Genetic Revolution: AI's Role in Biotech Innovations
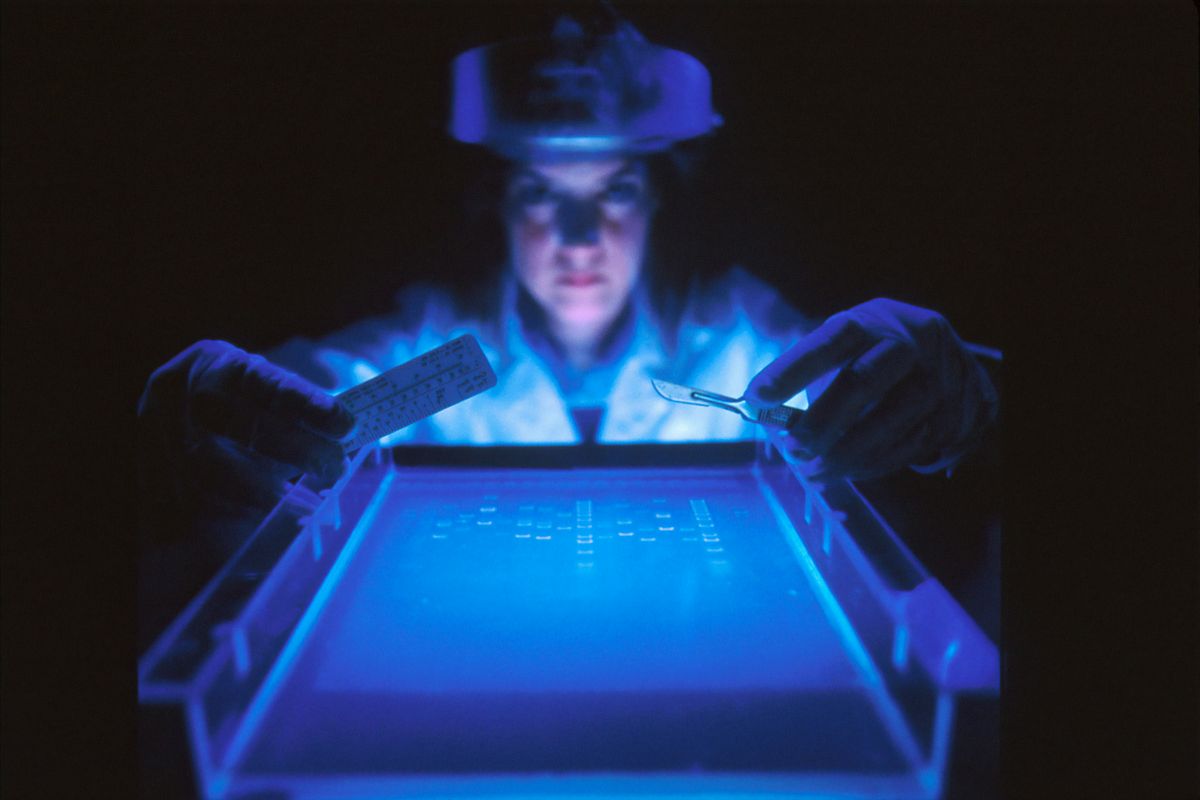
The fusion of artificial intelligence (AI) and biotechnology heralds a new era of innovation, promising to transform the landscape of healthcare, drug discovery, and beyond. As AI-driven tools become increasingly integrated into biotech research and development, they are accelerating the pace of breakthroughs, enhancing precision in diagnostics, and reshaping the commercialization of biotech products. However, amidst this genetic revolution, ethical and environmental considerations emerge, challenging us to navigate the complex interplay between technology and biology responsibly.
Key Takeaways
- AI is revolutionizing drug discovery by enabling the rapid development of molecular structures and facilitating the creation of 'AI-first' biotech companies.
- Machine learning in biotech is not only expediting research but also optimizing the commercial lifecycle of pharmaceuticals, from lab to market.
- Synthetic datasets and generative AI are enhancing data sharing and fueling inverse design, leading to breakthroughs in treatment discovery.
- AI is instrumental in advancing precision medicine, with applications like computer vision for cancer detection and CRISPR for gene editing.
- While AI in biotech offers immense potential for climate change mitigation and healthcare, it also raises significant ethical and surveillance concerns.
