Networking Evolution: AI in Networking Equipment
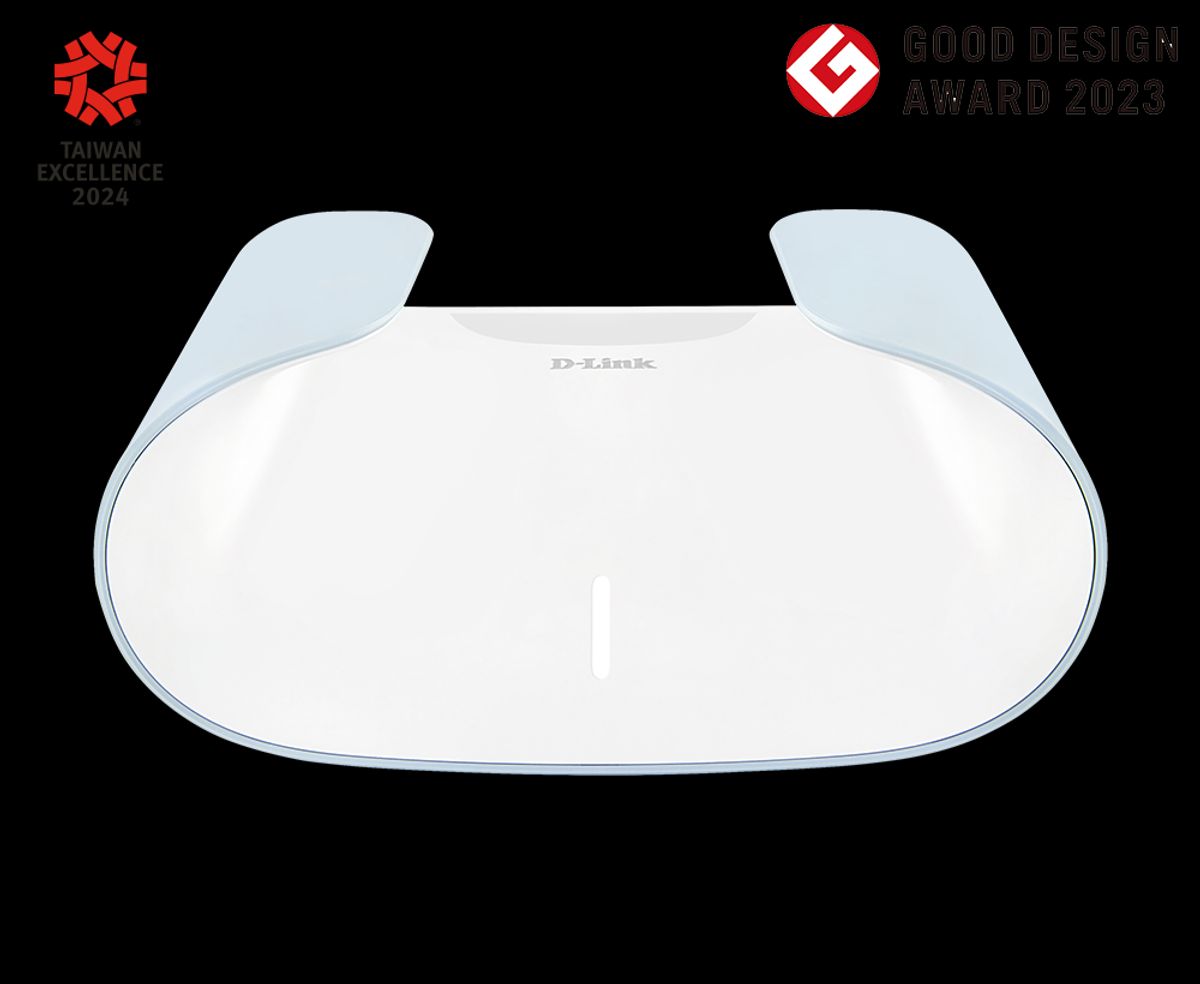
With the advent of Artificial Intelligence (AI), networking is undergoing a profound transformation, promising to revolutionize how data is transmitted, managed, and utilized across diverse environments. AI-powered networking, also known as AI networking, is poised to reshape the landscape of connectivity, offering unprecedented opportunities for optimization, automation, and innovation. As AI continues to evolve, its integration into networking equipment is becoming increasingly sophisticated, leading to smarter, more efficient, and more secure networks.
Key Takeaways
- AI Networking leverages advanced algorithms and machine learning to enhance network performance, reliability, and security, predicting behaviors and proactively addressing issues.
- Built-in AI acceleration, such as that spearheaded by Intel, is driving modern network innovation, optimizing resources for better efficiency and user experiences.
- AI-driven autonomous networking systems are set to revolutionize network management by enabling intelligent, self-optimizing networks without human intervention.
- AI Networking plays a critical role in strengthening cybersecurity by providing real-time threat identification, anomaly detection, and swift cyber attack responses.
