Innovations in Healthcare: AI and Biotechnology
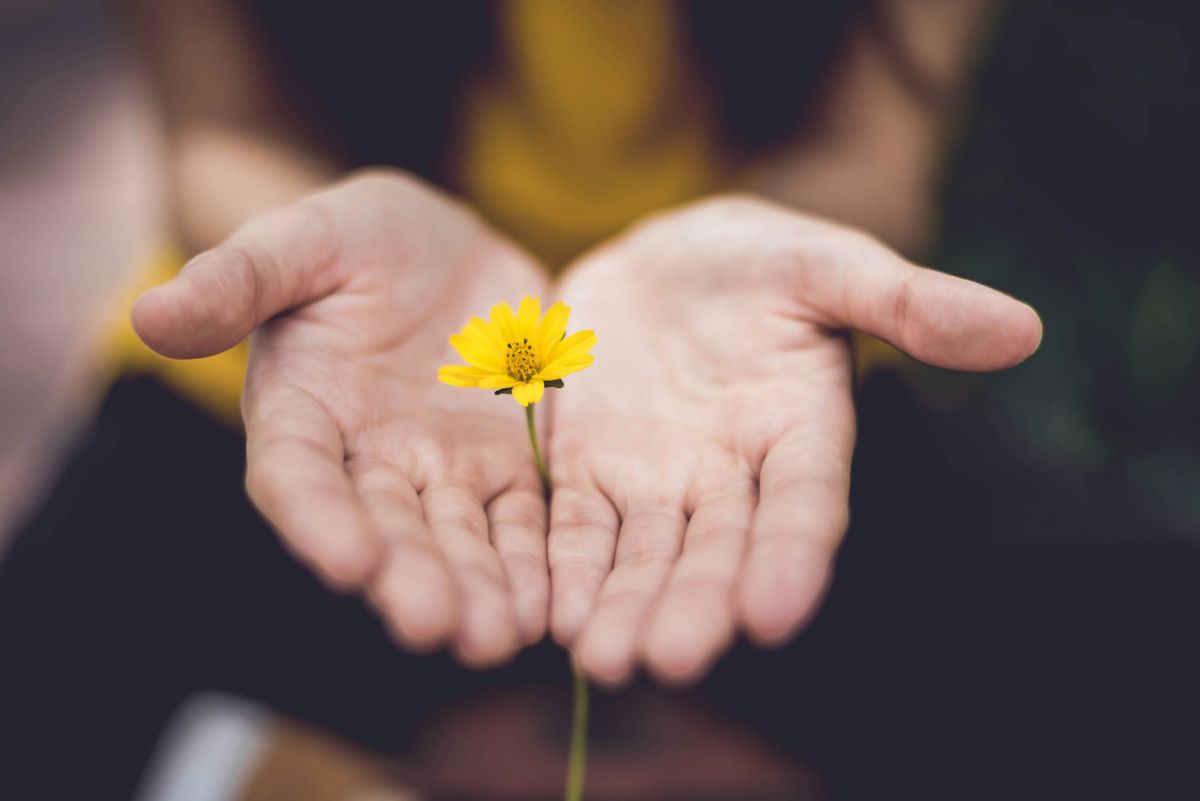
The field of healthcare is experiencing significant advancements with the integration of artificial intelligence (AI) and biotechnology. These innovations are revolutionizing the way healthcare is delivered and improving patient outcomes. From drug discovery to medical imaging, AI and biotechnology are reshaping the landscape of healthcare. Let's delve into the key takeaways from these groundbreaking developments:
Key Takeaways
- AI is transforming drug discovery by mapping the chemical space.
- New approaches in clinical trials powered by AI are leading to better results.
- Proprietary AI in medical coding is saving years of manual work.
- AI is reducing the burden on radiologists in medical imaging, improving efficiency and accuracy.
- Ethical concerns and resistance to AI adoption in healthcare are important factors that need to be addressed for the successful integration of AI technologies.
