Energy Efficiency Redefined: AI Solutions for the Industry
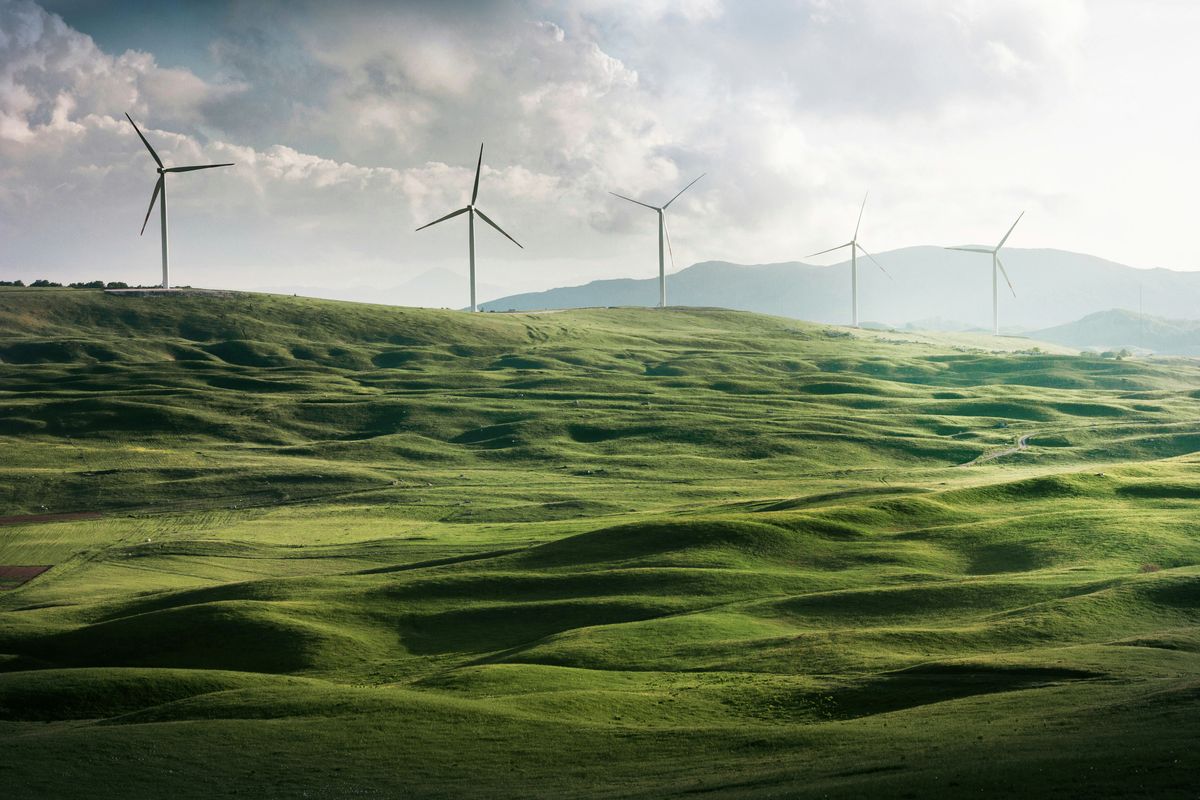
In the rapidly evolving landscape of the energy industry, the integration of AI solutions is redefining energy efficiency. From enhancing predictive maintenance to optimizing energy consumption and improving resource allocation, AI is playing a crucial role in transforming the sector. However, there are challenges in implementing these solutions, including data security concerns, integration with existing systems, and a skill gap in the workforce. Despite these challenges, measuring the impact of AI on energy efficiency is essential, with a focus on quantifying energy savings, assessing environmental benefits, and evaluating return on investment.
Key Takeaways
- AI solutions play a vital role in enhancing predictive maintenance in the energy industry.
- Optimizing energy consumption through AI leads to significant cost savings and environmental benefits.
- Efficient resource allocation is achieved through AI solutions, improving overall operational efficiency.
- Challenges in implementing AI solutions include data security concerns, integration issues, and the need to bridge the skill gap in the workforce.
- Measuring the impact of AI on energy efficiency requires quantifying energy savings, assessing environmental benefits, and evaluating return on investment.