- The integration of AI with edge computing optimizes data processing and minimizes latency, significantly enhancing the performance of edge computing applications.
The Essence of AI Networking
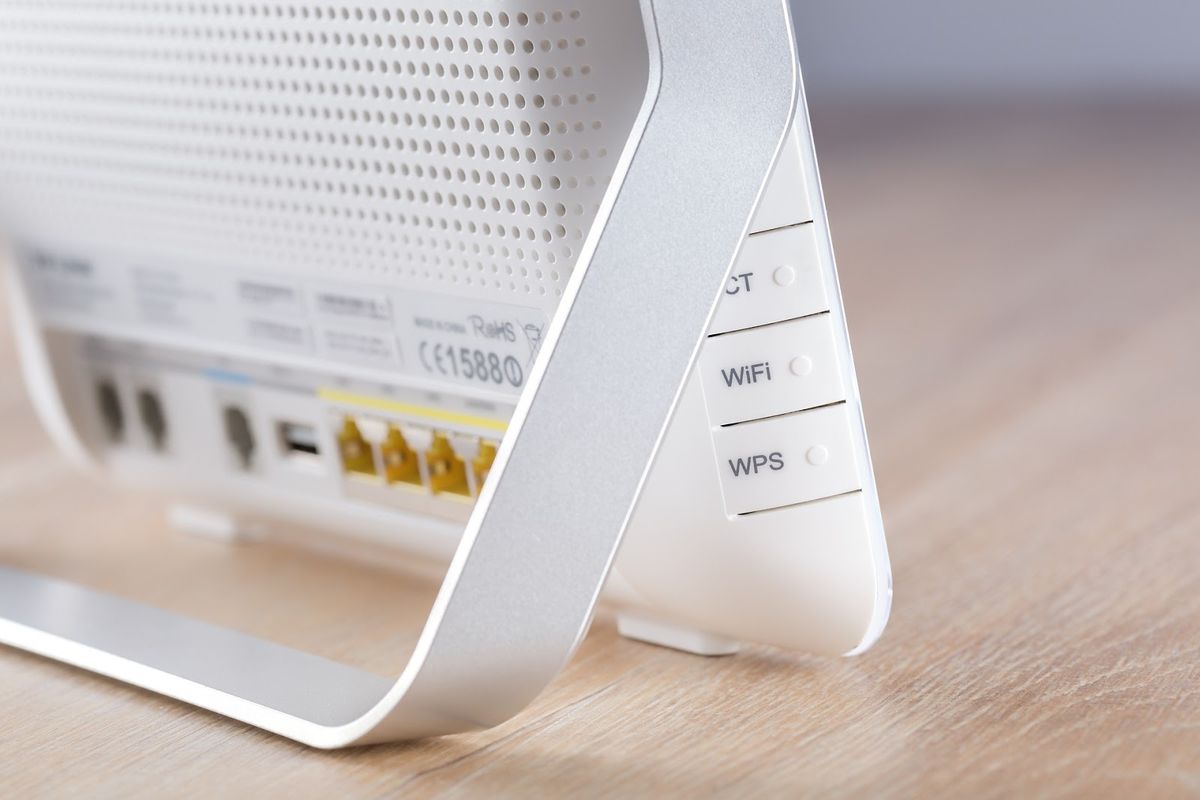
Advanced Algorithms and Machine Learning Techniques
The integration of artificial intelligence (AI) in networking equipment is revolutionizing the way networks are managed and operated. Advanced algorithms and machine learning techniques are at the forefront of this transformation, enabling networks to become more intelligent and responsive to the dynamic needs of users and applications.
- AI-driven analytics tools process vast amounts of network data to identify trends and optimize performance.
- Machine learning models are trained to predict network behavior, facilitating preemptive measures to ensure reliability.
- Continuous improvement is a hallmark of AI in networking, with systems evolving through iterative learning from data.
The synergy between AI and networking is not just about immediate gains in efficiency; it's about laying the groundwork for a future where networks self-manage and adapt in real-time to changing conditions.
With the rise of AI in various sectors, networking equipment must also evolve to support the increasing demands for AI-driven services. Companies like NVIDIA and Super Micro Computer are pivotal in providing the hardware capable of supporting edge AI solutions and on-premises deployment. The reach of AI extends to diverse environments, necessitating high-performance storage solutions and a network evolution tailored for efficient AI-driven services.
Real-Time Data Analysis and Pattern Recognition
The integration of AI into networking equipment has revolutionized the way networks are monitored and managed. AI transforms network optimization with capabilities such as real-time traffic analysis, predictive resource allocation, and automated issue resolution. By continuously analyzing network traffic, AI systems can identify patterns that may indicate performance issues or security threats.
Predictive maintenance and troubleshooting are critical applications of AI in IT services. These systems can forecast potential equipment failures by analyzing historical data alongside real-time network metrics, thus enabling proactive maintenance and reducing network downtime.
