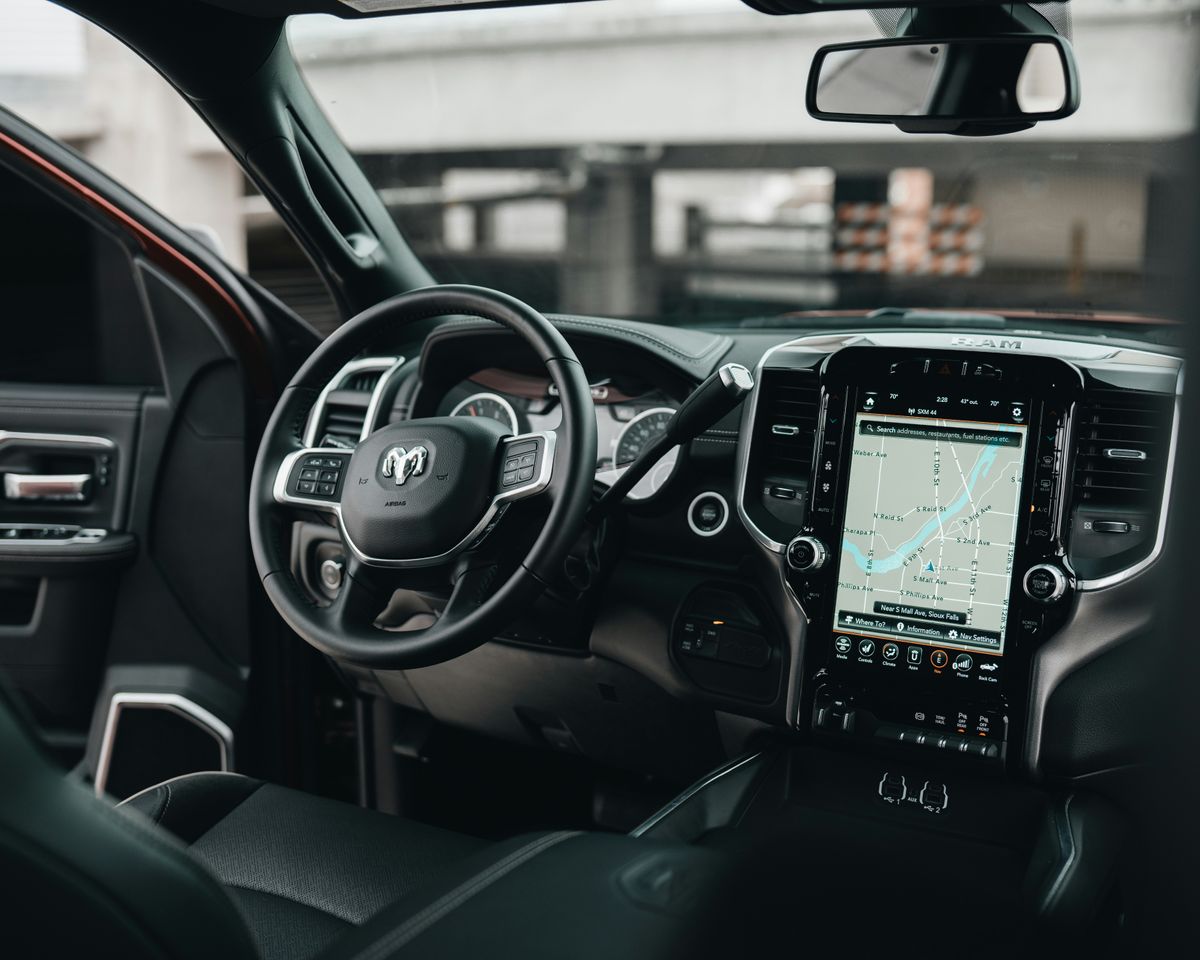
Generative Models for Novel Chemical Synthesis
The advent of generative AI has opened new avenues in the field of chemical synthesis. De novo molecular design is a process where AI suggests novel chemical structures that closely match a desired molecular profile. This involves creating a virtual chemical library for computational testing, which is then followed by synthesis and characterization.
Significant advances in deep generative modeling, such as variational autoencoders and GANs (Generative Adversarial Networks), have propelled this field forward. The 'Molecular Autoencoder', pioneered by Gómez-Bombarelli et al., showcased the potential of these technologies in detailed understanding and practical application.
The integration of AI in chemical synthesis not only accelerates the discovery process but also enhances the precision of the resulting compounds.
The table below summarizes key AI technologies and their contributions to chemical synthesis:
AI Technology | Contribution |
---|---|
Variational Autoencoders | Generating diverse chemical structures |
GANs | Refining the generation process |
Neural Networks | Predicting retrosynthetic pathways |
By leveraging these AI tools, chemists can navigate the vast chemical space more efficiently, leading to the discovery of new compounds with potential therapeutic and industrial applications.