Innovations in Healthcare: AI and Biotechnology
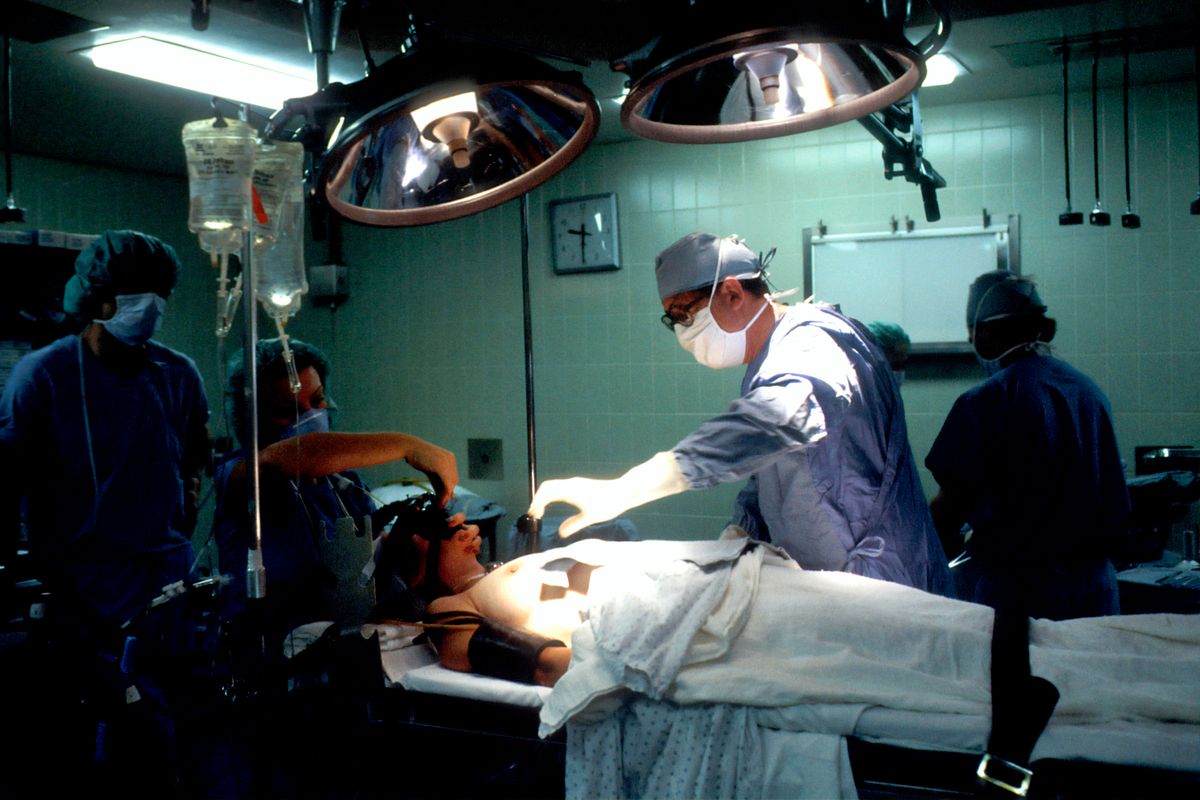
Developing and Deploying AI in Healthcare
The integration of artificial intelligence (AI) into healthcare is transforming the industry by enhancing clinical decision-making, optimizing operational efficiencies, and improving patient outcomes. Developing and deploying AI in healthcare requires a multifaceted approach, involving the creation of sophisticated algorithms, the management of large datasets, and the seamless incorporation of AI tools into existing healthcare workflows.
Healthcare organizations are increasingly turning to AI to address a range of challenges, from diagnostic accuracy to cost savings and patient satisfaction. In the United States, significant investment is being channeled into AI research and development, signaling a strong commitment to advancing this technology within the healthcare sector.
Adoption of AI in healthcare is not without its challenges. Ethical considerations, such as data privacy and potential job automation, are at the forefront of the discourse. Moreover, resistance from healthcare leaders can lead to uneven adoption rates. Despite these hurdles, the potential benefits of AI in healthcare are too compelling to ignore, particularly in developing nations where AI can reduce the need for outsourcing and enhance patient care.
The ability of AI to adjust course and provide tailored solutions is crucial for improving patient experiences and outcomes, especially in regions with limited healthcare resources.
Ethical Concerns in AI Practice
The integration of AI into healthcare brings forth a myriad of ethical considerations. AI in healthcare must prioritize human interactions, necessitating a foundation of responsibility and ethics in AI's creation, testing, deployment, and ongoing management. Ethical AI in healthcare focuses on responsibility, trust, and safety, ensuring that the technology serves to enhance, not undermine, the patient-care provider relationship.
