Renewable Energy 2.0: Navigating AI-driven Innovations
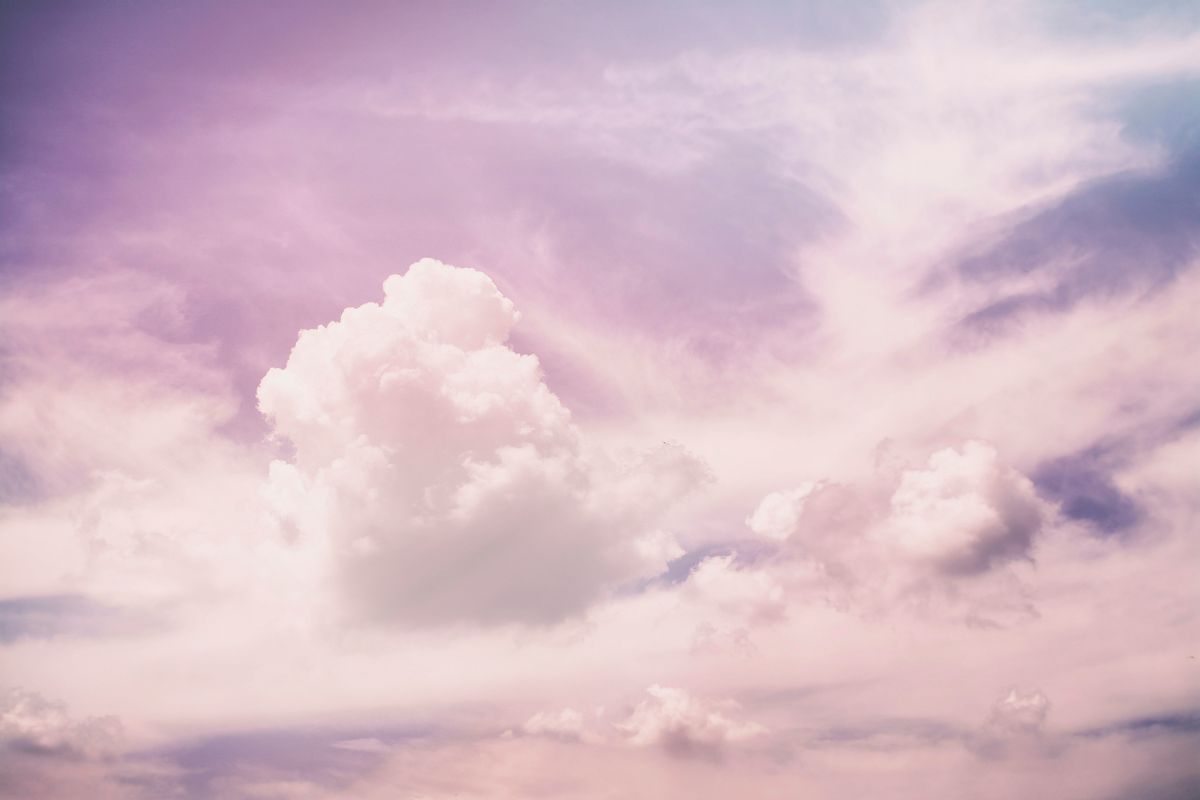
As the world pivots towards sustainable energy solutions, Artificial Intelligence (AI) stands at the forefront of this transformative journey. In the article 'Renewable Energy 2.0: Navigating AI-driven Innovations,' we delve into the myriad ways in which AI is reshaping the landscape of renewable energy. From optimizing production and enhancing grid management to revolutionizing energy consumption, AI's integration into the sector promises a future of efficiency, sustainability, and resilience. This article explores how AI-driven innovations are not only improving the output of renewable energy sources but also ensuring their seamless integration into our daily lives.
Key Takeaways
- AI optimization models significantly enhance the placement and maintenance of renewable energy installations, leading to increased efficiency and reduced downtime.
- Smart grids, powered by AI, are revolutionizing energy distribution, offering resilience and adaptability while engaging consumers with smart monitoring systems.
- Predictive analytics in AI are pivotal for balancing the supply and demand of energy, optimizing storage, and facilitating smart home energy systems integration.
- AI-enabled platforms and intelligent monitoring are key to reducing carbon footprints and preventing energy losses, advancing sustainability in energy consumption.
- The future of the energy sector is being redefined by AI innovations, with Machine Learning and Neural Networks tackling complex energy challenges and driving growth.
