Precision Medicine: AI's Influence on Drug Discovery
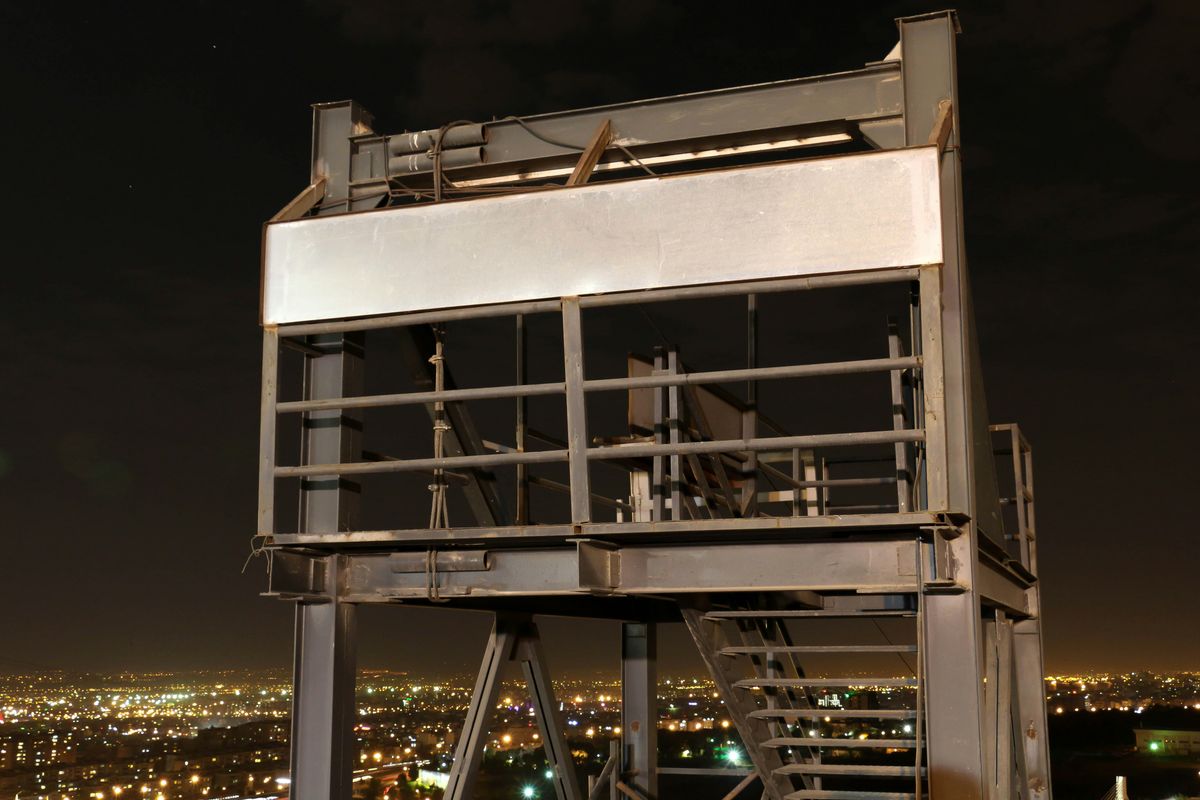
The integration of Artificial Intelligence (AI) into the realm of precision medicine marks a transformative era in healthcare, particularly in the domain of drug discovery. AI's sophisticated algorithms and machine learning techniques are revolutionizing the way we approach the development of new drugs, tailoring treatments to individual genetic profiles, and streamlining clinical trials. This article delves into the multifaceted impact of AI on precision medicine and the innovative strides being made towards more personalized and effective healthcare solutions.
Key Takeaways
- AI is playing a crucial role in personalizing treatment strategies, utilizing predictive analytics to enhance patient outcomes, and addressing the inefficiencies of traditional trial-and-error approaches.
- The drug discovery process is being accelerated by AI through the identification of drug targets, optimization of drug design, and the development of AI-designed drug candidates like INSO18_055.
- Genomics and big data are being leveraged by AI to predict disease risk and tailor treatments, marking a significant convergence of technologies that are reshaping healthcare.
- AI is transforming clinical trials by improving the selection process of participants, reducing costs, increasing efficiency, and introducing concepts like digital twins and virtual trials.
- Digital health tools empowered by AI are facilitating patient self-management of chronic conditions, providing real-time health monitoring, and paving the way for the future of patient-centered care.