Key ethical issues include:
- Data privacy and security
- Algorithmic bias
- Sustaining patient autonomy and informed consent
- Changing responsibilities of healthcare professionals
- The need for regulations to ensure the ethical use of AI
Algorithmic bias is a particularly pressing concern, as it can lead to unequal treatment and access to healthcare services. To mitigate such risks, AI must be trained on diverse and thoroughly verified datasets. Moreover, continuous monitoring and the establishment of ethical guidelines are essential to ensure AI's equitable application in healthcare.
The ethical deployment of AI in healthcare is not just a technical challenge but a moral imperative. It requires a concerted effort from all stakeholders to uphold the principles of respect for autonomy, beneficence, non-maleficence, and justice as outlined by the Office for Civil Rights.
The protection of patient rights, such as the right to informed consent and medical data protection, must remain paramount. As AI systems become more prevalent, the healthcare industry must navigate these ethical waters with care to maintain the trust and safety of all patients.
Resistance to AI Adoption in Healthcare
Despite the potential of AI to revolutionize healthcare, its adoption faces significant resistance. Healthcare leaders often exhibit skepticism, slowing down the integration of AI technologies into clinical practice. This reluctance stems from various concerns, including the protection of patient rights, such as informed consent and medical data privacy.
The introduction of AI also brings new risks, like algorithmic bias and machine morality issues, which could compromise patient outcomes. Moreover, the public's discomfort with sharing personal data for AI development is a notable barrier. A UK survey revealed that a significant majority of the population is uneasy about this trade-off.
The scarcity of real, accessible patient data hinders the progress of AI in healthcare, making it a critical point of contention for both developers and the public.
To address these challenges, ongoing research and ethical considerations are crucial for developing and deploying more artificial intelligence in healthcare responsibly.
AI in Drug Discovery
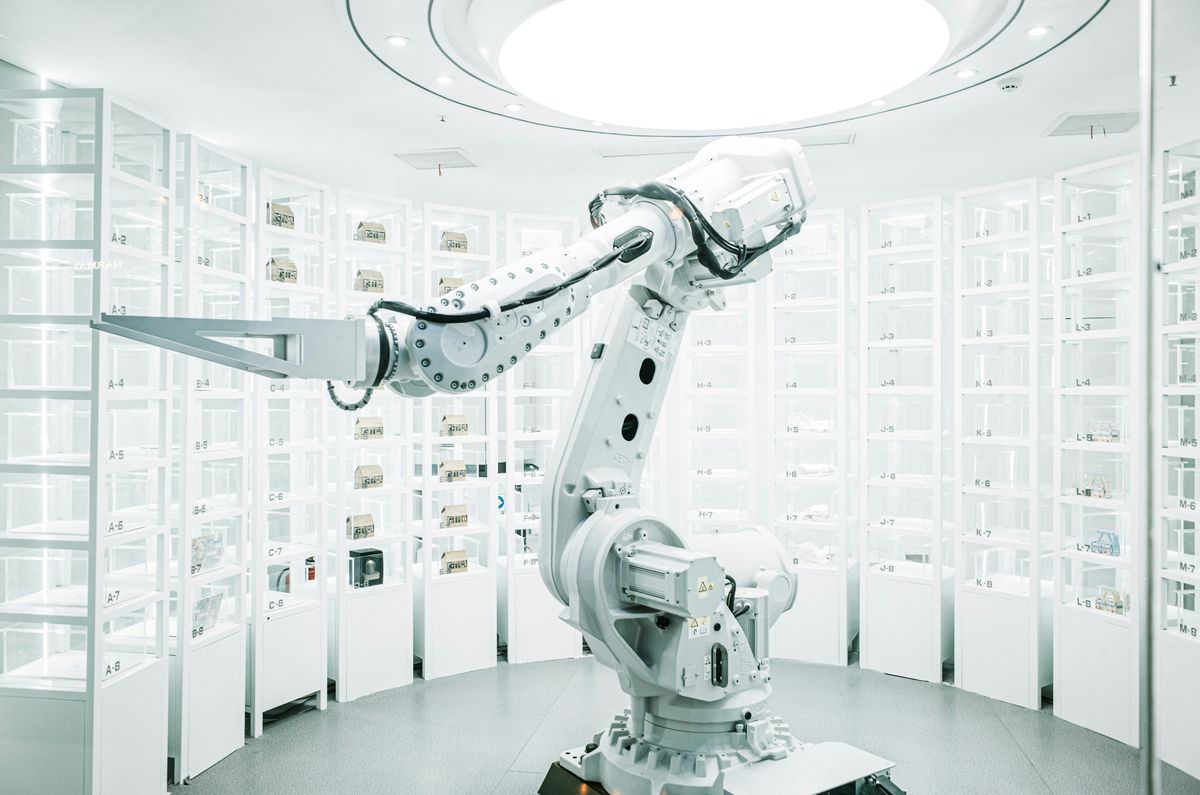
Mapping the Chemical Space
The quest to revolutionize drug discovery has led to the utilization of artificial intelligence (AI) in mapping the chemical space. This process involves the screening of vast libraries of small molecules to find potential drug candidates that bind to specific disease-related targets. AI accelerates this search by predicting the biological activity of compounds, thereby reducing the need for extensive laboratory testing.