AI's ability to analyze vast amounts of data and recognize complex patterns is pivotal for maintaining network reliability and efficiency.
For example, in the manufacturing sector, AI-driven predictive quality assurance uses machine learning (ML) algorithms to detect trends that could lead to product defects. This proactive approach allows for timely corrective actions, ensuring high-quality outputs and customer satisfaction.
Proactive Issue Resolution and Network Adaptation
The integration of AI into networking equipment has ushered in a new era of proactive issue resolution. Networks can now anticipate and rectify problems before they escalate, ensuring uninterrupted service and optimal performance. This is achieved through AI's ability to analyze vast amounts of data and identify patterns that may indicate potential issues.
AI revolutionizes telecommunications by enhancing network efficiency, improving customer experience, and enabling predictive maintenance.
AI-driven systems adapt in real time, adjusting configurations to maintain network integrity. This dynamic adaptation is crucial for handling unexpected traffic surges or infrastructure failures. The following list highlights key benefits of AI in proactive network management:
- Automated network configuration simplifies integration and reduces deployment times.
- Enhanced scalability and flexibility support growing network demands.
- Access to advanced network security services fortifies against digital threats.
Challenges such as infrastructure limitations, data security concerns, and regulatory compliance must be navigated to fully harness AI's potential in networking.
Key Components and Applications of AI in Networking
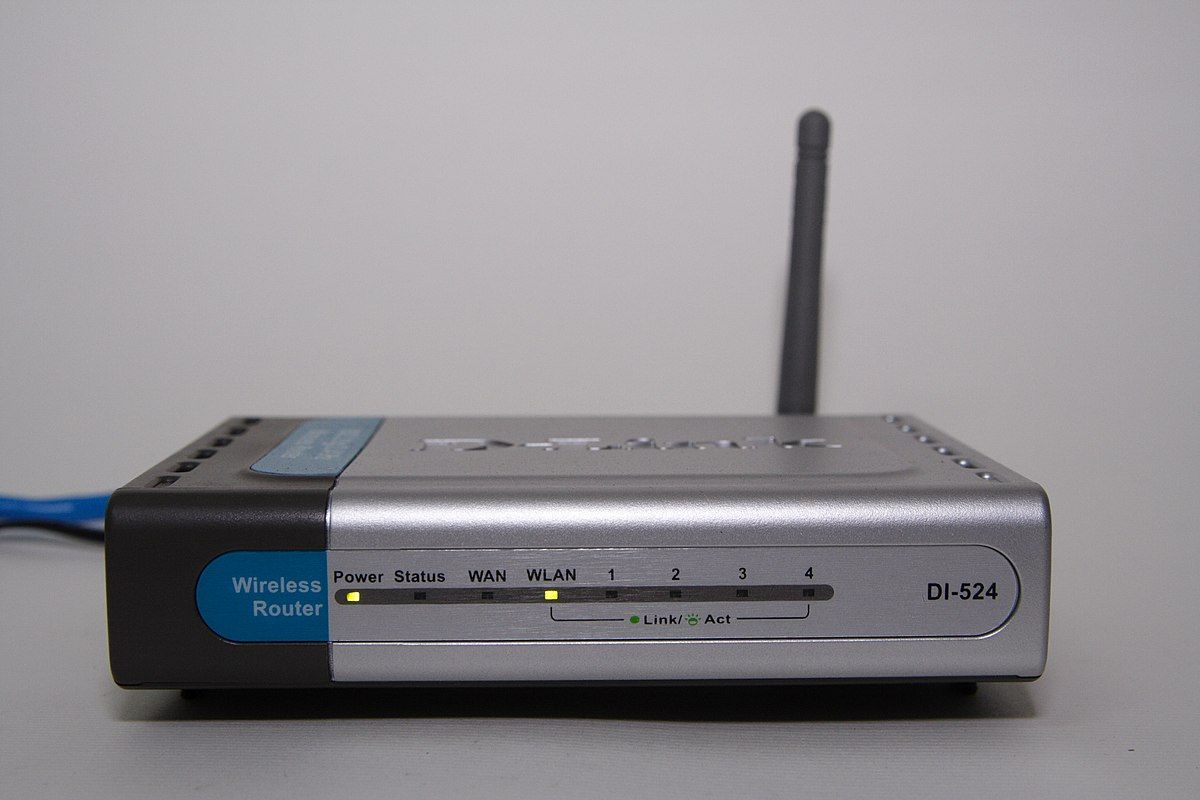
Dynamic Bandwidth Allocation and Traffic Prioritization
In the realm of AI networking, dynamic bandwidth allocation is a game-changer. By leveraging advanced AI algorithms, networks can now prioritize traffic in real-time, ensuring that critical applications receive the bandwidth they need to perform optimally. This is particularly beneficial in environments with fluctuating demands or complex traffic patterns.