The Role of AI in Personalized Treatment Strategies
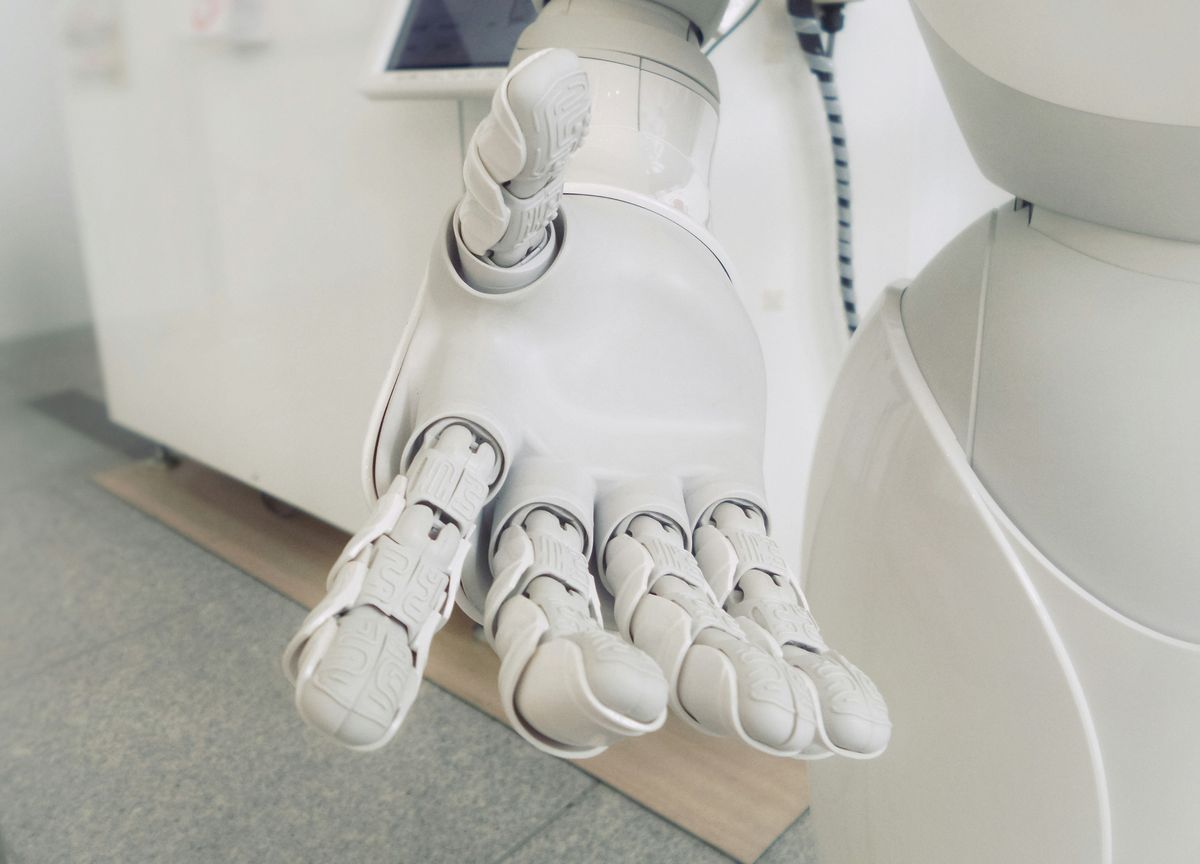
Understanding Precision Medicine
Precision medicine represents a transformative approach to healthcare, where treatments and preventive strategies are tailored to individual patient characteristics. This paradigm shift leverages cutting-edge technologies, including genomics, biomarkers, and digital health tools, to deliver care that is more effective and efficient.
AI in healthcare advances personalized medicine through tailored treatment plans, precise diagnostics, and accelerated drug discovery, improving patient outcomes and healthcare quality. By integrating AI-driven analytics, precision medicine can predict which treatment and prevention strategies will work in which groups of people. It's more than a one-size-fits-all approach; it's about the right treatment for the right person at the right time.
Precision medicine is not just a theoretical concept; it is already bringing about significant changes in the medical field. It is estimated that by 2030, precision medicine could save up to $460 billion in healthcare costs while enhancing the quality of life for millions.
The impact of precision medicine is evident across various aspects of healthcare:
- Predictive analytics for early disease detection
- Personalized treatment plans based on genetic profiles
- Revolutionizing clinical trials with more targeted participant selection
- Reducing the trial and error in traditional treatment approaches
By addressing the unique challenges posed by rare diseases and complex conditions, precision medicine, underpinned by AI, is setting a new standard in healthcare innovation.
AI-Driven Predictive Analytics
The advent of AI-powered predictive analytics is a game-changer in the realm of precision medicine. By analyzing vast datasets, AI algorithms can forecast potential health outcomes with remarkable accuracy. This foresight is pivotal for personalized medicine, as it enables healthcare providers to tailor treatment plans to the individual needs of patients, ensuring more efficient patient care and earlier detection of diseases.
- AI-driven analytics can identify patterns in genetic data, medical images, and bloodwork that may elude human analysis.
- These insights facilitate the development of tailored therapies, improving overall health management.
The integration of AI into predictive analytics marks a significant stride towards a more proactive and personalized healthcare system.