AI-Driven Optimization of Renewable Energy Production
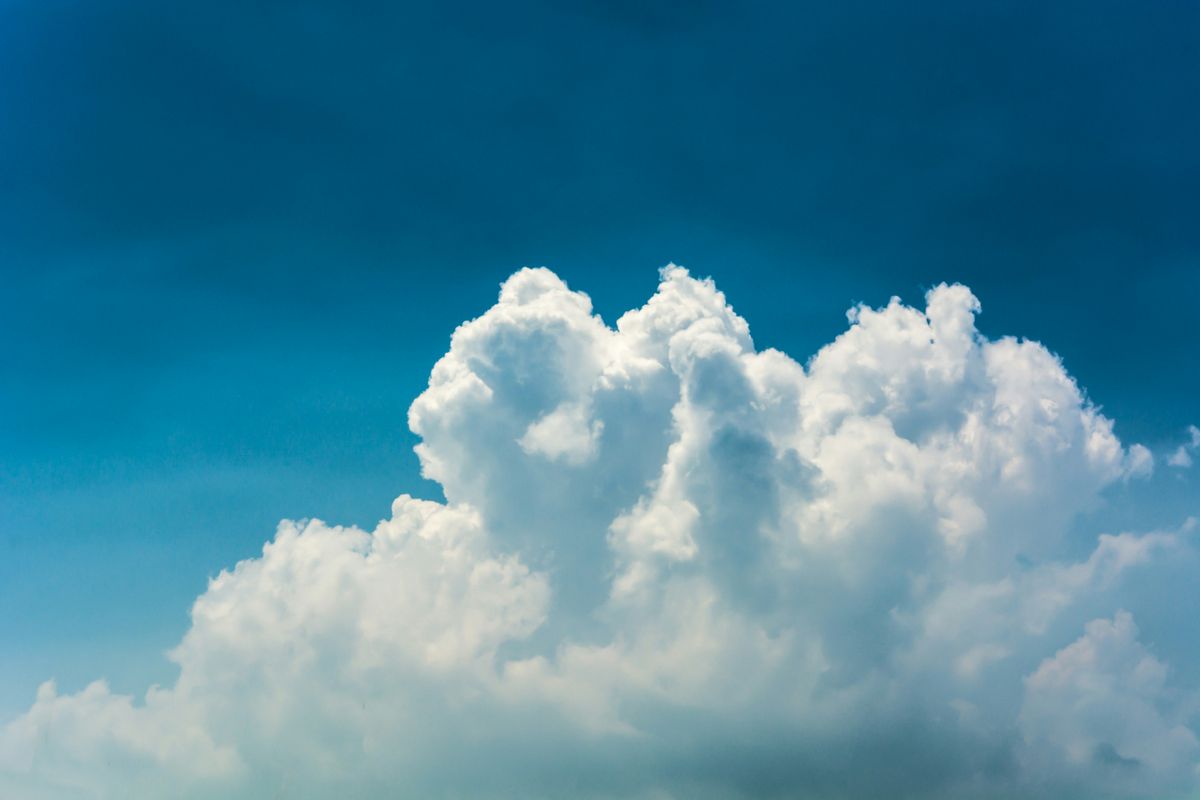
Predictive Analytics for Energy Forecasting
The advent of predictive analytics in the renewable energy sector marks a significant leap forward in forecasting accuracy. Neural Networks can use historical energy consumption data to forecast future energy demands with a startling degree of accuracy. This precision enables utility companies to maintain an optimal balance, ensuring that supply consistently meets demand while maximizing efficiency.
AI forecasting, a vital component of smart grids, employs machine learning models to predict energy demand. These models consider various factors such as time of day, seasonal weather patterns, economic conditions, and grid developments. As a result, energy suppliers can intelligently match dynamic supply with demand, optimizing the purchase of bulk power, charging storage facilities at ideal levels, and implementing AI-driven load shedding protocols.
Predictive maintenance is rapidly gaining traction as AI tools sift through vast data sets to identify early-stage trends that could signal future malfunctions. This proactive approach allows for preventive maintenance, potentially averting costly equipment failures and enhancing grid reliability.
The backbone of decentralized energy resources hinges on data analytics. Strategic data management is crucial for microgrid operations and the integration of DERs, ensuring efficient energy delivery even during extreme weather or high demand. This approach not only strengthens grid resilience but also facilitates a diverse energy portfolio, combining renewable and traditional power sources for consistent stability.
Intelligent Placement of Wind and Solar Installations
The strategic placement of wind and solar installations is pivotal for maximizing energy output. AI optimization models digest reams of geographic and meteorological data to identify the most productive sites. Factors such as latitude, cloud coverage, and solar irradiance are crucial in selecting locations with the highest energy generation potential.
Once operational, AI continues to play a vital role. Intelligent sensor networks and computer vision oversee the ongoing monitoring of facilities. Machine learning algorithms are adept at detecting malfunctioning panels or components that may require attention, ensuring continuous efficiency.
Wind power, now supplying over 6% of global electricity, benefits immensely from AI. Layout optimization algorithms consider topography and wind patterns for turbine placement. Moreover, turbines equipped with AI can self-adjust to changing conditions, dynamically optimizing their performance.
The integration of renewable energy sources into the power grid is complex, given their variability. AI's advanced forecasting algorithms are indispensable for predicting weather patterns and thus, optimizing energy production. This capability is essential for both solar and wind energies, which are inherently influenced by weather.