The Intersection of AI and Biotech: Pioneering Drug Discovery
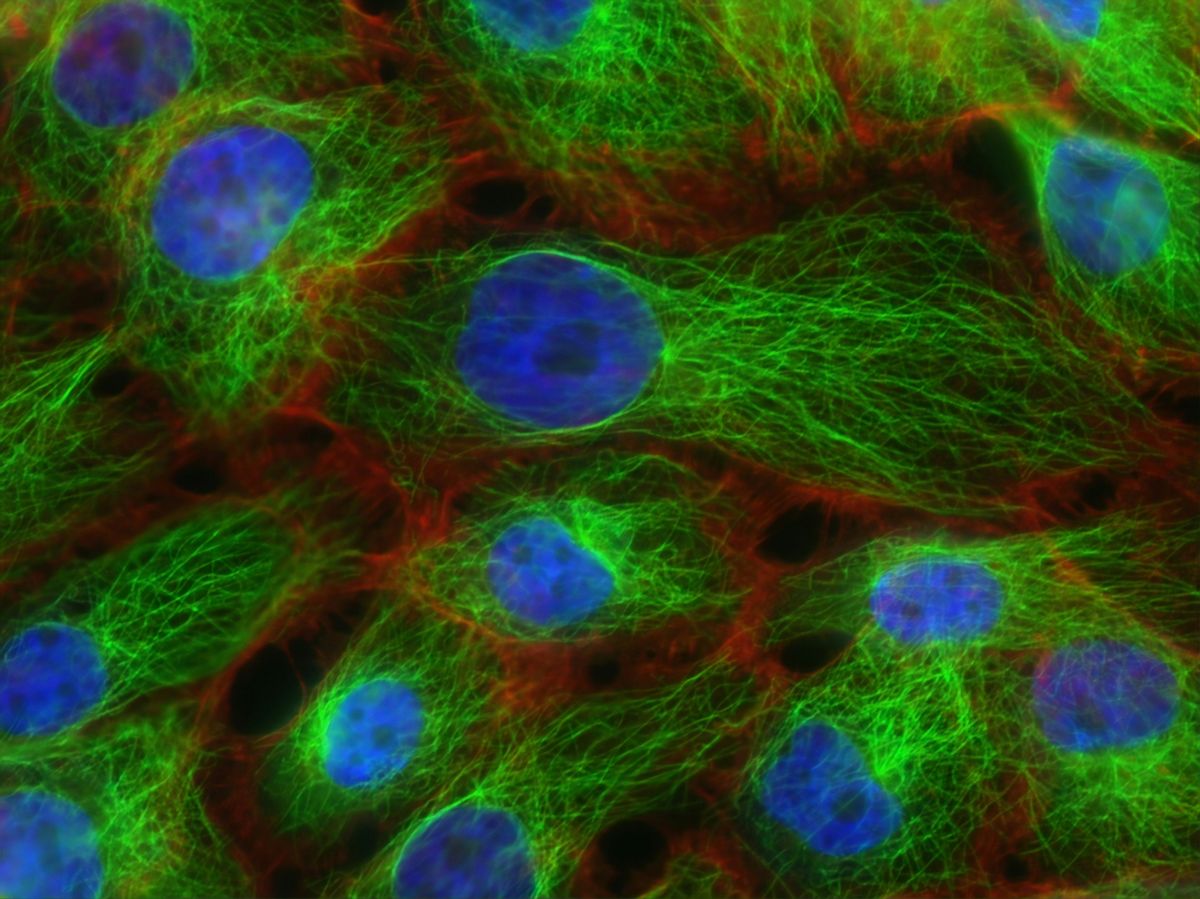
AI-Enabled Drug Discovery
The advent of AI in biotech is reshaping the landscape of drug discovery, offering unprecedented speed and precision in identifying new therapeutic candidates. By leveraging machine learning algorithms, researchers can now analyze vast datasets to predict molecular behavior and optimize drug efficacy and safety with greater accuracy than ever before.
- AI-driven platforms are capable of simulating millions of chemical combinations, streamlining the early stages of drug development.
- Advanced AI systems assist in precision medicine, tailoring treatments to individual genetic profiles.
- Investment in AI-first biotech companies has surged, reflecting confidence in AI's transformative potential.
AI is transforming the pharmaceutical industry by enhancing drug discovery, development, and personalized medicine through data analysis and efficiency improvements.
The integration of AI tools in the drug discovery process not only accelerates the development of new drugs but also opens the door to more personalized treatment strategies. As AI continues to evolve, its role in biotech promises to unlock new frontiers in healthcare innovation.
Machine Learning in Chemical Analysis
The integration of machine learning in chemical analysis is a transformative force in biotechnology. By harnessing the power of AI, researchers can now predict the optimal combination of chemicals for drug development, significantly reducing the time and resources required for manual experimentation. This approach not only streamlines the research process but also enhances the precision of the outcomes.
Machine learning algorithms excel at identifying patterns and predicting properties of chemical compounds. This capability is crucial for optimizing drug properties and tailoring medications to specific patient needs. For instance, AI can analyze vast datasets to determine which chemical combinations are most likely to yield effective and safe drugs.
AI optimizes drug properties, accelerates drug discovery, and revolutionizes clinical trials with personalized treatments and predictive analytics, advancing healthcare innovation.
The impact of AI in chemical analysis extends beyond the lab. It plays a pivotal role in the entire lifecycle of a chemical compound, from discovery to commercialization. Here's how AI is reshaping the journey of a chemical compound:
- Predicting market demand for specific chemicals or medicines.
- Assisting in the development of molecular structures tailored to the target market.
- Industrializing drug discovery through TechBio companies like Recursion, which received a significant investment from NVIDIA.
The synergy between AI and chemical analysis is paving the way for a new era of biotech innovations, where the speed and accuracy of drug discovery are enhanced, and the potential for personalized medicine is unlocked.