Enhancing Patient Outcomes with Personalized Care
The advent of precision medicine has been a game-changer in healthcare, enabling treatments that are tailored to the individual needs of patients. AI-driven predictive analytics are at the forefront of this revolution, providing clinicians with powerful tools to anticipate health outcomes and tailor interventions accordingly. This proactive approach can lead to earlier detection of diseases and more effective health management strategies.
Personalized medicine is not just a concept but a practice that is increasingly becoming the standard of care. By integrating genetic testing into the treatment planning process, physicians can identify an individual's disease risk and potential drug responses. This level of customization ensures that patients receive the most appropriate medications and therapies, minimizing side effects and maximizing efficacy.
The integration of AI into precision medicine is not only revolutionizing clinical trials but also empowering patients to take an active role in their healthcare journey. With the support of digital health tools, individuals can monitor their health in real-time, making informed decisions and managing their conditions more effectively.
The impact of AI on patient outcomes is profound and multifaceted. From accelerating drug discovery to providing personalized healthcare companions, AI is helping to streamline processes and enhance the quality of care. As we look to the future, the potential for AI to further improve patient outcomes is immense, with predictions suggesting significant healthcare cost savings and healthier lives for millions.
Revolutionizing Drug Discovery with AI
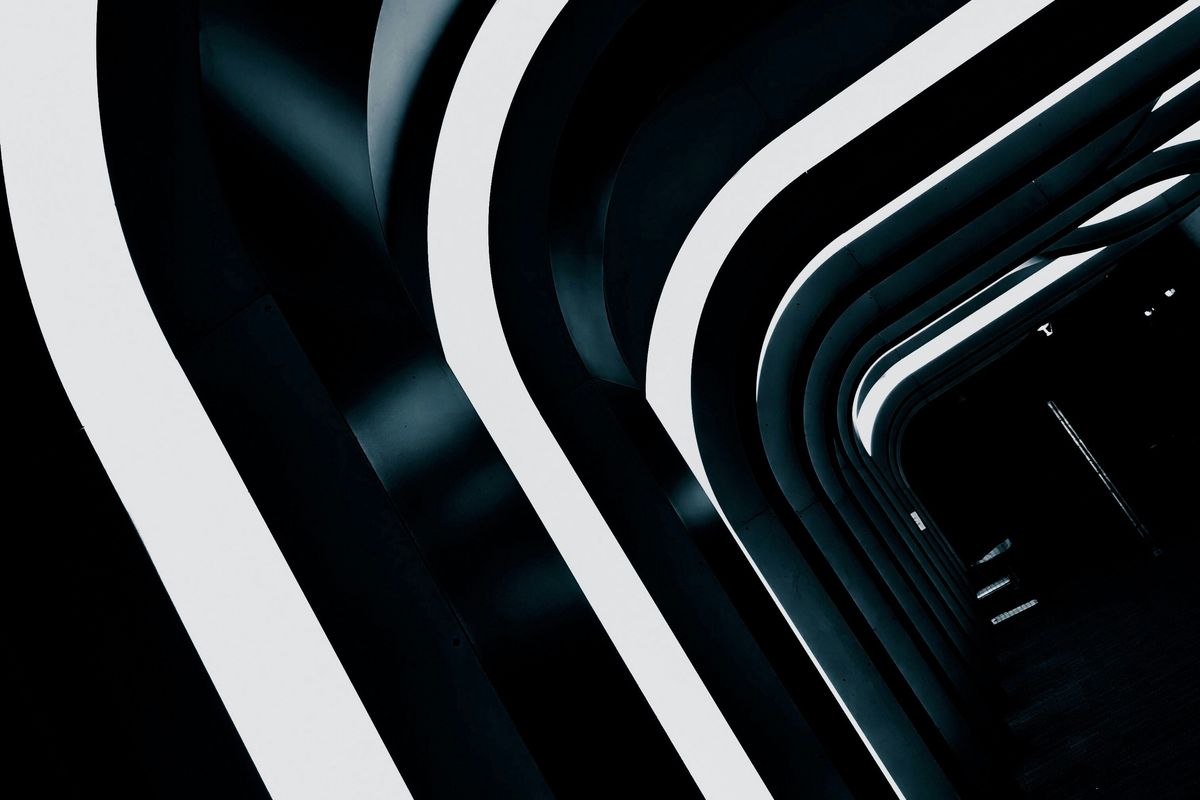
Accelerating the Identification of Drug Targets
The advent of AI in drug discovery has ushered in a new era where the traditional approach of 'Disease
ightarrow Target
ightarrow Drug' is being redefined. AI can sift through the mountains of biological literature and OMICS data to produce a plausible target hypothesis for a drug for a particular disease. This computational re-focusing is crucial, given that an estimated 10% of all conditions are misdiagnosed every year.
Historically, computational methods like the pharmacophore model have played a pivotal role in drug selection and optimization. Today, advances in AI and ML, including deep learning, are enabling the analysis of more complex and larger quantities of data. These methods, while primarily correlative, have shown promise in addressing the challenges of drug development.