Enhancing Facility Performance with Predictive Maintenance
The integration of AI into renewable energy systems has ushered in a new era of operational efficiency, particularly through predictive maintenance. AI tools are now indispensable for early detection of potential equipment failures, ensuring that maintenance can be scheduled proactively, rather than reactively. This approach not only prevents costly downtime but also extends the lifespan of valuable assets.
Predictive maintenance leverages AI to analyze vast amounts of data, identifying subtle trends that could indicate impending malfunctions. Such foresight allows for timely interventions, minimizing the risk of unexpected breakdowns and optimizing the performance of energy facilities.
Efficient energy usage is another benefit of AI-driven maintenance. By optimizing resource allocation, businesses can achieve significant energy savings, which translates to lower operational costs and a reduced carbon footprint. The following points highlight the advantages of predictive maintenance in renewable energy systems:
- Reduction in unplanned downtime and emergency repairs
- Enhanced reliability and efficiency of energy production assets
- Cost savings through optimized maintenance scheduling and resource utilization
Asset optimization is a key focus, balancing the need for maximum efficiency with the reliability of the energy systems. AI and machine learning (ML) are at the forefront of this effort, providing layers of monitoring and advanced protection that evolve from simple threshold alerts to complex predictive models.
Smart Grids and AI: Revolutionizing Energy Distribution
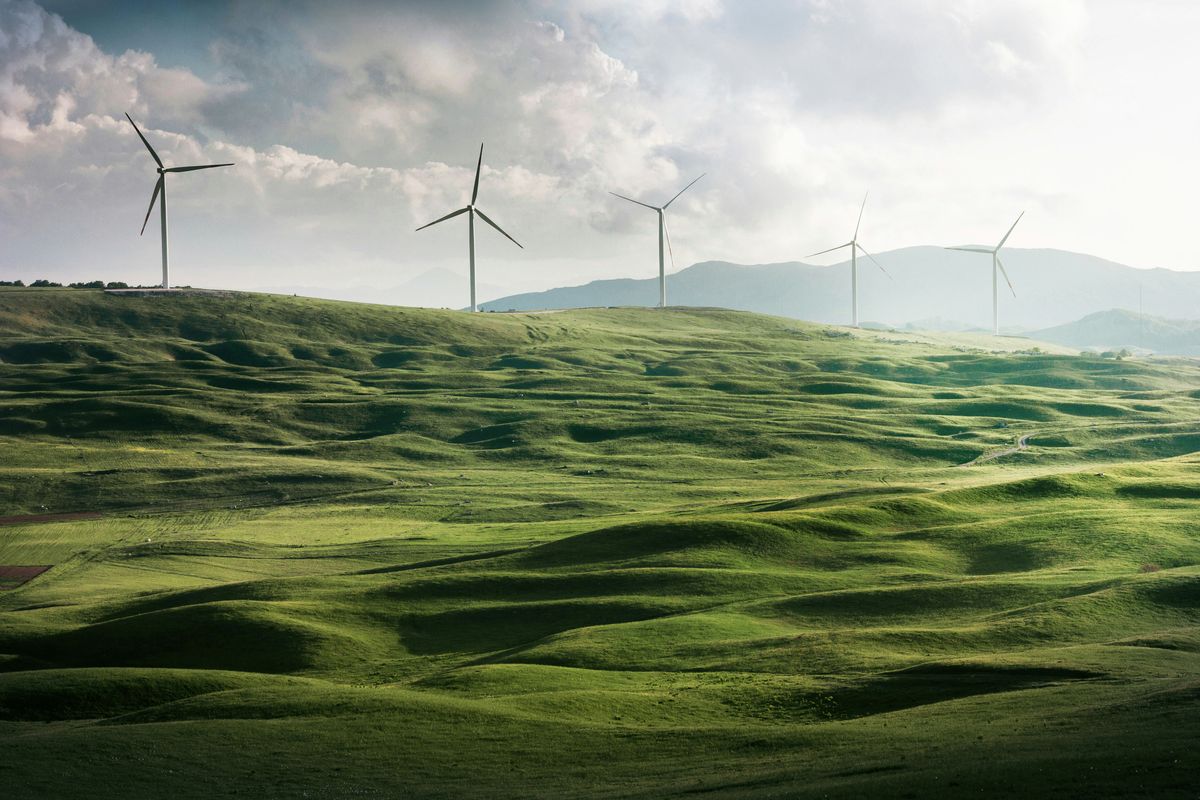
Adaptive Network Management for Efficiency
The advent of AI in energy management has led to a paradigm shift in how we approach grid efficiency. AI revolutionizes energy efficiency and the transition to renewables by enabling adaptive network management. This approach allows for the dynamic adjustment of energy distribution based on real-time demand and supply conditions.
Selective power reduction to lower priority areas is a critical strategy when supply is constrained, ensuring that essential services maintain uninterrupted power. AI's predictive analytics play a pivotal role in preventing grid imbalances and blackouts, which are common issues in outdated networks.
Asset optimization is another cornerstone of AI-driven network management. By maximizing asset efficiency and reliability, AI helps balance performance with maintenance needs, avoiding unnecessary downtime and extending the lifespan of energy infrastructure.