Case Studies: AI-First Biotechs
The landscape of biotechnology is rapidly evolving with the integration of artificial intelligence. AI-first biotechs are pioneering this transformation, having centered their entire research and development around AI tools. These companies are not only reshaping the way drugs are discovered but are also redefining the investment landscape in the biotech sector.
An analysis of approximately 200 AI-first biotechs shows a staggering investment of over $18 billion in the past decade. This investment, however, is extremely targeted. A mere 20 of these companies have attracted 60% of the total funding, with a strong focus on disease understanding and small molecule applications. The table below illustrates the investment distribution among the top 20 AI-first biotechs, highlighting the concentration of funds in specific use case families.
Rank | Company | Investment (US$ Billion) |
---|---|---|
1 | Company A | 2.5 |
2 | Company B | 1.8 |
3 | Company C | 1.2 |
... | ... | ... |
20 | Company T | 0.5 |
The strategic allocation of investments is indicative of the high expectations placed on AI-driven methodologies to revolutionize drug discovery and development processes.
The trend is not limited to funding; it extends to the commercialization phase as well. AI-first biotechs are utilizing AI-based platforms to develop molecular structures tailored to the target market, streamlining the journey from the lab to the marketplace.
Beyond the Lab: AI's Impact on Biotech Commercialization
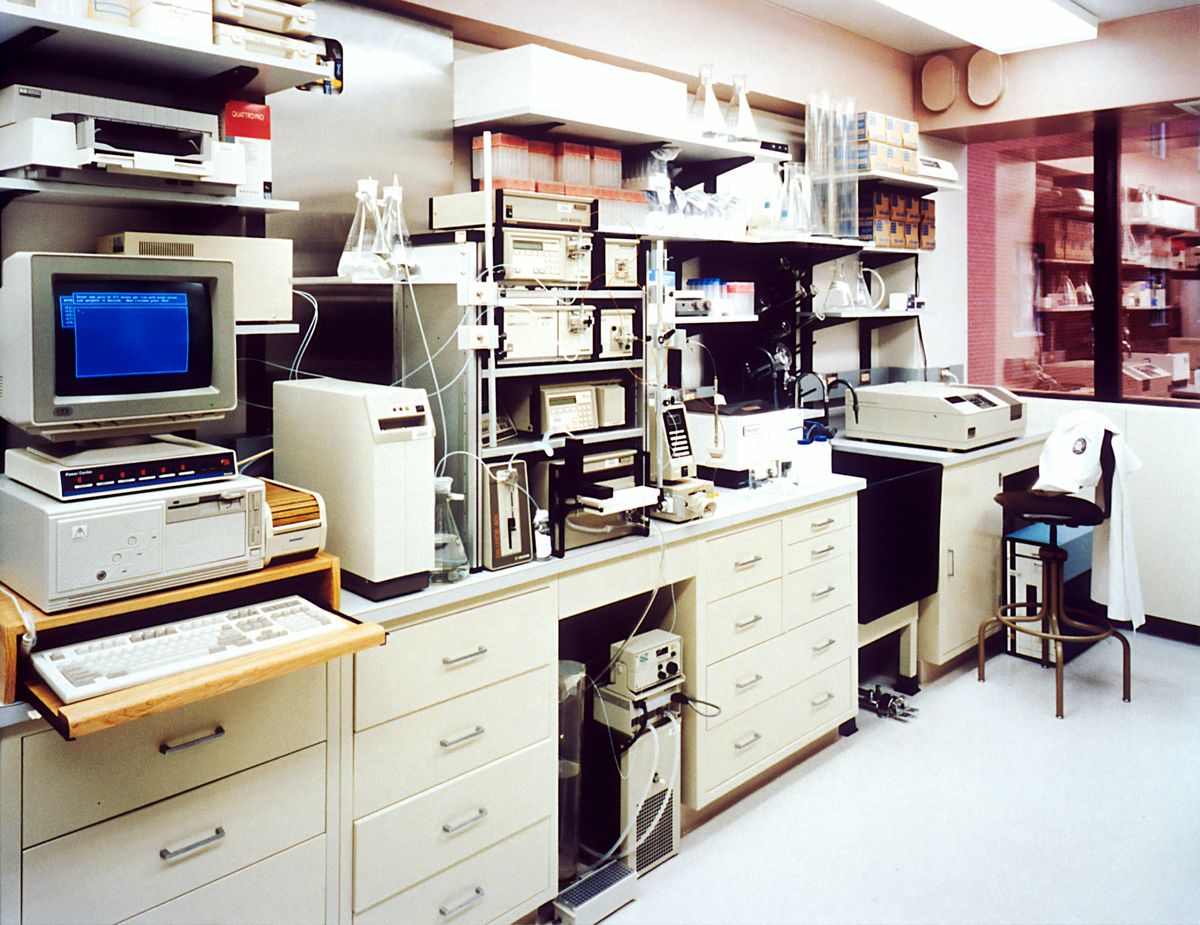
From Research to Market: The AI Journey
The transition from research to market is a critical phase in the lifecycle of biotech innovations, and AI is playing an increasingly pivotal role in this journey. Generative AI is transforming the way companies approach the commercialization of their products. By providing on-demand retrieval, summarization, and synthesis of data, these tools are enhancing the productivity of market analysts and accelerating the path to market.