AI's influence is not limited to target identification; it extends to the design of drug candidates, such as INSO18_055, which is now in the pipeline for clinical trials.
The integration of AI in drug discovery is not without its challenges, but it presents significant opportunities. AI/ML applications are beginning to yield results that could shorten the time to identify clinical candidates and streamline the entire drug development process.
Optimizing Drug Design and Development
The integration of AI into drug design and development marks a transformative shift from traditional methods. AI-driven models are now capable of analyzing vast datasets, leading to more informed decisions in the early stages of drug discovery. This not only accelerates the process but also enhances the precision of targeting specific diseases.
Computational approaches have evolved significantly since the inception of the pharmacophore model in the 1960s. Today, AI and machine learning (ML) techniques, including deep learning, are being applied to understand complex biological systems and predict how new drugs will interact with their targets. The promise of AI in this field is underscored by the development of drugs like INSO18_055, which was identified and designed using AI technologies and is now advancing through the pipeline.
The reimagining of the drug development paradigm from disease to target to drug reflects a strategic shift towards a more efficient and effective process. By leveraging AI, the industry aims to reduce the high failure rates and exorbitant costs associated with traditional drug development.
The table below highlights the stark contrast between traditional and AI-enhanced drug development:
Aspect | Traditional Approach | AI-Enhanced Approach |
---|---|---|
Time | 14 years on average | Reduced significantly |
Cost | ~$2 billion | Potentially lower |
Success Rate | ~10% | Expected to improve |
Embracing AI in drug development is not without challenges. While AI methods are adept at identifying correlations, establishing causality remains a hurdle. Nonetheless, the industry's pivot towards AI signifies a commitment to overcoming these obstacles and improving outcomes for both physicians and patients.
Case Study: INSO18_055 and AI's Impact
The development of INSO18_055 by Insilico Medicine marks a significant milestone in the realm of drug discovery, showcasing the practical applications of AI in this field. AI's role in identifying drug targets and designing drug candidates has transitioned from theoretical to tangible, with INSO18_055 now advancing through clinical trials. Although no drugs developed solely using AI have received FDA approval yet, the case of INSO18_055 illuminates the potential for AI to revolutionize the drug development process.
The promise of AI in drug development is not just in the creation of new drugs, but in the potential to reduce the high failure rates traditionally associated with this sector.
The journey of INSO18_055 from conception to clinical trial encapsulates the transformative power of AI in drug development:
- AI-driven predictive models identified novel drug targets.
- Advanced algorithms designed the drug candidate with precision.
- The speed of development was significantly accelerated.
- The approach aims to enhance the efficacy and safety profile of the drug.
The success of AI in this case study serves as a beacon for future endeavors, where AI integration into drug development could become the norm rather than the exception.
AI-Enhanced Genomics and Big Data in Medicine
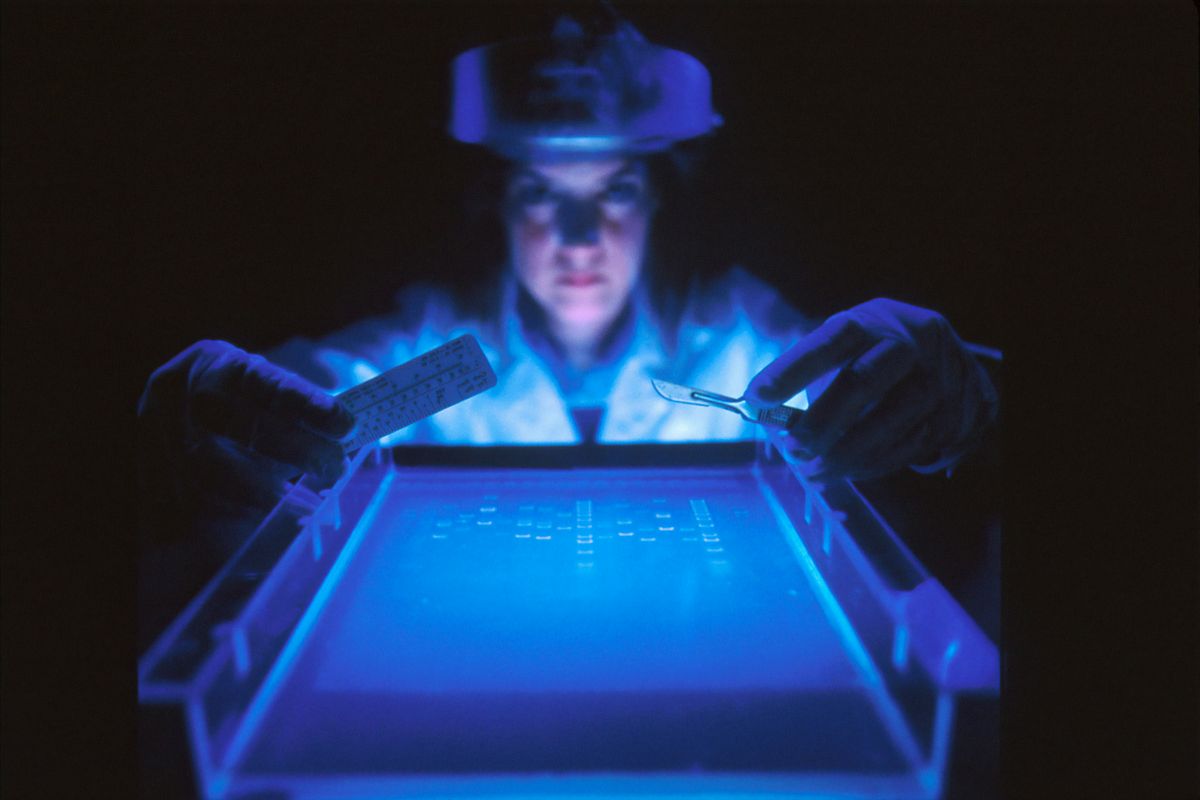
Decoding DNA for Disease Prediction
The advent of genomics has ushered in a new era in medicine, where decoding an individual's DNA can reveal not just their ancestry, but also their predisposition to certain diseases. This genetic foresight is pivotal in precision medicine, allowing for the development of proactive strategies to either prevent or mitigate the impact of potential health issues.