AI-driven bandwidth allocation not only enhances network efficiency but also significantly improves user experiences. It allows for the seamless operation of high-priority tasks, even during peak usage times.
Predictive analytics are crucial for capacity planning, allowing networks to scale resources efficiently. This leads to optimized resource allocation and, as a result, substantial cost savings. The table below summarizes the impact of AI on network management:
Aspect | Impact of AI |
---|---|
Network Efficiency | Improved through dynamic resource optimization |
User Experience | Enhanced by prioritizing critical applications |
Operational Efficiency | Increased by automating routine tasks |
Cost Savings | Achieved through reduced need for manual intervention |
Autonomous networking systems further extend these capabilities, making intelligent decisions and self-optimizing without human intervention. Technologies such as MPLS enhance this process by directing data along the most efficient routes, akin to a GPS for network traffic.
Enhanced User Experiences through Network Efficiency
The integration of AI in networking has led to significant advancements in network optimization. By dynamically allocating bandwidth and prioritizing traffic, AI algorithms ensure that network resources are utilized in the most efficient way possible. This results in reduced latency and a seamless user experience, especially in environments with complex traffic patterns or variable demands.
Network efficiency is not just about speed; it's about the intelligent management of the network's capabilities. Features such as Simplified virtual network management and Infrastructure as a Service (IaaS) contribute to a more flexible and scalable network infrastructure. These improvements directly translate to an enhanced user experience, with users enjoying consistent connectivity and access to advanced network security services.
The relentless evolution of the digital landscape necessitates networks that can adapt quickly and efficiently. AI-driven networks are at the forefront of this transformation, providing the agility needed to support new business models and streamline existing operations.
The challenges faced by network operators, such as the overwhelming complexity of modern networks, are being addressed by AI's ability to automate and optimize. This not only elevates the end-user experience but also positively impacts business outcomes by reducing the time spent on manual tasks and creating operational efficiencies.
Adapting to Fluctuating Demands with AI
The integration of AI into networking equipment has revolutionized the way networks handle fluctuating demands. AI-driven systems are capable of predicting demand patterns and adjusting resources in real-time to ensure optimal performance. For instance, in the context of data centers, AI can predict and manage these fluctuating demands, allocating computing and storage resources more precisely and efficiently.
AI's predictive capabilities extend beyond simple forecasts, enabling networks to dynamically adapt to changing conditions without manual intervention.
The benefits of AI in adapting to demand fluctuations are numerous:
- Enhanced forecasting accuracy: By analyzing historical data and current trends, AI can provide more accurate predictions of network demand.
- Resource optimization: AI enables the efficient allocation of network resources, reducing waste and improving overall network efficiency.
- Cost savings: With better demand prediction and resource allocation, networks can operate more cost-effectively, passing savings onto consumers or reinvesting in further technological advancements.
Security Enhancement through AI Networking
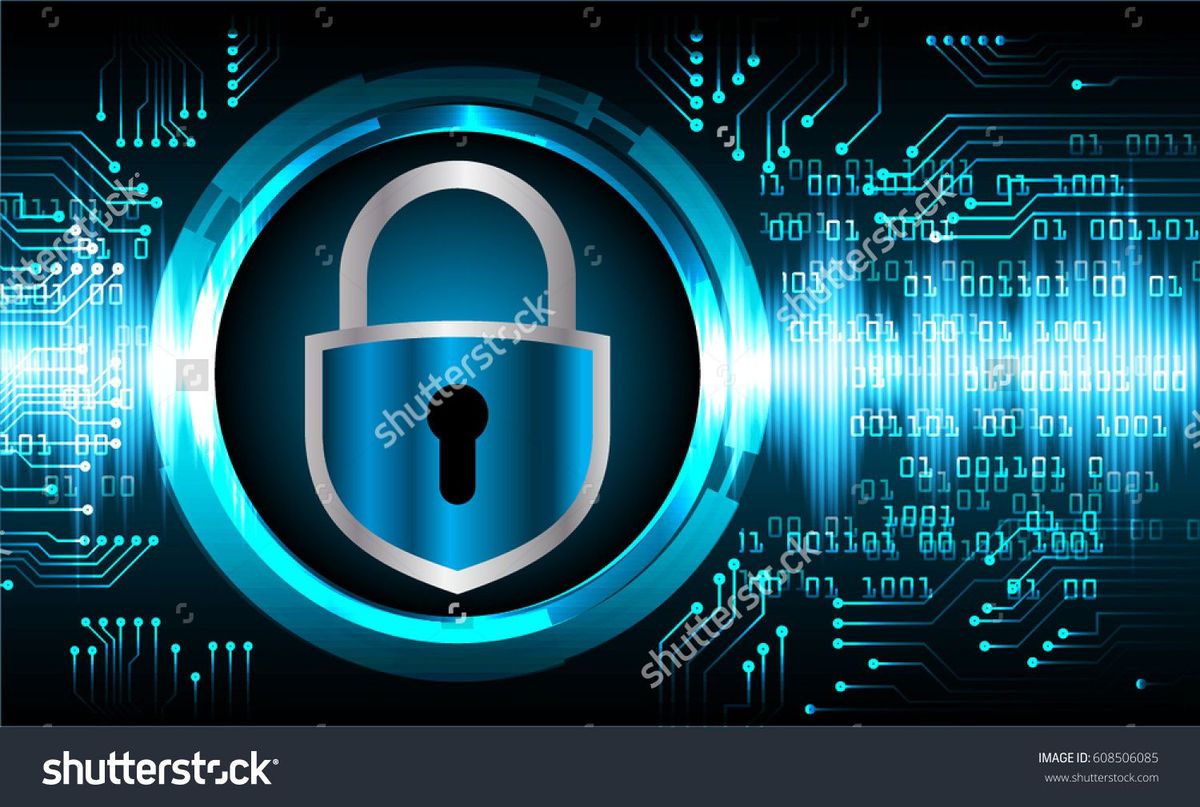
Real-Time Threat Identification and Mitigation
In the realm of AI networking, real-time threat identification and mitigation is a cornerstone for maintaining robust security postures. AI-driven systems continuously monitor network traffic, swiftly identifying and addressing potential threats. This proactive approach ensures that security incidents are managed before they can escalate, significantly reducing the risk of data breaches and other cyber attacks.