The Role of AI in Energy Efficiency
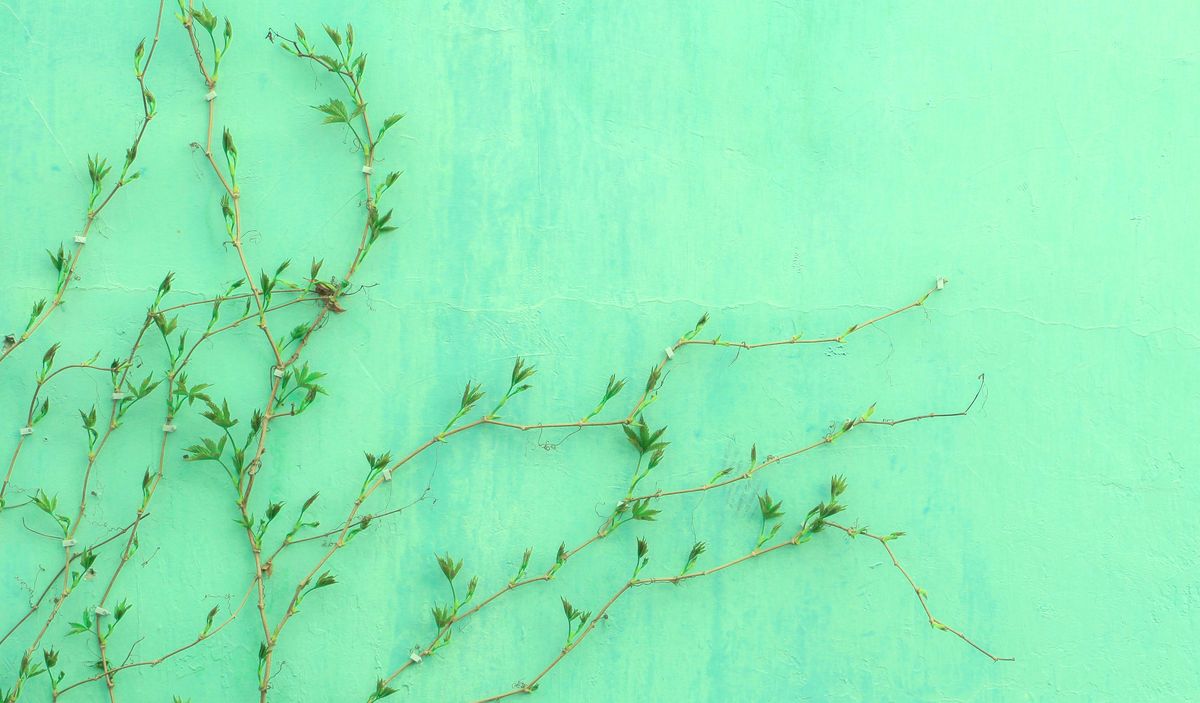
Enhancing Predictive Maintenance
Predictive maintenance is a game-changer in the realm of energy efficiency, leveraging the power of artificial intelligence to anticipate equipment failures before they occur. By predicting maintenance needs, AI enables proactive repairs, minimizing downtime and conserving energy that would otherwise be lost in unexpected breakdowns.
- Early detection of potential issues
- Scheduling of maintenance activities
- Reduction in unplanned outages
Predictive maintenance not only extends the lifespan of machinery but also ensures that equipment operates at peak efficiency. Integration with building management systems enhances overall efficiency, making AI-driven predictive maintenance a cornerstone of modern energy-saving strategies.
The implementation of AI in predictive maintenance can be seen across various industries, from manufacturing to energy production, where the precision and accuracy of AI algorithms significantly cut energy waste.
Optimizing Energy Consumption
Artificial Intelligence (AI) is revolutionizing the way industries manage and optimize energy consumption. By leveraging AI algorithms, companies can achieve more efficient energy distribution, ensuring that energy is used where it's needed most and when it's most cost-effective. AI-driven systems can predict peak demand times and adjust energy usage accordingly, leading to significant cost savings and reduced environmental impact.
Energy optimization is not just about reducing consumption but also about smart distribution. AI algorithms can analyze energy demands and supply trends to optimize the distribution of energy across buildings and industrial facilities. This results in a more balanced load on the power grid and prevents wasteful energy expenditure.
By implementing AI solutions for energy optimization, industries can expect a more sustainable operation, with the dual benefits of cost reduction and environmental responsibility.
The impact of AI on energy efficiency can be measured in various ways, including the reduction in total energy usage and the smoothing of peak demand curves. These metrics are crucial for understanding the effectiveness of AI in energy management.
Improving Resource Allocation
The integration of AI into resource allocation processes is a game-changer for energy management. By leveraging machine learning algorithms, AI systems can analyze vast amounts of data to optimize the distribution of energy resources. This leads to a more efficient use of power, minimizing waste and maximizing output.
AI-powered resource allocation not only streamlines operations but also contributes to significant cost savings. In industries such as manufacturing and data centers, where energy demand is high, the impact of AI can be particularly profound. For example, in data centers, AI can adjust cooling systems in real-time, based on the current load, to ensure optimal performance without unnecessary energy expenditure.
The strategic application of AI in resource allocation can lead to a more sustainable and cost-effective energy landscape.