Genomics contains critical information related to potential function and dysfunction. For instance, mutations in the BRCA1 gene significantly raise the risk of breast cancer, although they do not guarantee the onset of the disease. By understanding these genetic markers, healthcare providers can tailor their approach to each patient, considering their unique genetic makeup.
Precision medicine, informed by genomics, is not a one-size-fits-all solution. It is a nuanced approach that takes into account individual differences in people's genes, environments, and lifestyles.
The integration of AI in genomics has been transformative, optimizing the analysis of vast datasets and enhancing the accuracy of disease prediction. AI's ability to process and interpret complex genetic information rapidly accelerates the journey from genetic insight to clinical application, ultimately leading to more personalized and effective healthcare solutions.
Big Data Analytics in Healthcare
The integration of big data analytics in healthcare is transforming the landscape of patient care and disease management. Employing predictive analytics, healthcare professionals can now develop machine learning models that forecast the likelihood of patients developing certain diseases. This proactive approach enables earlier interventions and more tailored treatment strategies.
Big data analytics also play a crucial role in enhancing the efficiency of healthcare systems. By analyzing vast datasets, AI algorithms can identify patterns and insights that would be impossible for humans to discern unaided. These insights can lead to improved patient outcomes and more efficient resource allocation.
The synergy between big data analytics and healthcare is paving the way for a future where medical decisions are more data-driven and personalized than ever before.
Here are some key benefits of big data analytics in healthcare:
- Early detection of diseases
- Customized patient care plans
- Reduction in healthcare costs
- Improved patient engagement and satisfaction
- Enhanced drug development processes
The Convergence of Genomics and AI Technologies
The integration of genomics and artificial intelligence (AI) is transforming the landscape of precision medicine. AI's analytical prowess is pivotal in interpreting the complex data derived from genomic sequencing. This synergy enables the identification of genetic markers associated with diseases and the prediction of individual responses to various treatments.
- DNA sequencing technology, coupled with AI, can reveal individual disease risks and potential drug responses.
- AI assists in analyzing the vast datasets generated by genomics, providing a comprehensive health perspective.
- The collaboration between AI and genomics paves the way for more accurate diagnoses and tailored treatment plans.
By reducing the trial and error in traditional treatment approaches, AI-enhanced genomics leads to more effective treatments for patients with complex or rare diseases.
While the potential is immense, the pharma industry faces challenges in regulation and standardization that could influence the deployment of AI in healthcare. Addressing these challenges is crucial for harnessing the full potential of AI in precision medicine.