The integration of AI into network security has led to impressive outcomes, as evidenced by solutions like Sutherland Netshield AI for Operators. Users of such AI-powered tools have reported substantial improvements:
- 50% reduction in service-impacting equipment failures
- 60% decrease in time to resolve service-impacting issues
Real-time network monitoring with AI ensures quick responses to network issues and uninterrupted services. AI in telecommunications enables personalized marketing strategies for customer engagement and loyalty.
These advancements in AI networking not only enhance security but also contribute to a more efficient and reliable network infrastructure. As AI continues to evolve, its role in identifying and mitigating threats in real-time becomes increasingly integral to the modern digital landscape.
Anomaly Detection and Cyber Attack Response
In the realm of cybersecurity, AI-driven anomaly detection stands as a critical line of defense. By continuously monitoring network traffic, AI systems can identify deviations from normal behavior, signaling potential cyber threats. These anomalies trigger alerts, allowing for immediate investigation and response. Not all detection methods are equal; AI-based solutions often combine supervised machine learning and deep learning to enhance their effectiveness.
Anomaly detection is not just about recognizing threats, but also about responding to them efficiently. AI enables rapid analysis of the threat landscape, facilitating a swift and informed reaction to cyber attacks. This is particularly vital in Operational Technology (OT) and Industrial Control Systems (ICS), where security breaches can have severe consequences.
AI's capability to adapt to new and emerging threats by learning from past incidents ensures that network security is continuously evolving. This dynamic approach is essential for staying ahead of sophisticated cyber attackers.
The integration of AI in cybersecurity has led to the development of robust systems that not only detect but also predict potential security incidents. This predictive maintenance is crucial for preemptive action, minimizing the impact of attacks and maintaining network integrity.
Strengthening Cybersecurity with AI Algorithms
The integration of Artificial Intelligence (AI) into network security has revolutionized the way cybersecurity defenses are constructed and maintained. AI-based cyber analytics systems not only detect threats but also respond to them intelligently. They use adaptive algorithms and artificial intelligence to analyze network traffic patterns, identifying anomalies and suspicious activities that could indicate a cyber attack.
By employing AI algorithms, networks become more resilient to the sophisticated tactics used by cybercriminals. These systems can preemptively identify potential breaches and abnormal activities, ensuring a proactive defense mechanism is in place. The ability to mitigate threats in real-time is a significant advancement over traditional security measures, which often rely on reactive approaches.
The proactive approach to security is essential in safeguarding networks against the ever-evolving threats found in today's digital landscape, making it an area where the benefits of AI are especially compelling.
Furthermore, AI in networking frees up valuable time for network administrators, allowing them to focus on strategic initiatives that drive business growth and enhance customer outcomes. This shift from routine tasks to more complex, value-added activities is a key benefit of integrating AI into cybersecurity strategies.