To ensure a smooth transition, companies are adopting a structured approach to their AI journey. This includes the establishment of cross-functional leadership teams to address ethical AI principles and the creation of policies that align with the company's risk appetite. Such measures are crucial for maintaining transparency and consistency across the enterprise.
Moreover, the integration of AI into the commercialization process is not just about technology adoption; it's about preparing the workforce for new roles and opportunities. Companies are recognizing the importance of including employees in shaping the AI journey, which can lead to a radical rethink of value chains and business models.
The AI journey in biotech commercialization is not a solo endeavor. Collaborating with ecosystems can be a transformative route, leveraging disruptive technology to redefine industry standards.
Predictive Analysis for Market Demand
In the rapidly evolving biotech industry, predictive analysis is becoming an indispensable tool for companies aiming to stay ahead of market trends. By leveraging the power of machine learning and AI, firms can forecast product demands, assess equipment efficiency, and identify potential risks with unprecedented accuracy. This strategic approach not only streamlines the research-to-market journey but also ensures that resources are allocated efficiently to meet future demands.
The application of predictive analytics extends beyond mere sales forecasting. It facilitates a deeper understanding of consumer behavior, enabling companies to tailor their products to specific market needs. For instance, in the consumer packaged goods sector, pattern recognition through generative AI has proven crucial for scaling operations and enhancing customer accessibility to previously unstructured information.
Investment in predictive analytics is not just about technology; it's about gaining a strategic edge in a competitive landscape.
Furthermore, the insights gained from predictive analytics are not confined to marketing strategies. They have broad applications across various business functions, including HR, compliance, finance, and supply chain management. By recognizing patterns and applying them to different use cases, companies can optimize their operations and drive growth across the board.
Investment Trends in TechBio Companies
The biotech industry is witnessing a surge in investments, particularly in companies that integrate artificial intelligence (AI) into their operations. AI empowers companies in risk management, long-term investment strategies, and generative AI for chemical innovation, leading to transformative advancements in the industry. This influx of capital is not only fueling research and development but also reshaping the competitive landscape.
Investors are increasingly attracted to TechBio companies that demonstrate a strong AI foundation. For instance, a recent significant investment in a clinical-stage TechBio company aimed to accelerate the development of AI foundation models for chemistry and biology. These models are expected to be optimized and provided to other biotechnology firms, showcasing a trend towards collaborative growth and shared technological platforms.
The strategic deployment of AI in biotech is reshaping investment patterns, with a focus on sustainable, long-term growth and innovation.
The following table illustrates the investment per use case of the top 20 biotech companies:
Use Case | Investment (US$ Billion) |
---|---|
Drug Discovery | 5.2 |
Clinical Trials | 3.8 |
Data Analytics | 2.7 |
AI Development | 4.1 |
Other | 1.5 |
Source: CMS.wellcome.org
The data reflects a clear prioritization of AI-related initiatives, with drug discovery and AI development receiving the highest levels of investment. This trend is indicative of the industry's belief in the transformative potential of AI to lead the next wave of biotechnological breakthroughs.