Transforming Clinical Trials through AI
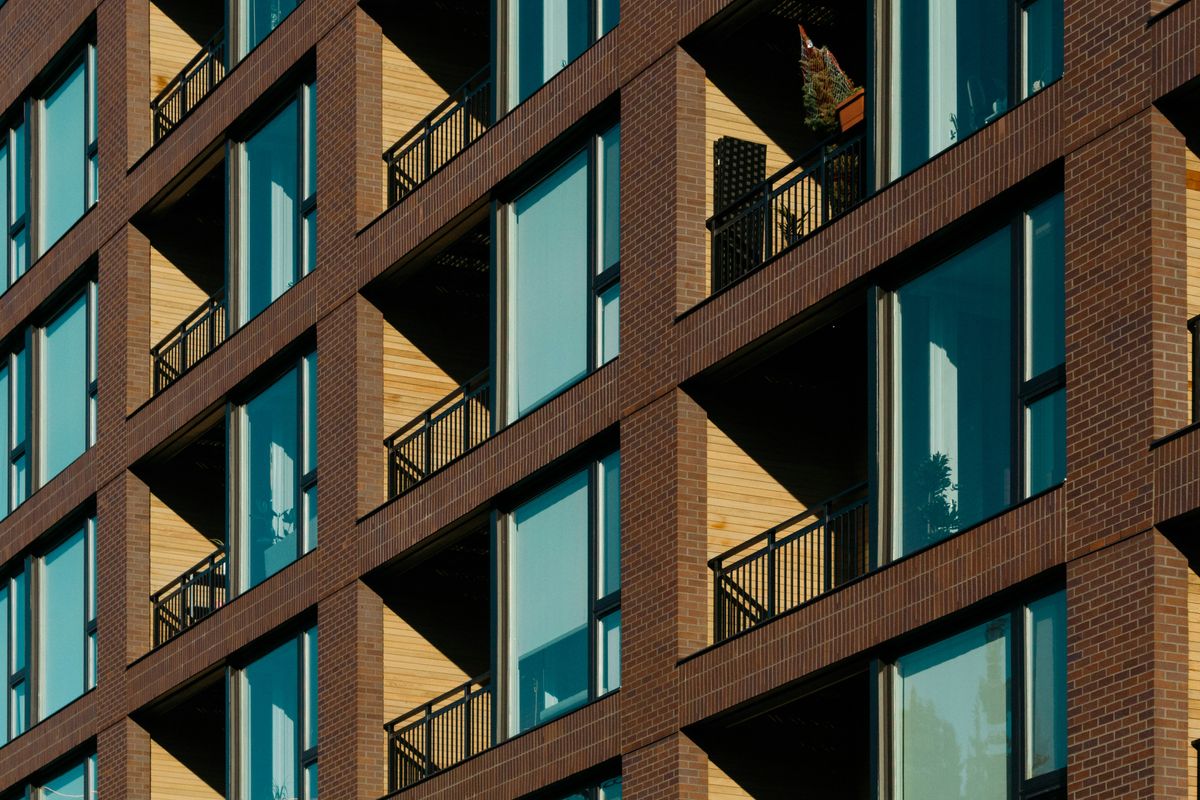
Streamlining Participant Selection
The integration of AI into clinical trials is a game-changer for participant selection. Technology has the ability to streamline formerly complicated admissions requirements, making them more accessible to qualified applicants. By leveraging predictive analytics and machine learning, AI can identify the most suitable candidates for a trial, ensuring a diverse and representative sample.
Stratification of disease processes allows for the creation of subgroups within patient populations, which can lead to more targeted and successful clinical trials. This approach not only refines the selection process but also uncovers unique drug development opportunities:
- Identification of patient subtypes
- Recognition of novel therapeutic targets
- Improved regulatory approval rates
Building trust with new models for clinical trial design is essential. Decentralized strategies and patient-centric designs increase participation and acceptance of novel therapies, especially in underserved communities.
The real-world diversity of patients is a critical factor in clinical trials. Current efforts to enhance diversity are expanding to include a broader range of demographics, such as age, gender, ethnicity, disability, and even previously excluded groups like children and pregnant women.
Reducing Costs and Improving Efficiency
The integration of AI into clinical trials is a game-changer for the pharmaceutical industry. By leveraging AI-driven algorithms, the process of developing new drugs becomes not only faster but also more cost-effective. AI technologies can reduce costs, accelerate drug development, streamline patient recruitment processes, and shorten study durations. This efficiency is crucial in an industry where time and financial resources are of the essence.
Precision medicine and AI go hand in hand in revolutionizing clinical trials. The use of AI allows for more targeted and efficient trials, which in turn leads to significant cost reductions. For instance, AI can help in:
- Identifying the most promising drug candidates early in the development process.
- Optimizing trial design to ensure the most effective use of resources.
- Enhancing patient recruitment by accurately matching participants to trials.
The ultimate goal is to enhance healthcare efficiency and promote affordability, making life-saving treatments more accessible to patients.
The impact of AI on the cost and efficiency of clinical trials is not just theoretical. Data shows a marked improvement in the number of successful trials and a reduction in the time it takes to bring new drugs to market. The following table illustrates the progress made between 2019 and 2023:
Year | Increase in Clinical Trials | Pipeline Value Growth |
---|---|---|
2019 | - | - |
2023 | 50% | Quadrupled |
These advancements underscore the transformative power of AI in the healthcare sector, particularly in the realm of clinical trials.
Digital Twins and Virtual Trials
In the realm of clinical trials, the concept of digital twins represents a groundbreaking shift. These virtual replicas of patients or tissues allow researchers to simulate and analyze the effects of drugs in a controlled digital environment. Digital twins serve as a bridge between theoretical models and real-world applications, enhancing the predictive power of clinical trials.
The benefits of digital twins in clinical trials include:
- Improved patient safety by anticipating adverse reactions
- Reduction in the need for physical trials, thereby lowering costs
- Enhanced ability to tailor treatments to individual patient profiles
By leveraging digital twins, we can expect a more nuanced understanding of drug interactions and patient responses, leading to more effective and safer treatments.