Autonomous Networking: The Future of Self-Optimizing Networks
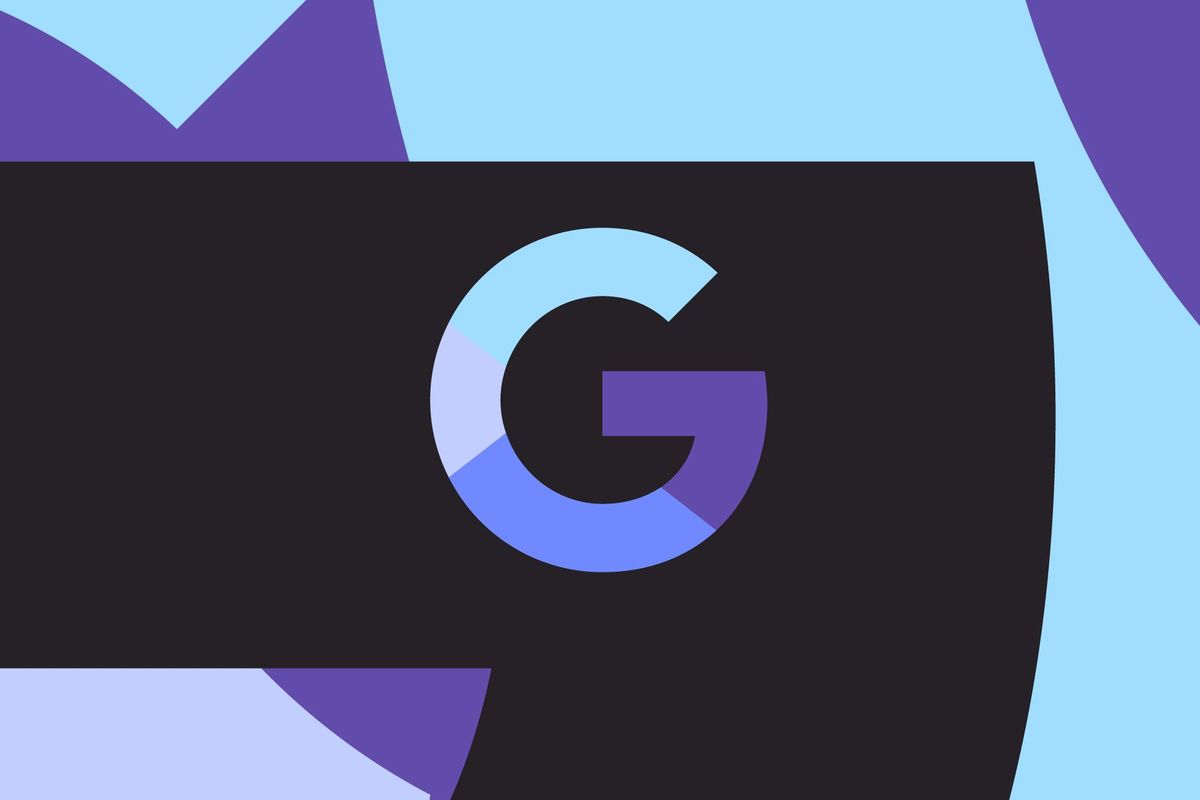
Intelligent Decision-Making without Human Intervention
The advent of AI in networking has paved the way for systems capable of making intelligent decisions autonomously. Autonomous networks are now a reality, where AI-driven systems continuously learn and adapt to optimize network performance. These systems can manage complex tasks such as resource allocation and traffic engineering with minimal human oversight.
AI revolutionizes telecommunications by automating network management, enhancing security, and enabling personalized customer service. Predictive maintenance, a key application of AI, optimizes network performance and efficiency, ensuring that the network is always running at its best.
The synergy between AI and autonomous networking creates a robust framework for self-optimization. This not only improves operational efficiency but also significantly reduces the need for manual intervention in routine tasks.
The following list highlights the core benefits of intelligent decision-making in AI networks:
- Enhanced network reliability and uptime
- Reduced operational costs through automated processes
- Improved customer satisfaction with better service quality
- Swift adaptation to changing network conditions and user demands
Continuous Learning and Network Adaptation
In the realm of autonomous networking, continuous learning is a cornerstone that ensures networks remain efficient and up-to-date with the latest technological trends. By leveraging adaptive neural networks, these systems can scale and evolve, interpreting vast amounts of data to optimize performance and resource management.
The ability to self-optimize through continuous learning and adaptation is not just a futuristic concept; it's a present-day reality that drives operational efficiency and strategic growth.
With the integration of AI, networks can proactively learn from each interaction, adapting to new conditions and demands. This results in a dynamic environment where network administrators are relieved from routine tasks, enabling them to concentrate on strategic initiatives that enhance customer experiences and foster business expansion.
- Predictive analytics for early issue detection
- Real-time optimization of resource allocation
- Dynamic adaptation to changing network demands
The integration of continuous learning mechanisms within networking systems is a testament to the adaptability and resilience of AI-driven technologies. It exemplifies how networks can not only react to changes but also anticipate and prepare for future shifts, ensuring sustained performance and reliability.
Resource Allocation and Traffic Engineering Automation
The integration of AI into networking has revolutionized the way resources are allocated and traffic is engineered. AI-driven systems dynamically adjust to network conditions, ensuring optimal performance and efficiency. By analyzing traffic patterns and predicting future demands, AI enables networks to be proactive rather than reactive.
Resource allocation and traffic engineering are now more agile, with AI providing the ability to adapt to real-time changes in network traffic. This results in a more resilient network infrastructure capable of handling unexpected surges or declines in demand without human intervention.