- Chemoproteomics
- PROTAC's
- RNA-targeting SMOLs
AI's predictive capabilities are not just limited to identifying compounds but also extend to optimizing drug properties, such as solubility and efficacy. This optimization is crucial in developing medications that are not only potent but also safe and manufacturable at scale.
By leveraging AI, researchers can navigate the complex landscape of chemical compounds more efficiently, bringing new treatments to patients faster.
The integration of AI in drug discovery is a testament to the innovation powered by people who strive to push the boundaries of medical science. It represents a significant step towards a future where personalized medicine and targeted therapies become the norm, offering hope for conditions once deemed undruggable.
Clinical Trials
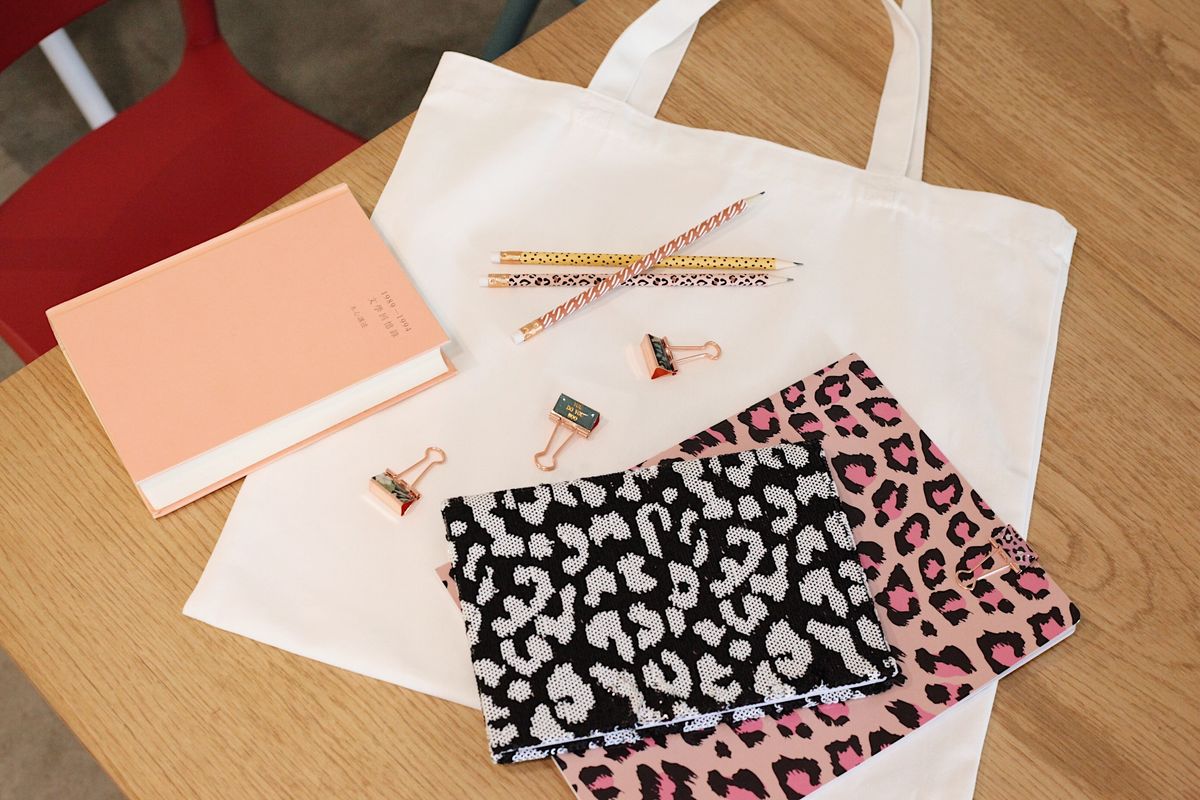
New Approaches
The advent of AI in clinical trials is revolutionizing the way we approach drug development. AI algorithms can predict potential complications related to diabetes and heart conditions by analyzing historical patient data, which aids healthcare providers in tailoring interventions more effectively.