The integration of digital twins into clinical trials is not without challenges. It necessitates a reevaluation of traditional trial designs and a willingness to embrace new technologies that can address evolving questions in drug development. As we move towards more personalized medicine, the diversity of patient populations in trials—and their digital counterparts—must reflect real-world complexity.
Digital Health and AI-Powered Patient Empowerment
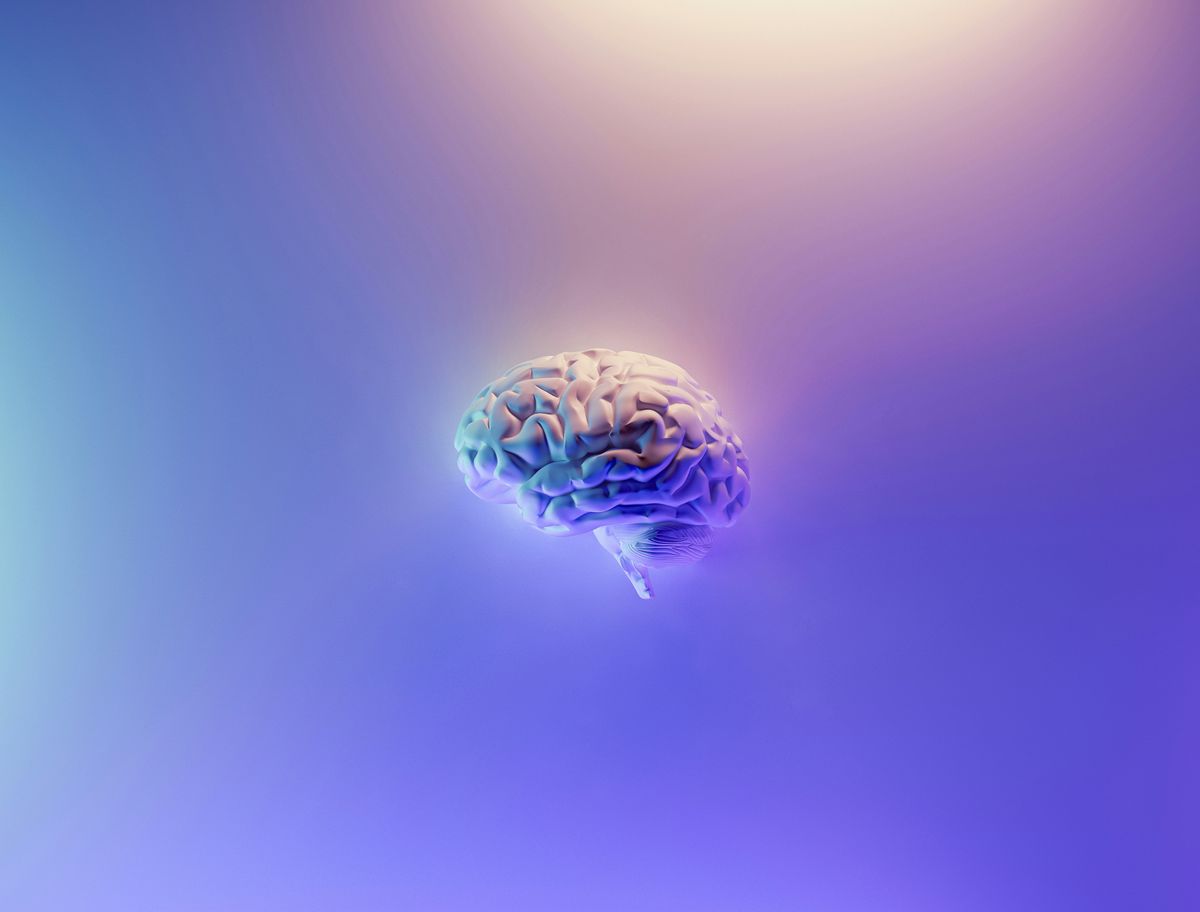
Self-Management Tools for Chronic Conditions
The advent of AI-powered self-management tools has marked a significant shift in how individuals with chronic conditions engage with their health. AI integration in healthcare improves personalized treatment, remote monitoring, medication management, and predictive maintenance for enhanced patient care and operational efficiency. These tools empower patients to take an active role in managing their conditions, often leading to better health outcomes and a reduced burden on healthcare systems.
Social determinants of health and clinical history play a crucial role in the management of chronic conditions. Patients often deal with multiple comorbid conditions and a complex regimen of medications and supplements. AI can help in stratifying these patients into subgroups for better disease management and targeted care.
It is critical to recognize that disease is a process, not a state. AI tools can assist in developing disease trajectories based on temporal clinical measurements, providing a more dynamic and personalized approach to disease management.
The use of AI in self-management is not without challenges. Data may be incomplete or contain errors, and there is a need for careful interpretation to account for the heterogeneity of real-world patients. However, the potential benefits are substantial, offering unique opportunities for improved patient outcomes.