The automation of these processes through AI not only saves time but also reduces the potential for human error, leading to a more reliable network ecosystem.
The benefits of AI in networking are clear, from improved network optimization to enhanced user experiences. As AI continues to learn and adapt, the future of networking looks to be one where networks self-optimize in an ever-changing digital landscape.
Integrating AI with Edge Computing
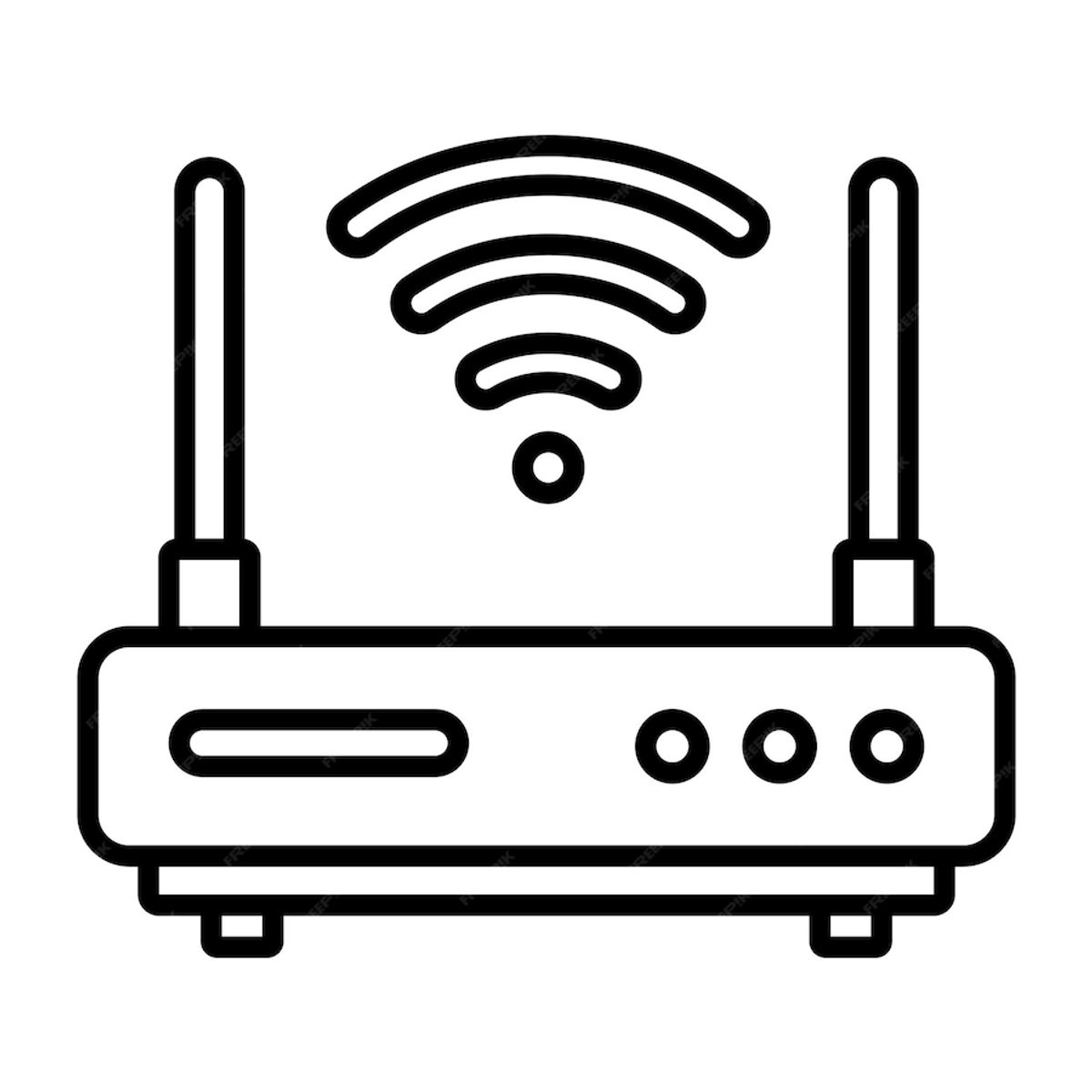
Optimizing Data Processing in Edge Architectures
In the realm of edge computing, AI networking plays a pivotal role by distributing computational tasks across edge devices and centralized servers. This strategic allocation not only minimizes latency but also reduces bandwidth usage, which is crucial for the performance of edge computing applications.
By leveraging AI to intelligently manage data processing, edge architectures can operate more efficiently, paving the way for advanced applications that require real-time analytics and decision-making.
The integration of AI with edge computing also involves the optimization of hardware resources. Power sharing in blade systems, for instance, improves PUE (Power Usage Effectiveness), enabling energy-efficient computing tailored for AI applications. This synergy between AI and edge computing is not just a technical enhancement; it's reshaping industries and daily life with its advanced hardware evolution.