Innovative methods such as symbolic reasoning, deep learning, and Bayesian networks are being integrated into clinical trial design. These approaches enhance the predictive power and efficiency of trials, leading to faster patient access to new treatments.
The potential of AI to transform clinical trials extends beyond mere data analysis. It offers a dynamic evaluation approach, enabling real-time adjustments and more personalized patient care.
The table below summarizes the various AI approaches and their applications in healthcare:
AI Approach | Application in Healthcare |
---|---|
Symbolic | Personalized treatment plans |
Deep Learning | Predictive analytics |
Bayesian Networks | Risk assessment |
Evolutionary Algorithms | Drug formulation |
By embracing these new approaches, the healthcare industry is poised to overcome traditional barriers and accelerate the journey from laboratory to patient.
Better Results
The integration of artificial intelligence in clinical trials is revolutionizing the way we approach drug development. AI-driven analytics are enhancing the precision of trials, leading to more reliable outcomes and a reduction in the time and resources required. By leveraging AI, researchers can better predict patient responses and optimize trial design.
One of the key benefits of AI in clinical trials is the ability to analyze vast amounts of data quickly. This capability allows for a more nuanced understanding of patient populations and the efficacy of treatments. For instance, AI algorithms can identify subtle patterns in patient data that might indicate a higher risk of adverse reactions, enabling more targeted and safer clinical trials.
The use of AI in clinical trials is not just about efficiency; it's about achieving better health outcomes and bringing life-saving treatments to patients faster.
The following table illustrates the impact of AI on clinical trial outcomes:
Metric | Before AI | With AI |
---|---|---|
Trial Duration | 10 years | 7 years |
Patient Recruitment Time | 24 months | 18 months |
Adverse Events | 15% | 10% |
As we continue to refine AI technologies, the potential for improved patient outcomes and more efficient healthcare delivery becomes increasingly clear.
Medical Coding
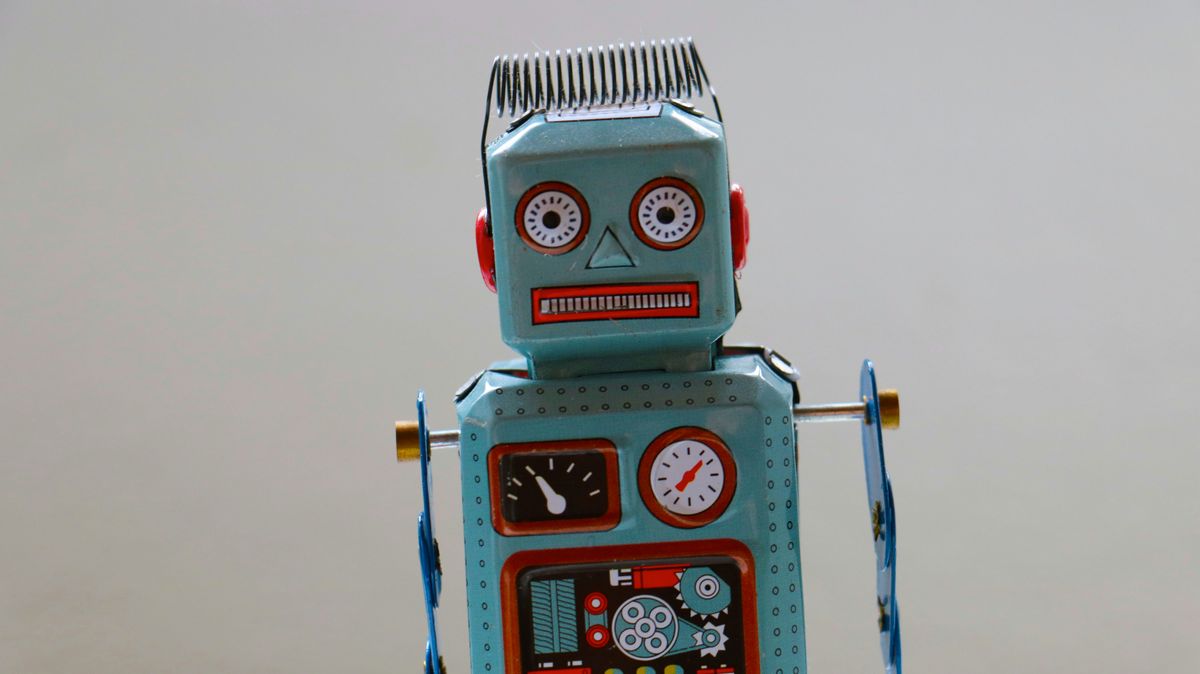
Proprietary AI Saves Manual Work