Data-Driven Biotech: The Power of Synthetic Datasets
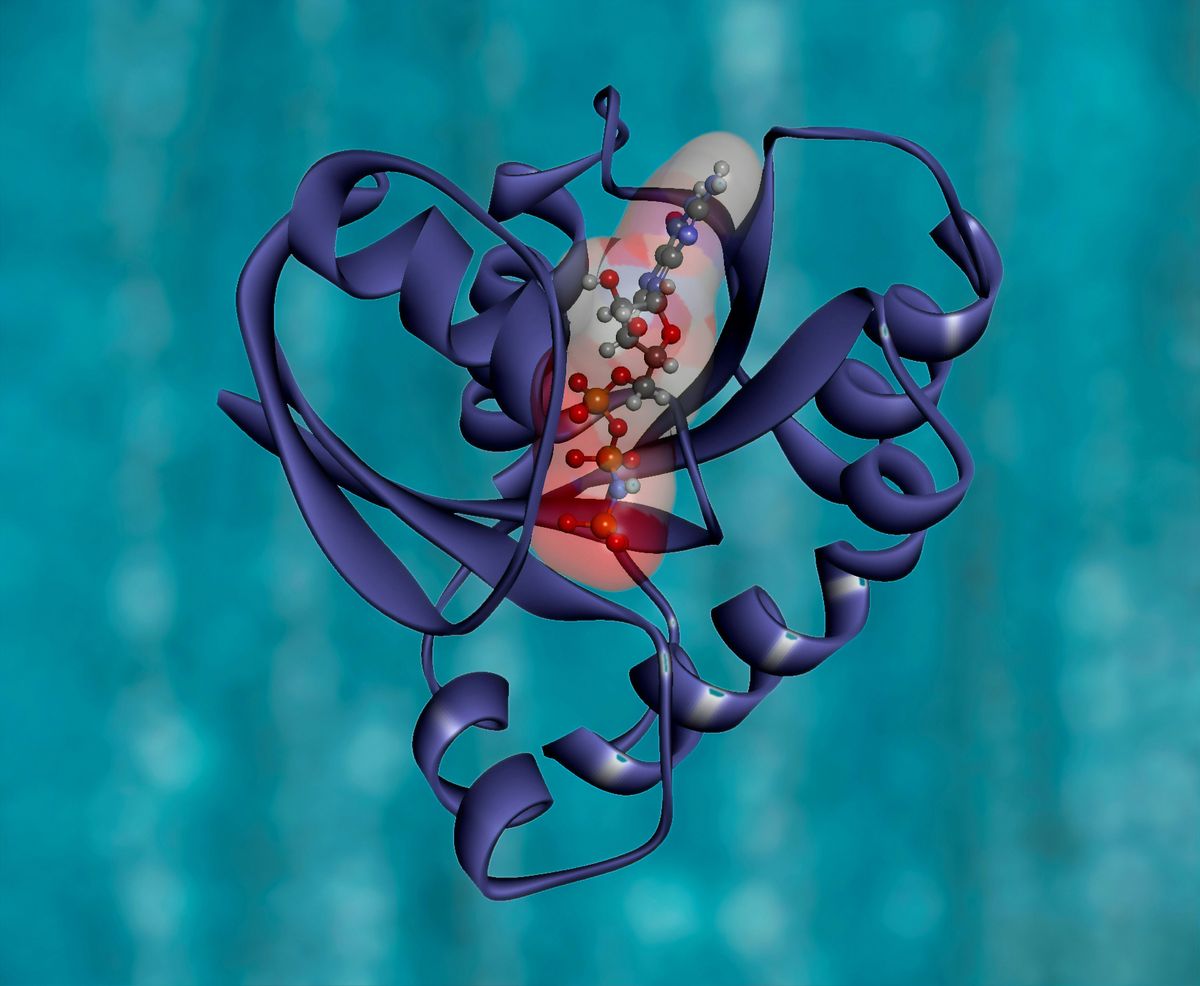
Enhancing Data Sharing in Healthcare
The integration of AI in healthcare has led to a paradigm shift in how medical data is shared and utilized. Data-pooling among healthcare organizations and their partners has created a larger pool of information, fostering medical breakthroughs and new opportunities for collaboration. This synergy is not only about sharing data but also about creating new business models and capturing value that leads to improved patient outcomes.
The challenges of data sharing in healthcare are significant, often hindered by privacy concerns, regulatory barriers, and the protection of intellectual property. However, the holy grail for healthcare and pharmaceutical firms is the ability to access patient records at scale to identify patterns for more effective treatments.
The advent of electronic medical records (EHRs) and the investment of $19 billion by the US government to expedite their adoption have provided the medical community with a wealth of data. This data enhances diagnosis and treatment, with a majority of acute care hospitals and office-based physicians now utilizing EHRs.
The potential of AI in life sciences, once limited by organizational silos, is now being unleashed through the creation of synthetic datasets. These datasets are statistically reliable and anonymized, enabling safe and secure data-sharing on a large scale. The result is a revolution in drug discovery and the ethical deployment of AI technology, with collaborative partnerships between Big Pharma and AI biotech firms leading the way.
Generative AI and Inverse Design
Generative AI is transforming the landscape of biotech innovation, particularly in the realm of de novo drug design. Generative models are capable of creating new molecular structures by learning from vast datasets of known compounds. This approach is not only accelerating the discovery process but also enabling the exploration of chemical spaces that were previously inaccessible.
The process of inverse design is particularly intriguing. Here, scientists present the AI with a specific biological target or desired property, and the AI generates potential compounds that could achieve the desired outcome. This method flips traditional drug discovery on its head, starting with the goal and working backward to the solution.
Generative AI's impact is not limited to drug discovery. Its versatility allows for applications across various biotech processes, including:
- Rapid prototyping and iteration of designs
- Enhancement of productivity in business processes
- Identification of novel applications through experimentation
The synergy between generative AI and inverse design is paving the way for unprecedented advancements in biotech, fostering a new era of personalized medicine and targeted therapies.
Privacy and Ethical Considerations
The integration of AI in biotechnology brings forth a myriad of privacy and ethical considerations that must be navigated with care. Ethical AI practices in biotechnology are crucial for innovation and societal values. AI's capability to analyze vast datasets can lead to groundbreaking medical discoveries, yet it also raises concerns about the confidentiality of sensitive patient information.
Advocate and enable ethical AI use. Organizations must establish clear guidelines for the ethical use of generative AI, ensuring fairness, transparency, and responsible AI practices. This includes creating policies that address the balance between innovation and privacy, such as the framework developed by PwC, which articulates approved tools, acceptable use cases, and restrictions on data input.
The holy grail of healthcare is the ability to access and analyze patient records at scale, identifying patterns for more effective treatments. However, the pursuit of this goal must not come at the expense of individual privacy rights and the ethical use of data.