The benefits of AI in edge computing are manifold, including:
Minimizing Latency and Bandwidth Usage
In the realm of AI networking, minimizing latency and bandwidth usage is crucial for maintaining high-performance connectivity. AI-driven optimization of network resources is pivotal, particularly in scenarios with complex traffic patterns or fluctuating demands. By dynamically allocating bandwidth and prioritizing traffic, AI algorithms ensure efficient network operation and superior user experiences.
Latency reduction is not just about speed; it's about the responsiveness of the network to the needs of the users and applications. For instance, Intel's Programmable Solutions Group (PSG) is set to launch new radio macro and mMIMO Enablement Packages, alongside Intel Precision Time Protocol Servo, to provide dynamic, low-latency solutions.
The integration of AI in networking equipment is transforming the landscape, leading to significant energy and cost savings. This is exemplified by the performance improvements in 5G core workloads and the anticipated broad availability of Intel Infrastructure Power Manager software, which aims to reduce CPU power while maintaining performance metrics.
The following table summarizes the benefits of AI in minimizing latency and bandwidth usage:
Benefit | Description |
---|---|
Enhanced Responsiveness | AI algorithms adjust in real-time to user demands, reducing latency. |
Efficient Resource Allocation | Dynamic bandwidth allocation prevents congestion and optimizes throughput. |
Energy and Cost Savings | Advanced AI techniques lead to lower power consumption and operational costs. |
Enhancing Edge Computing Application Performance
The integration of AI with edge computing is a transformative approach that significantly boosts application performance. By leveraging AI's capabilities, edge computing architectures can distribute computational tasks more effectively, leading to reduced latency and optimized bandwidth usage. This strategic distribution ensures that edge applications run more smoothly and responsively, providing a seamless user experience.
Edge Computing Integration plays a pivotal role in supporting the dynamic nature of edge architectures. It allows for intelligent task distribution between edge devices and centralized servers, which is crucial for maintaining high performance levels. As a result, businesses can recognize greater energy and cost savings, contributing to a substantial improvement in performance per rack, especially for demanding workloads like 5G core operations.
The combination of AI and edge computing unlocks new opportunities for businesses across various sectors and paves the way for innovative applications.
The right platform is essential for scaling AI and edge solutions efficiently. Enterprises are now leveraging the vast amounts of data generated at the edge to deliver new intelligent services, enhance customer experiences, and scale operations through automation. This not only makes them more competitive in terms of pricing but also helps them address labor shortages, marking a new era of opportunity for edge AI.
Conclusion
The integration of AI into networking equipment marks a significant milestone in the evolution of connectivity. As we have explored, AI Networking is set to transform the way data is managed and transmitted, offering enhanced performance, reliability, and security. The proactive capabilities of AI algorithms to predict and mitigate issues before they arise, coupled with their role in optimizing network resources, are pivotal in meeting the demands of modern digital landscapes. While challenges such as data privacy, algorithmic biases, and interoperability must be navigated, the collaborative efforts of industry leaders, researchers, and policymakers are paving the way for a smarter, more efficient, and secure networked future. The synergy between AI and networking is not just a technological advancement; it is the foundation for a connected world enriched by the possibilities of 5G, IoT, and other emerging technologies.
Frequently Asked Questions
How does AI networking enhance network performance and security?
AI networking leverages advanced algorithms and machine learning techniques to analyze vast amounts of data in real-time, identify patterns, predict network behavior, and proactively address potential issues. This improves network uptime, enables dynamic adaptation, and strengthens cybersecurity by identifying and mitigating threats swiftly.
What role does AI play in dynamic bandwidth allocation and traffic prioritization?
AI algorithms optimize network resources by dynamically allocating bandwidth and prioritizing traffic based on real-time data analysis. This leads to improved network efficiency and better user experiences, especially in environments with fluctuating demands or complex traffic patterns.
Can AI-driven autonomous networking operate without human intervention?
Yes, AI-driven autonomous networking systems are designed to make intelligent decisions and self-optimize without human intervention. They continuously learn and adapt, managing complex tasks like resource allocation, routing optimization, and traffic engineering autonomously.
How does AI networking contribute to the advancement of edge computing?
AI networking supports edge computing architectures by optimizing data processing and communication between edge devices and servers. By intelligently distributing computational tasks, AI minimizes latency and bandwidth usage, enhancing the performance of edge computing applications.
What challenges does AI networking face, and how can they be addressed?
AI networking faces challenges such as data privacy concerns, algorithmic biases, and interoperability issues. Addressing these requires interdisciplinary collaboration, robust regulatory frameworks, and a commitment to ethical and responsible deployment of AI technologies in networking.
How will AI impact the future of networking with emerging technologies like 5G and IoT?
AI is set to significantly impact networking by enhancing network intelligence and enabling seamless integration with emerging technologies such as 5G and IoT. As AI evolves, it will drive advancements in algorithms and networking, leading to a smarter, more efficient, and secure connected world.