Real-Time Health Monitoring
The advent of digital health tools has significantly advanced the capabilities of real-time health monitoring. These tools, often in the form of wearable devices, collect a myriad of data points that are crucial for tracking an individual's health status. The integration of artificial intelligence (AI) with these devices has opened up new possibilities for predictive analytics in healthcare.
Real-time monitoring systems can now analyze data from wearables to predict potential health issues before they become critical. For instance, AI algorithms can assess patterns in heart rate variability, sleep quality, and physical activity to provide early warnings of health deterioration. This proactive approach to health management empowers patients to seek timely medical intervention.
- Mental health tracking: AI helps in identifying patterns that may indicate stress or depression.
- Activity tracking: Monitors daily physical activity to encourage a healthy lifestyle.
- Metabolism monitoring: Provides insights into nutritional intake and energy expenditure.
- Sleep analysis: Evaluates sleep patterns to improve overall well-being.
By leveraging AI for real-time health monitoring, healthcare providers can offer more personalized and timely care, potentially reducing the need for emergency interventions and hospital readmissions.
The Future of Patient-Centered Care
The advent of AI in healthcare has paved the way for a future where patient-centric systems are at the forefront of health technology. Personalized treatment is no longer a distant dream but a rapidly approaching reality. As we move towards 2024, the Health Tech industry is shifting from a one-size-fits-all approach to one that embraces the uniqueness of each patient's needs.
Precision medicine, bolstered by AI, is not just a buzzword; it's a paradigm shift that promises to enhance the quality of life for patients. By integrating predictive analytics, genomics, and digital health tools, AI is enabling a more nuanced understanding of individual health profiles.
The potential of AI in precision medicine is immense. It's estimated that by 2030, AI could save up to $460 billion in healthcare costs while significantly improving patient outcomes.
The table below outlines the key components of AI-driven healthcare solutions that are transforming patient care:
No. | AI Healthcare Solution |
---|---|
1. | Predictive Analytics |
2. | Personalized Medicine |
3. | Revolutionizing Clinical Trials |
4. | Digital Health Tools |
5. | Accelerated Drug Discovery |
6. | Personal Healthcare Companions |
Building trust with patients through new models for clinical trial design and decentralized strategies is essential. This approach not only improves patient outcomes but also reduces costs and streamlines healthcare processes.
Conclusion
The integration of AI into precision medicine represents a paradigm shift in drug discovery and healthcare. By harnessing the power of predictive analytics, genomics, and big data, AI is enabling a more personalized approach to treatment, revolutionizing clinical trials, and accelerating the drug discovery process. The success stories emerging from AI applications, such as Insilico Medicine's INSO18_055 and Sanofi's AI-driven drug discovery, underscore the potential for AI to not only streamline the development pipeline but also to enhance the accuracy and efficacy of treatments. While challenges remain, particularly in moving from correlative to causal relationships, the opportunities for AI to improve patient outcomes and optimize healthcare are vast and continually expanding. As AI models evolve and become more sophisticated, we can anticipate a future where precision medicine, powered by AI, becomes the standard for patient care, leading to better health outcomes and a transformation in the way we approach disease and treatment.
Frequently Asked Questions
What is AI for Precision Medicine?
AI for precision medicine refers to the application of artificial intelligence technologies to tailor medical treatment to the individual characteristics of each patient. It involves analyzing large datasets, including genetic information, to predict disease risk, prognosis, and response to treatments, thereby enhancing personalized care and treatment strategies.
How is AI revolutionizing drug discovery?
AI is revolutionizing drug discovery by accelerating the identification of drug targets, optimizing drug design and development, and reducing the time and costs associated with these processes. AI algorithms can analyze vast quantities of data to identify potential candidates and improve the efficiency of clinical trials.
Can AI improve the success rate of clinical trials?
Yes, AI can improve the success rate of clinical trials by using algorithms to screen and select suitable candidates, making trials more efficient, and reducing costs. AI-driven predictive analytics can also help in creating digital twins and virtual trials, which can lead to more successful clinical outcomes.
What role does genomics play in AI-driven precision medicine?
Genomics plays a crucial role in AI-driven precision medicine by providing the genetic data that AI algorithms analyze to predict individual disease risks and drug response potential. This information helps doctors make more accurate diagnoses and personalized treatment plans.
How do digital health tools empower patients in precision medicine?
Digital health tools empower patients by allowing them to monitor and manage their own health in real-time. This leads to better self-management of chronic conditions, improved overall well-being, and a more patient-centered approach to healthcare.
What is the impact of AI on the efficiency of healthcare systems?
AI has a significant impact on the efficiency of healthcare systems by reducing trial and error in treatment approaches, enabling effective treatments for complex diseases, and streamlining healthcare operations. As a result, patients experience better outcomes, costs are lower, and healthcare delivery becomes more efficient.