Commercial entities, such as office buildings and data centers, are also reaping the benefits of AI efficiencies. AI-driven building management systems utilize sensor networks to monitor and adjust energy usage in real-time, ensuring optimal consumption and reducing waste.
AI-Powered Solutions for Grid Resilience
The integration of AI into the energy sector has brought about a paradigm shift in how we manage and distribute electricity. AI's role extends beyond production; it is redefining energy consumption and grid management. Through sophisticated demand forecasting models, AI enables power generators and utilities to anticipate consumption patterns, leading to smarter energy distribution and reduced waste.
Optimized energy flow is crucial for enhancing grid stability and resilience. AI's prowess in cybersecurity also fortifies the grid against potential threats, ensuring the uninterrupted delivery of power. This dual capability of AI ensures sustainable and resilient grids, capable of withstanding the challenges of modern energy demands.
On the technical front, renewable energy sources face issues of intermittent, storage inefficiency, and grid integration challenges. AI solutions help optimize output, addressing these technical hurdles and paving the way for more reliable renewable energy systems.
The advent of decentralized energy resources (DERs) and smart grids has introduced a complex layer to energy management, necessitating innovative approaches to ensure their seamless integration and operation. Here, data analytics, powered by AI, becomes indispensable:
- Analyzing vast amounts of data from various sources, including IoT devices.
- Providing invaluable insights into grid operations.
- Enabling real-time decision-making.
- Enhancing the grid's responsiveness to changing energy demands.
Smart Monitoring Systems for End-User Engagement
Smart monitoring systems are transforming how end-users interact with their energy consumption. Advanced Metering Infrastructure (AMI) is at the forefront of this change, providing detailed insights into usage patterns. AMI systems enable two-way communication between consumers and utility providers, allowing for real-time data exchange and more informed decision-making.
Smart home ecosystems are another key player, leveraging AI to analyze appliance-level energy data. This facilitates not just monitoring but also intelligent automation, optimizing device operation to match utility rates and reduce costs.
The benefits of smart monitoring are clear:
- Enhanced visibility into energy consumption
- Identification of inefficiencies and waste
- Customized recommendations for energy savings
With the integration of AI, smart monitoring systems are not just reactive but proactive, identifying potential savings and automating adjustments to promote efficiency and sustainability.
The surge in AI adoption among energy and utility companies underscores the potential of these technologies to revolutionize energy management and engagement for consumers.
AI: The Game Changer in Renewable Energy Integration
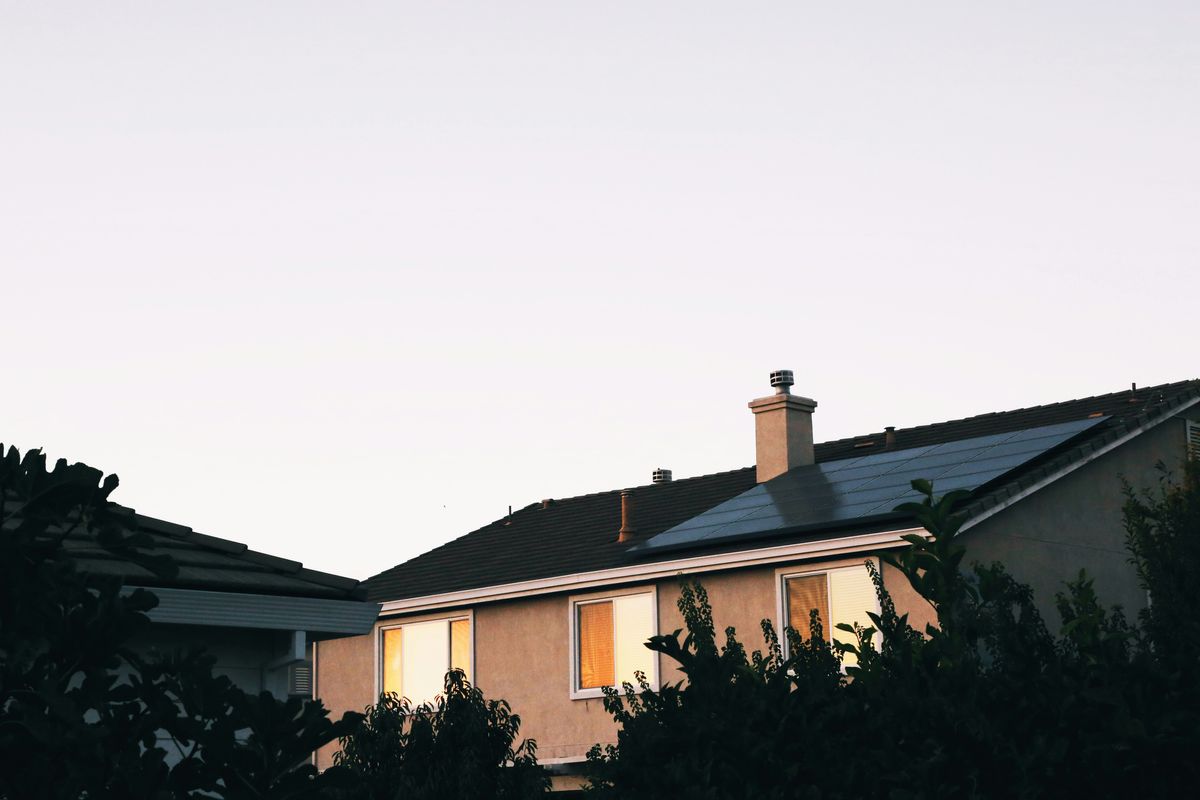
Balancing Supply and Demand with Predictive Analytics
The advent of AI in the energy sector has been nothing short of revolutionary. Predictive analytics, powered by machine learning and neural networks, is at the forefront of this transformation. By analyzing historical energy consumption data, AI systems can forecast future demands with remarkable precision. This not only ensures a consistent supply but also enhances the overall efficiency of the energy grid.