The following list outlines key steps organizations can take to ensure ethical AI deployment:
- Define ethical AI principles and a governance model aligned with the enterprise strategy.
- Establish ethical foundations for technical robustness, compliance, and human-centricity of AI.
- Implement governance controls and an execution roadmap for embedding AI into operational processes.
Revolutionizing Healthcare: AI in Precision Medicine and Diagnostics
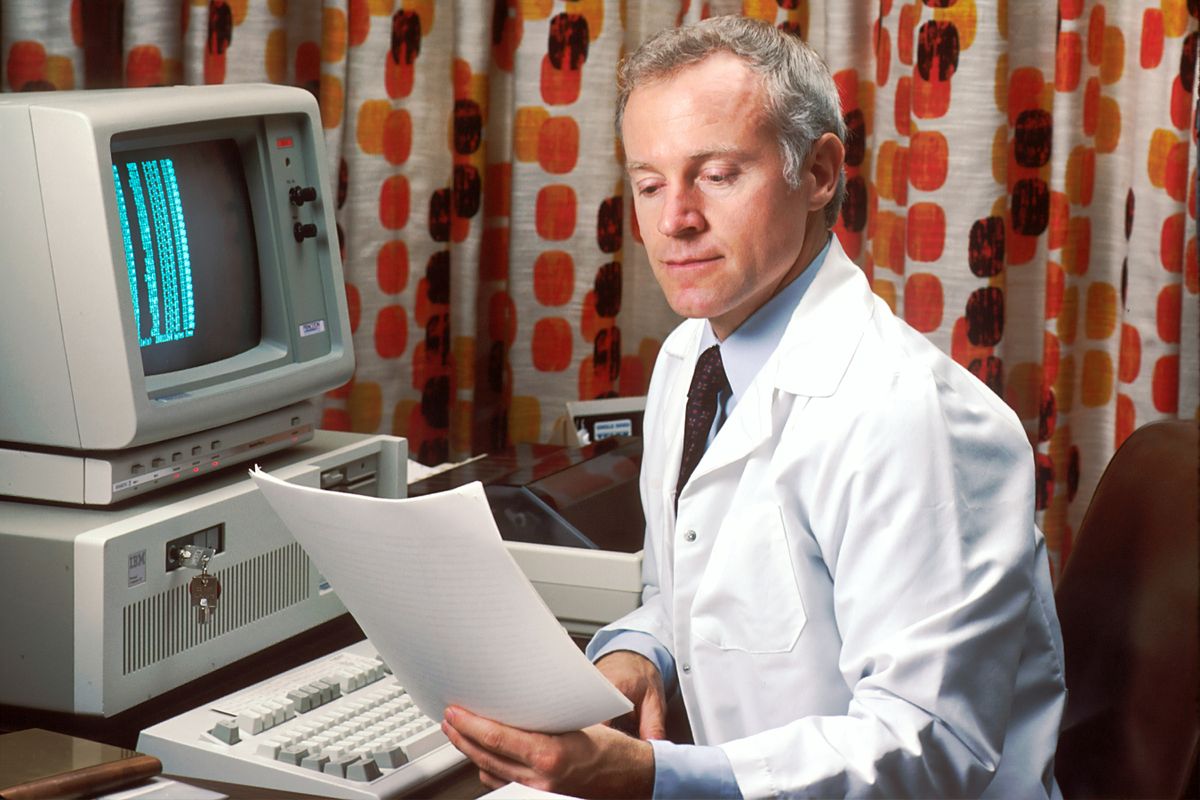
Computer Vision for Cancer Detection
The integration of AI in healthcare is transforming the landscape of diagnostics, with computer vision playing a pivotal role in the early detection of cancer. AI-driven diagnostic tools are enhancing the precision and speed of cancer screenings, leading to potentially life-saving early interventions.
Medical institutions are increasingly adopting AI technologies, such as generative AI models, to analyze medical scans for signs of cancer. This approach not only improves accuracy but also reduces the time required for diagnosis, allowing for quicker treatment planning.
AI in healthcare revolutionizes diagnostics and treatment with sophisticated tools for faster, more accurate diagnoses. Personalized medicine and patient care are enhanced through AI analysis of genetic data.
Collaborations between biotech companies and medical institutions are fostering advancements in this field. For example, the partnership between Agilent Technologies Inc. and Proscia's Concentriq Dx platform aims to deliver a comprehensive digital pathology system that integrates seamlessly with existing pathology workflows.
The potential of AI in cancer detection is further underscored by investments in TechBio companies, such as the $50 million invested by NVIDIA in Recursion, which is at the forefront of using AI for drug discovery and personalized medicine.
CRISPR and Gene Editing Breakthroughs
The advent of CRISPR technology has been a cornerstone in the field of genetic engineering, enabling precise edits to DNA sequences. This has opened up new possibilities for treating genetic disorders, such as the recent FDA-approved CRISPR-based treatment for sickle-cell disease. AI's integration with CRISPR has further accelerated the pace of these innovations, allowing for more efficient identification of genetic targets and prediction of outcomes.