AI in the Design of Next-Generation Catalysts
The integration of AI in catalyst design is transforming the field of materials science. AI-driven approaches are enhancing the precision and speed in developing catalysts that are crucial for various industrial processes. By utilizing machine learning algorithms, researchers can predict and optimize the performance of catalysts, leading to breakthroughs in efficiency and sustainability.
AI integration in design not only accelerates the discovery of new catalysts but also enables the creation of materials with bespoke properties. This is particularly evident in the development of Metal-Organic Frameworks (MOFs), where AI's predictive capabilities are used to tailor materials for specific applications like carbon capture or energy conversion.
The potential of AI in catalyst design is vast, with the ability to revolutionize how we approach molecular design and synthesis.
The table below illustrates the impact of AI on the design of next-generation catalysts:
Aspect | Impact of AI Integration |
---|---|
Precision in Design | Enhanced |
Speed of Discovery | Increased |
Tailoring of Properties | Customizable |
Sustainability of Processes | Improved |
Thought leaders in the field emphasize the importance of ethical AI development, transparency, and trust to ensure that the innovations in catalyst design are not only effective but also responsible and inclusive.
Impact of Generative AI on Chemical Industry Dynamics
The advent of Generative AI (GenAI) marks a significant milestone in the evolution of the chemical industry. Generative AI is emerging as the catalyst that could usher in a new era of innovation, offering powerful tools to tackle the industry's challenges, from the need for novel materials to eco-friendly manufacturing processes.
GenAI's influence extends across various domains, from R&D to commercialization, reshaping the landscape of the chemical sector. Its application in chemical process engineering, for instance, promises to revolutionize the way we approach problem-solving and innovation.
The potential of GenAI to mitigate existing bottlenecks in the industry is profound. It is not just about enhancing current processes but also about creating new opportunities for growth and sustainability. The table below outlines the key areas where GenAI is set to make an impact:
GenAI Applications | Expected Impact |
---|---|
R&D Innovation | Accelerated discovery of new materials |
Manufacturing Efficiency | Optimized processes and reduced waste |
Commercialization | Streamlined market entry for novel products |
As we look to the future, the chemical industry stands on the brink of a transformative shift, driven by the capabilities of GenAI. The insights from recent research and webinars underscore the importance of embracing this technology to stay competitive and meet the ever-evolving demands of the market.
Embracing the Future of Chemistry with AI
As we conclude our exploration of AI applications in the chemical industry, it is evident that artificial intelligence is not just an auxiliary tool but a revolutionary force reshaping the landscape of chemical research and development. From predictive modeling and smart lab instruments to addressing environmental concerns and streamlining manufacturing processes, AI's integration into chemistry heralds a new epoch of innovation and efficiency. The potential for AI to accelerate discovery, enhance safety, and foster sustainability in the chemicals and materials industry is immense. As researchers and industry professionals continue to harness the power of AI, we stand on the cusp of a smarter, more sustainable future in chemistry, where the synergy between human intellect and machine intelligence catalyzes progress beyond our current imagination.
Frequently Asked Questions
How is AI revolutionizing drug discovery in chemistry?
AI is revolutionizing drug discovery by accelerating the identification of new compounds, enhancing predictive models for drug efficacy, and streamlining clinical trials. By analyzing vast datasets, AI can predict the success of chemical transformations, suggest novel synthetic pathways, and facilitate high-throughput screening.
What role does AI play in the development of smart and sustainable materials?
AI algorithms are being used to advance the development of smart materials by customizing their properties and optimizing their design for specific applications. Additionally, AI contributes to the creation of sustainable materials by predicting environmental impacts and recommending safer alternatives.
How does AI improve efficiency in chemical manufacturing processes?
In chemical manufacturing, AI enhances process efficiency through automation, predictive maintenance, and quality control. AI systems can anticipate equipment failures, optimize production parameters, and ensure consistent product quality, leading to reduced downtime and increased output.
In what ways does AI contribute to environmental and laboratory safety?
AI contributes to safety by predicting chemical hazards, suggesting safer chemical processes, and monitoring laboratory conditions. It helps in making informed decisions that reduce environmental impact and enhance safety protocols in research settings.
What is Generative AI, and how is it impacting the chemical industry?
Generative AI (GenAI) refers to AI models that can generate novel chemical structures and synthesis pathways. It is impacting the chemical industry by enabling the design of next-generation catalysts, novel chemical synthesis, and transforming the dynamics of chemical research and commercialization.
Can AI replace human researchers in chemical laboratories?
While AI significantly enhances efficiency and discovery in chemical laboratories by automating tasks and analyzing data, it is not a replacement for human researchers. Instead, AI serves as a valuable tool that complements human expertise and creativity.