Predictive analytics plays a pivotal role in the intelligent balancing of supply and demand. It enables energy suppliers to purchase power in precise quantities, optimizing the use of storage facilities and implementing smart load shedding protocols. The result is a more cost-effective and sustainable energy system that is less reliant on fossil fuels and more on renewable sources.
The integration of AI into smart grids facilitates a dynamic and responsive energy network. It supports the shift towards decarbonization by enabling data-driven decision-making and promoting the use of 'smart' materials that self-diagnose and repair, thereby extending their lifespan.
The table below illustrates the impact of AI on energy demand forecasting and supply optimization:
Factor | Impact of AI |
---|---|
Energy Demand Forecasting | Improved accuracy and efficiency |
Supply Optimization | Reduced wastage and cost savings |
Renewable Energy Utilization | Increased practicality and affordability |
Grid Stability | Enhanced through intelligent protocols |
Optimizing Energy Storage and Grid Management
The integration of AI into energy storage and grid management is revolutionizing the sector, ensuring that the flow of electricity is optimized for both stability and resilience. AI's role in load forecasting is pivotal, as it allows grid operators to predict storage levels and balance supply with demand, especially when considering the variable nature of renewable energy sources like solar and wind.
Energy storage technologies have seen rapid advancements, with AI optimizing the charge and discharge cycles of large battery installations. This not only prolongs battery life but also reduces replacement costs, making renewable energy more economically viable. AI's proficiency extends to cybersecurity, fortifying the grid against potential threats and maintaining an uninterrupted power supply.
- Improved battery technologies
- Enhanced load balancing capabilities
- Initiatives for grid resilience
AI solutions are essential for addressing the intermittent nature of renewable energy and the challenges of grid integration. By smoothing out supply volatility and selectively reducing power in lower priority areas during constraints, AI helps prevent grid imbalances and energy waste.
Facilitating Smart Home Energy Systems
The advent of AI in the energy sector is not only revolutionizing sustainability practices but also enhancing the way we manage energy at home. Smart home energy systems, empowered by AI, are transforming residential energy consumption by providing actionable insights and automating control over household appliances.
Smart home ecosystems are increasingly becoming sophisticated, with AI-driven solutions offering a multitude of benefits:
- Granular data collection at the appliance level, enabling detailed monitoring and analysis.
- Customized insights into energy usage patterns, identifying inefficiencies and potential savings.
- Intelligent automation for device scheduling, optimizing energy use based on utility rates and user preferences.
AI is demystifying energy consumption, making waste visible and driving tangible efficiency improvements.
By integrating AI into smart home systems, consumers can enjoy a more interactive and responsive energy management experience. This not only contributes to individual cost savings but also plays a crucial role in combating climate change and maintaining electric grid stability. AI is essential for achieving greater efficiency, reducing carbon emissions, and addressing the broader environmental challenges we face today.