CRISPR and AI together are not just about treating diseases; they are also about preventing them. AI in genetic testing and patient care revolutionizes personalized medicine, offering early interventions and tailored treatments for better health outcomes. The synergy between these technologies is charting a new course for healthcare, where interventions are not only reactive but also proactive.
The potential of CRISPR is not limited to human health. Its applications extend to agriculture, biofuels, and environmental management, showcasing its versatility and the breadth of its impact.
While the promise of CRISPR is immense, it is crucial to navigate the ethical landscape carefully. The misuse of gene editing could lead to unintended consequences, making governance and oversight paramount.
The Future of Personalized Treatment
The advent of precision medicine is a testament to the transformative power of biotechnology when fused with artificial intelligence. AI-powered systems are becoming integral in tailoring treatments to the individual's genetic profile, lifestyle, and environmental factors, heralding a new era in healthcare. This approach not only promises enhanced patient outcomes but also signifies a shift towards more cost-effective healthcare solutions.
Personalized medicine is not a distant dream but a burgeoning reality, with entities ranging from academic circles to biotech startups and pharmaceutical giants exploring its vast potential. Companies like Agilent Technologies are at the forefront, developing tools such as DNA sequencing and analysis, and collaborating with institutions like the Mayo Clinic to innovate early cancer detection methods.
The integration of AI in precision medicine is reshaping the landscape of treatment discovery and drug development, enabling a more nuanced understanding of individual health profiles.
The FDA's recent approval of CRISPR-based treatments for conditions like sickle cell disease exemplifies the strides being made in this field. AI's role extends beyond analysis, aiding in the synthesis of proteins and chemicals to create custom solutions for complex health issues.
Ethical and Environmental Implications of Biotech Innovations
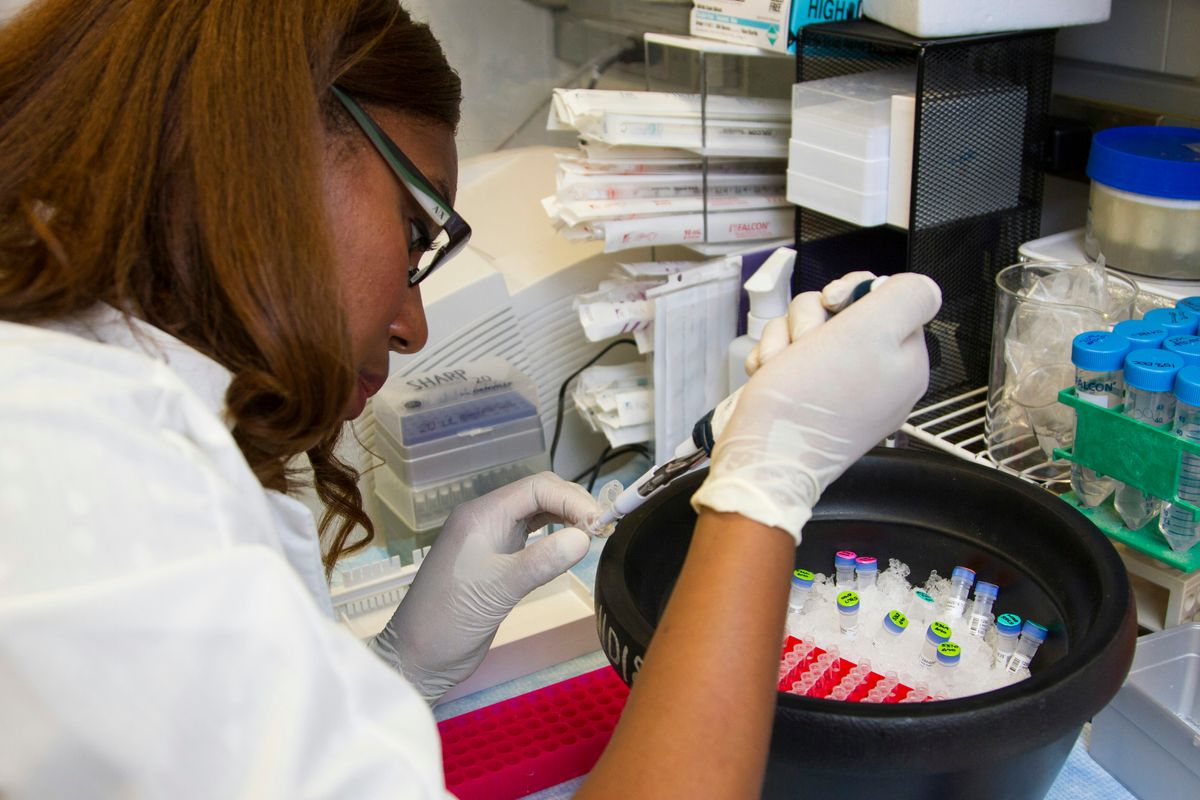
The Dilemma of Genetic Experimentation
The advancement of biotechnology brings forth a myriad of ethical concerns, particularly in the realm of genetic experimentation. Safety is a paramount issue, as the long-term effects of genetic modification are not fully understood. The potential for misuse, such as the development of bioweapons or the creation of bio-enhanced surveillance systems, adds a layer of complexity to the ethical debate.