The integration of proprietary AI systems in medical coding is transforming the landscape of healthcare administration. AI in healthcare revolutionizes diagnostics and treatment with sophisticated tools for faster, accurate diagnoses. Personalized medicine advances with tailored treatments based on individual characteristics and genetic data.
The use of AI in medical coding automates the translation of healthcare diagnoses, procedures, medical services, and equipment into universal medical alphanumeric codes. This automation significantly reduces the time and effort required for manual coding, which can span years of work for complex cases.
The benefits of AI-driven medical coding include:
- Enhanced accuracy and consistency in coding
- Reduced operational costs
- Minimized billing and coding errors
- Improved compliance with healthcare regulations
- Faster reimbursement processes for healthcare providers
Medical Imaging
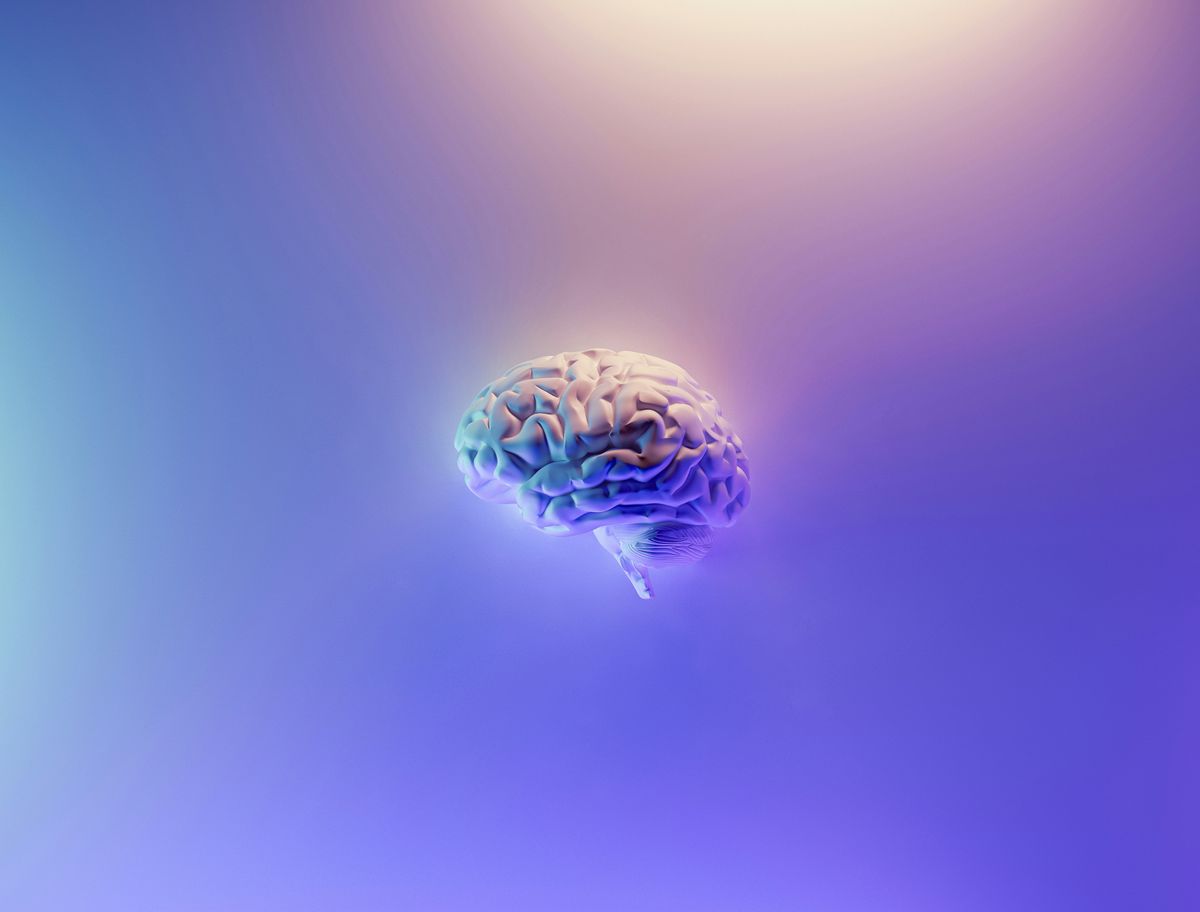
Reducing Burden on Radiologists
The integration of AI into medical imaging is transforming the role of radiologists by automating time-consuming tasks and accelerating workflows. This not only enhances the detection capabilities but also significantly reduces the radiologists' workload. As a result, medical teams can support a greater number of patients with faster, treatment-critical answers.
AI's capability in medical imaging is not just about matching the accuracy of healthcare professionals in disease identification; it also brings non-interpretive benefits. For instance, AI can reduce noise in images, create high-quality images from lower doses of radiation, and automatically assess image quality. These advancements are crucial in fields like nuclear medicine where precision is paramount.
The use of AI in radiology extends to the processing of large and diverse data sets. Through machine learning algorithms and deep learning, AI can triage and interpret traditional radiographs, providing valuable insights. The potential for AI to assist in the triage and interpretation of X-ray pictures is especially significant, given their prevalence in radiology departments.
The promise of AI in healthcare is to enable personalized treatment plans, improve patient care through real-time monitoring and predictive analytics, and support medical professionals in making informed decisions.
Conclusion