Improving Efficiency and Sustainability in Energy Consumption
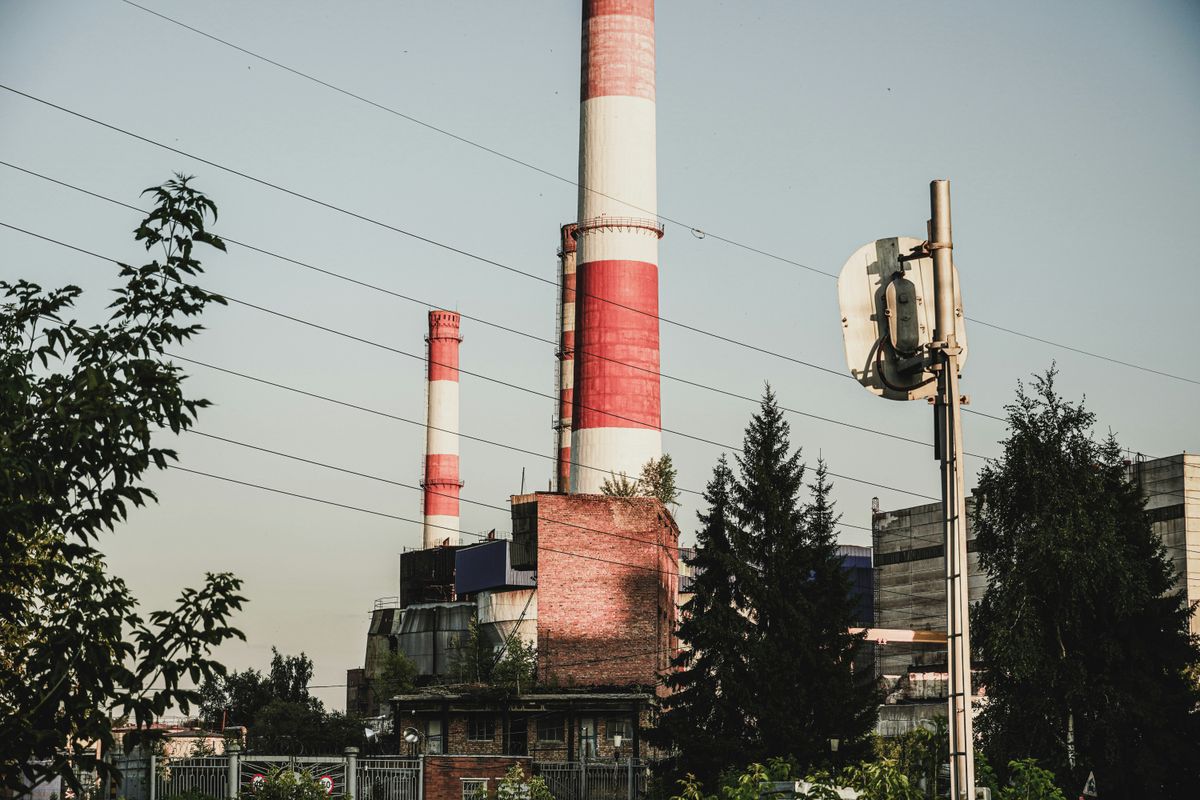
AI-Enabled Platforms for Energy Optimization
The advent of AI-enabled platforms has marked a significant leap forward in the realm of energy optimization. These platforms harness the power of artificial intelligence to provide actionable insights into energy consumption patterns, leading to more efficient use of resources. Smart home ecosystems and commercial facilities alike benefit from AI-driven analytics, which not only track and analyze energy usage but also suggest ways to reduce waste and improve overall efficiency.
- Smart thermostats and interactive energy management systems empower consumers to actively manage their energy use.
- Cloud-based analytics platforms process appliance-level data, offering tailored recommendations for energy savings.
- AI-powered automation intelligently schedules device operation, optimizing for cost and energy conservation.
AI-driven innovations are transforming the energy sector, enabling smarter consumption and promoting sustainability. By minimizing carbon footprints and preventing energy losses, these technologies are pivotal in the transition towards a more eco-friendly and efficient energy landscape.
The integration of AI into energy management software solutions, such as Datakwip, is a testament to the potential of these technologies. Datakwip optimizes energy consumption in commercial facilities by tracking energy patterns and providing AI-driven insights, which is a clear example of how AI is reshaping energy efficiency strategies.
Reducing Carbon Footprints through Intelligent Monitoring
The advent of AI-driven innovations has ushered in a new era of energy efficiency and waste management. These smart systems are pivotal in promoting sustainability, economic benefits, and environmental stewardship. By optimizing operations and fostering a circular economy, AI is instrumental in reducing carbon footprints.
AI applications are not only transforming the way we manage energy but also how we monitor and reduce emissions. From space-based monitoring of power plant emissions to real-time analytics of utility consumption, AI is at the forefront of identifying and mitigating wasteful practices.
For instance, software solutions like Proficy for Sustainability Insights utilize analytics to continuously detect excess usage and other forms of waste in utilities such as electricity and natural gas. This capability is essential for setting benchmarks and driving down emissions. Moreover, companies like Cirrus Nexus are leveraging the power of AI to strategically allocate computing loads to regions with abundant renewable energy, thereby minimizing carbon emissions and optimizing data center operations.
Intelligent monitoring is not just about tracking; it's about actionable insights that lead to tangible reductions in resource usage and emissions. The table below illustrates the impact of such AI-driven strategies:
Strategy | Resource Monitored | Reduction Achieved |
---|---|---|
Proficy for Sustainability Insights | Utilities (Electricity, Natural Gas, etc.) | Continuous waste detection |
Cirrus Nexus | Computing Emissions | Up to 34% for certain workloads |
Preventing Energy Losses with Smart Technologies
The integration of AI into energy systems is transforming the way we manage and conserve power. AI-driven technologies are pivotal in reducing energy waste across various sectors. By leveraging smart thermostats and interactive energy management systems, consumers can significantly decrease their energy consumption. These systems provide tailored insights on usage patterns, fostering a cost-effective and environmentally friendly approach to energy management.