Generative AI has emerged as a versatile tool in this field, often likened to a digital Swiss Army knife for its ability to adapt to various needs. However, its centralized control could lead to the neglect of specialized use cases that are critical for safe and ethical experimentation.
The ethical considerations surrounding genetic modification and biotechnology are vast and multifaceted, requiring careful governance and oversight to ensure responsible development.
The following points highlight key ethical concerns:
- The risk of unforeseen consequences from altering genetic material.
- The possibility of exacerbating social inequalities through access to genetic enhancements.
- The challenge of establishing robust governance to prevent unethical experimentation.
Biotech's Role in Climate Change Mitigation
The biotech industry is increasingly recognized for its potential to address environmental challenges. Biotech innovations are pivotal in developing sustainable solutions such as biofuels, bioremediation, and crop fortification. These advancements not only contribute to combating climate change but also bolster food security and healthcare.
AI contributes to addressing climate change through predictive analytics, energy optimization, and conservation efforts. Trustworthy AI development is crucial for long-term sustainability and societal benefits, including healthcare breakthroughs.
Biotech's integration with AI is transforming the sector, optimizing production through data analytics and automation. This synergy is evident in areas like AI-enabled drug discovery and DNA data storage, which also have significant implications for energy and environmental sectors. The table below highlights the multifaceted impact of biotech on climate change mitigation:
Sector | Contribution |
---|---|
Energy | Development of biofuels |
Agriculture | Crop fortification |
Healthcare | CRISPR-enabled treatments |
As we harness the power of biotech, it is imperative to ensure that the development of these technologies is responsible and aligned with ethical standards, to maximize their positive impact on society and the environment.
Surveillance and Privacy in the Age of Bio-enhanced Tech
The integration of biotechnology with surveillance capabilities presents a complex landscape of privacy concerns. Biotech companies amass mountains of sensitive information: genetic sequences, medical records, and even facial and behavioral data gleaned from wearables. This accumulation of data raises critical questions about consent, ownership, and the potential for misuse.
Artificial intelligence (AI) plays a pivotal role in processing and analyzing this vast amount of data. However, the power of AI to unlock insights from biometric and health-related information also opens the door to sophisticated surveillance systems. These systems could surpass traditional privacy boundaries, leading to a bio-enhanced panopticon that monitors individuals at an unprecedented scale.
The ethical implications of such extensive data collection and surveillance are profound. Safeguarding individual privacy rights while harnessing the benefits of biotech innovations is a delicate balance that society must navigate.
The following points highlight key considerations in this domain:
- Establishing clear regulations on data usage and privacy.
- Ensuring transparency in data collection and processing.
- Developing robust cybersecurity measures to protect sensitive information.
- Promoting ethical standards and accountability among biotech entities.
Conclusion
The integration of artificial intelligence (AI) into the biotechnology sector marks a pivotal shift in the way we approach healthcare, agriculture, and environmental challenges. AI's role in drug discovery, DNA data storage, and molecular communication is not only accelerating innovation but also reshaping the lifecycle of medical treatments from the lab to commercialization. However, as we embrace these advancements, it is crucial to remain vigilant about the ethical implications and potential misuse of biotechnologies. The future of biotech is undeniably intertwined with AI, and its judicious application promises to unlock unprecedented possibilities for improving human life and the world we inhabit, provided we navigate its complexities with responsibility and foresight.
Frequently Asked Questions
How is AI revolutionizing drug discovery in biotech?
AI is revolutionizing drug discovery by enabling the rapid analysis and synthesis of chemical compounds, predicting their interactions, and optimizing drug design through machine learning algorithms. This accelerates the development process and reduces the need for manual labor in labs.
What are the emerging trends in biotechnology?
Emerging trends in biotechnology include the integration of Artificial Intelligence (AI), Big Data analytics, biomanufacturing, precision medicine, and tissue engineering, all aimed at enhancing innovation and efficiency in the biotech sector.
How is AI used in precision medicine and diagnostics?
AI is used in precision medicine and diagnostics through computer vision for detecting cancers in medical scans and generative AI models that assist in designing personalized treatment plans by analyzing patient-specific data.
What role does AI play in biotech commercialization?
AI plays a critical role in biotech commercialization by aiding in the development of molecular structures targeted for specific markets, predicting market demand for new chemicals or medicines, and streamlining the entire lifecycle of a product from research to market.
What are the ethical and environmental implications of biotech innovations?
Biotech innovations raise ethical questions regarding genetic experimentation and privacy, especially with bio-enhanced surveillance technologies. Environmentally, biotech can contribute to climate change mitigation through bioremediation and sustainable biofuel production.
How do synthetic datasets support data-driven biotech?
Synthetic datasets, created by generative AI, allow for secure data-sharing among healthcare organizations by providing statistically reliable and anonymized data. This enables large-scale pooling of information, which can lead to more significant discoveries and collaborations in biotech.