In conclusion, the integration of artificial intelligence and biotechnology in healthcare has paved the way for significant advancements in medical research, diagnosis, treatment, and patient care. The use of AI algorithms and technologies has revolutionized the healthcare industry, enabling more efficient processes, improved outcomes, and personalized medicine. As we continue to explore the potential of AI and biotechnology, it is essential to address ethical concerns, data privacy issues, and ensure widespread adoption across healthcare systems. The collaboration between experts in AI, biotechnology, and healthcare is driving a positive change towards a more interconnected and innovative healthcare ecosystem. The future of healthcare looks promising with the continued development and deployment of AI and biotechnological solutions.
Frequently Asked Questions
What are the key advancements enabling the growth of AI applications in healthcare?
Medical and technological advancements over the years have enabled the growth of AI applications in healthcare, including developments in drug discovery, personalized medicine, disease diagnosis, genomics, bioprocessing, and agricultural biotechnology.
What are some ethical concerns related to AI practice in healthcare?
Ethical concerns in AI practice in healthcare include issues such as data privacy, automation of jobs, and representation biases. Research is ongoing to address these concerns and ensure responsible AI implementation.
Why is there resistance to AI adoption in healthcare?
Resistance to AI adoption in healthcare can stem from various factors, including concerns about the impact on traditional healthcare practices, uncertainties about AI technology reliability, and challenges in integrating AI systems into existing healthcare workflows.
How is AI used in drug discovery?
AI is utilized in drug discovery to map the chemical space, accelerate the pace of drug discovery, and optimize the development of new pharmaceutical compounds.
What role does AI play in medical coding?
AI in medical coding helps save time and effort by automating the process of assigning diagnostic and procedure codes to patient records, reducing the burden of manual work for healthcare professionals.
How does AI contribute to medical imaging?
AI in medical imaging enhances the interpretation of diagnostic images, reduces the burden on radiologists by assisting in image analysis, and improves the efficiency and accuracy of medical diagnostics.