- Smart Grid Systems to Reduce Energy Use
- Clean, Renewable Energy Sources
- Energy Conservation with Smart Storage Solutions
AI is a key to eliminating energy inefficiency at a systemic level, ensuring that every watt of clean power is optimally distributed and consumed.
Residential consumers, in particular, benefit from AI-powered energy monitoring solutions. Smart home ecosystems analyze appliance-level energy data, offering customized insights into consumption patterns and identifying areas of waste. With AI-powered automation, devices can be scheduled intelligently, aligning with utility rates and reducing unnecessary energy use.
Driving Innovation in the Energy Sector with AI
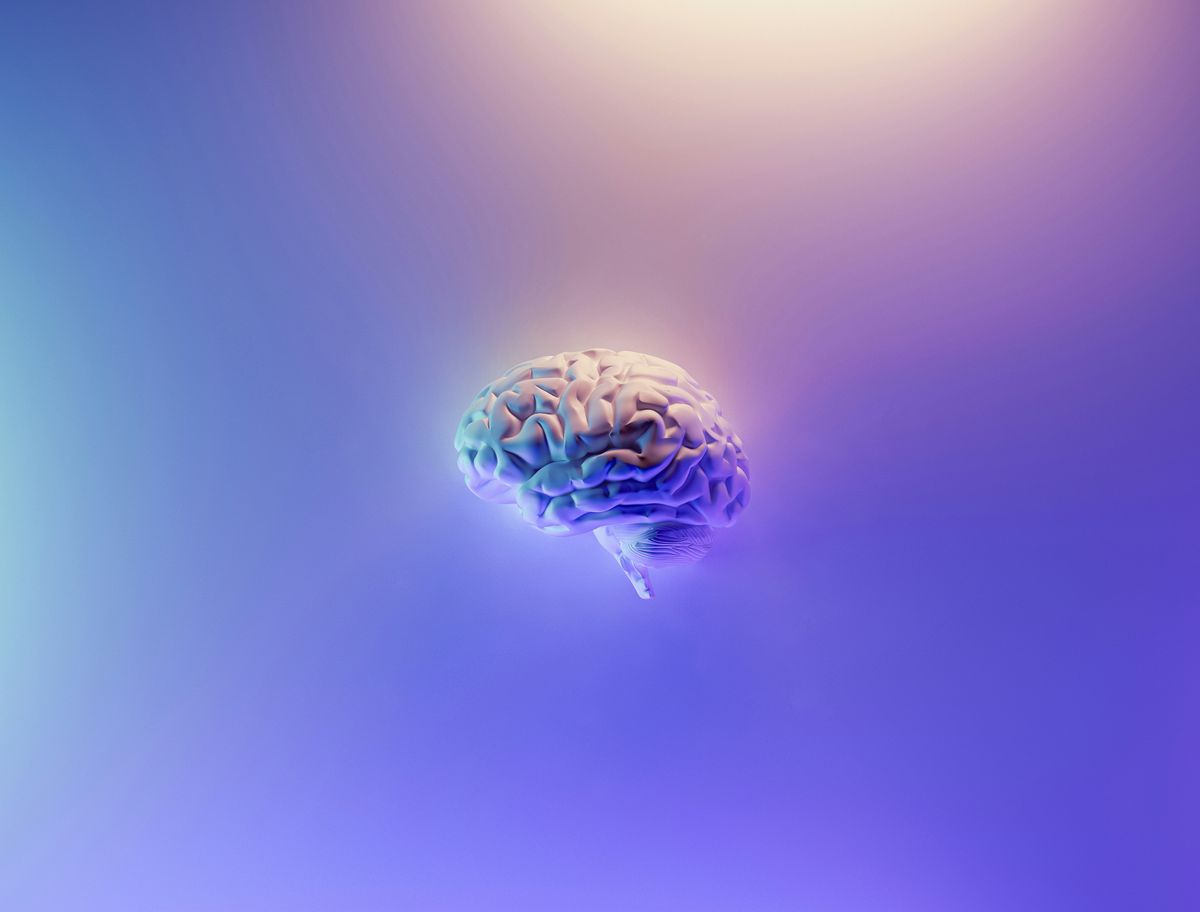
Machine Learning and Neural Networks in Energy Applications
The advent of Machine Learning (ML) and Neural Networks has ushered in a new era of smart automation within the renewable energy sector. These technologies are pivotal in optimizing the fabrication of solar cell parameters, as they can process vast amounts of data to identify patterns and predict outcomes with remarkable precision. ML techniques, such as artificial neural networks (ANN) and genetic algorithms (GA), are now considered some of the most realistic tools for enhancing energy production efficiency.
Neural Networks, in particular, have demonstrated their ability to forecast future energy demands by analyzing historical energy consumption data. This predictive capability is crucial for utility companies aiming to maintain an optimal balance between efficiency and supply reliability. By predicting demand, energy providers can minimize waste and ensure a consistent energy supply, which is essential for both economic and environmental sustainability.
In the materials domain, ML is contributing to the development of 'smart' materials. These advanced materials are designed to self-diagnose and repair, thereby extending their lifespan and reducing maintenance costs. The integration of ML and Neural Networks in energy applications not only promises a more efficient and sustainable future but also signifies a transformative step towards intelligent energy management and material utilization.
AI Techniques for Tackling Energy Challenges
The integration of Artificial Intelligence (AI) in the energy sector is pivotal for addressing the myriad of challenges it faces. AI techniques are transforming the landscape of energy management, from optimizing the flow of renewable energy to enhancing the efficiency of consumption patterns.
AI's capability to model and predict energy needs leads to more strategic deployment of resources. For instance, Machine Learning algorithms can analyze vast datasets to forecast energy demand, while Neural Networks can identify patterns that help in the intelligent design of materials and systems. These techniques not only improve the operational efficiency of energy systems but also contribute to the broader goal of sustainability.
The adoption of AI in energy and materials is a leap towards a future where every watt of clean power is utilized to its fullest potential, minimizing waste and maximizing impact.
Despite the promise, the sector faces challenges such as data security and the complexity of integrating AI into existing infrastructures. However, solutions are emerging to overcome these hurdles, ensuring that AI's potential can be fully harnessed. The table below outlines some of the key challenges and corresponding AI-driven solutions:
Challenge | AI-Driven Solution |
---|---|
Forecasting Energy Demand | Predictive Analytics |
Optimizing Resource Allocation | Machine Learning Models |
Enhancing Material Efficiency | Neural Network Design |
Ensuring Data Security | Advanced Encryption Techniques |
AI integration in the energy sector enhances grid stability, predicts demand, optimizes storage, and promotes sustainability. Asia-Pacific leads in AI adoption for energy efficiency and innovation, crucial for combating climate change.
The Future of Energy Intelligence and AI Potential
The advent of AI in the energy sector marks a pivotal shift towards what can be termed as energy intelligence. AI revolutionizes the energy sector by enhancing efficiency, optimizing renewable sources, enabling predictive maintenance, and improving grid management for a sustainable future. This transformation is not just a fleeting trend but a robust pathway to a more resilient and sustainable energy landscape.
Let's consider some notable benefits of AI-driven applications:
- Improving outage prediction and response times
- Accurate forecasting of energy production from renewable sources
- Enhancing grid management and energy storage
- Offering smart home platforms that optimize energy consumption
AI's contributions to the sector are innovative and dynamic, ushering us into an era of "energy intelligence". As we look ahead, the potential for AI to drive further innovation in energy and materials is immense. AI techniques like Machine Learning and Neural Networks are set to redefine our approach to energy use, leading to groundbreaking advancements in resource computation, energy optimization, and material science.
The integration of AI into energy operations unlocks a higher level of service delivery, innovation, and efficiency. It's a transformative power that stakeholders are beginning to harness, reshaping the energy industry into one that is more adaptive, intelligent, and forward-thinking.
Harnessing AI for a Sustainable Energy Future
In summary, the integration of AI into renewable energy systems marks a transformative leap towards a sustainable future. The insights and efficiencies gained from AI-driven innovations are not only optimizing energy production and grid management but are also empowering consumers to make smarter energy choices. As we navigate the complexities of renewable energy integration, AI stands as a beacon of progress, guiding us toward a more efficient, reliable, and clean energy landscape. The potential of AI to revolutionize the energy sector is immense, and its continued evolution promises to accelerate our transition to a world powered by renewable energy. Embracing these AI-driven advancements is crucial for achieving our environmental goals and securing a resilient energy infrastructure for generations to come.
Frequently Asked Questions
How is AI optimizing renewable energy production?
AI optimization models are being used to intelligently place wind turbines and solar arrays, perform predictive maintenance to minimize downtime, and utilize forecasting solutions to anticipate energy supply levels. This helps integrate renewable sources into the grid more seamlessly.
What role does AI play in smart grid technology?
AI is integral to the development of smart grids, offering adaptive network management for increased efficiency, resilience, and end-user engagement. AI-powered systems can predict outages, optimize energy distribution, and manage energy storage, revolutionizing how power grids operate.
In what ways does AI contribute to energy efficiency?
AI contributes to energy efficiency by enabling smart grid technologies that reduce waste and optimize energy usage. It also provides businesses and consumers with intelligent monitoring systems to track and minimize their carbon footprints, and prevent energy losses.
How does AI facilitate the integration of renewable energy into power grids?
AI facilitates the integration of renewable energy into power grids by using predictive analytics to balance supply and demand, optimizing energy storage and grid management, and supporting smart home energy systems that adjust consumption based on availability and need.
What innovative AI techniques are being applied in the energy sector?
Innovative AI techniques in the energy sector include machine learning and neural networks for analyzing vast datasets, optimizing facility design and performance, and developing energy applications that tackle various challenges in production, distribution, and consumption.
What is the potential future impact of AI on the energy sector?
AI's future impact on the energy sector includes leading the charge towards more sustainable, efficient, and resilient energy systems. AI is expected to continue driving innovation, boosting efficiency, and helping to tackle the challenges of decarbonization and renewable energy integration.