The benefits of AI-driven resource allocation extend beyond immediate financial savings. It also plays a crucial role in promoting sustainability by reducing the carbon footprint of industrial operations. As companies strive to meet environmental targets, AI becomes an indispensable tool in their arsenal.
Challenges in Implementing AI Solutions
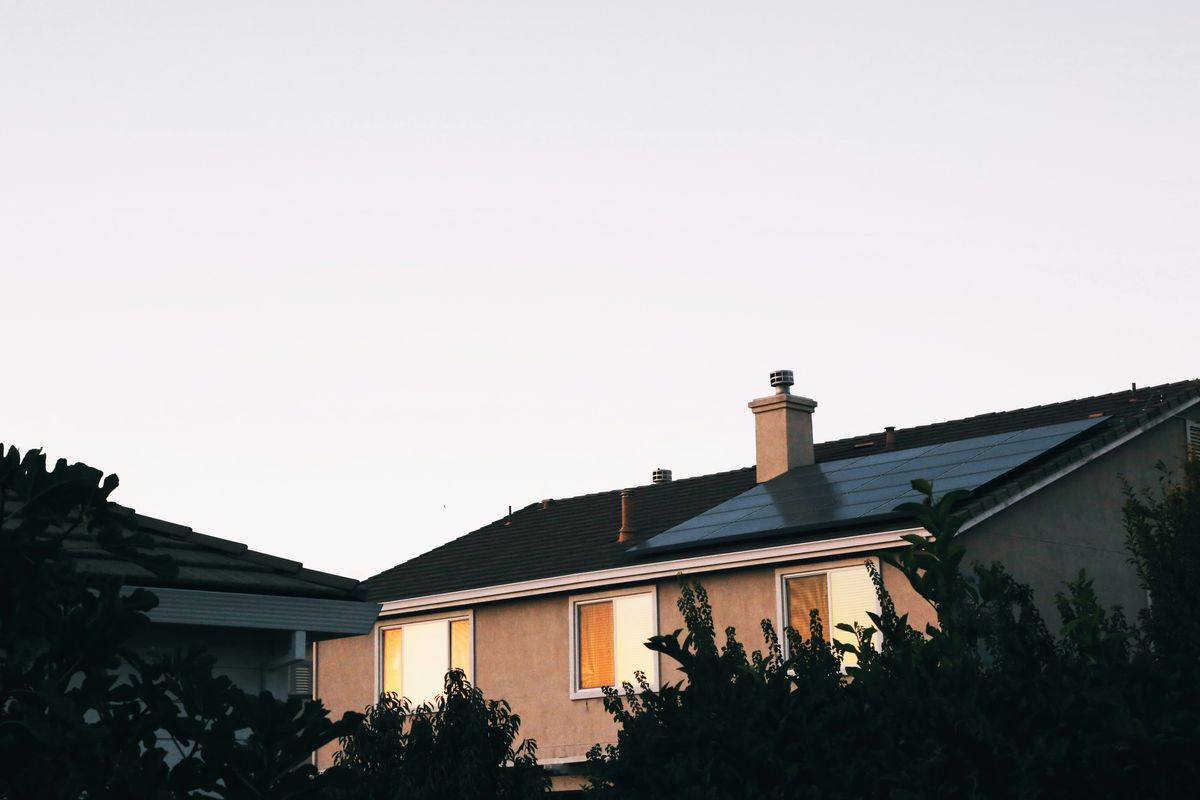
Data Security Concerns
Integrating AI solutions into existing industrial systems presents a unique set of challenges. Compatibility between new AI tools and legacy equipment is often a hurdle, as older systems may not be designed to communicate with modern AI applications. This can lead to a need for significant upgrades or even complete overhauls of current infrastructure.
- Assessment of current systems' capabilities
- Identification of necessary upgrades
- Development of integration plans
- Execution of a phased implementation strategy
Ensuring a smooth integration requires careful planning and a strategic approach to avoid disruptions in operations. It is crucial to establish a clear roadmap that outlines the steps for successful AI adoption.
Moreover, the integration process must be managed with an eye on maintaining data integrity and operational continuity. The transition to AI-enhanced systems should be seamless, minimizing downtime and ensuring that the industrial processes remain efficient and secure.
Integration with Existing Systems
Integrating AI solutions into existing energy systems poses a significant challenge for many organizations. Compatibility issues can arise, as legacy systems may not be designed to communicate effectively with modern AI technologies. To address this, businesses must often undertake a comprehensive system overhaul or develop customized interfaces, which can be both time-consuming and costly.
Interoperability is a key factor in the successful integration of AI. It ensures that new AI tools can work seamlessly with current infrastructure, enabling a smoother transition and more immediate benefits in terms of energy efficiency. The following list outlines the typical steps involved in integrating AI with existing systems:
- Assessment of current infrastructure
- Identification of compatibility gaps
- Development of integration plans
- Customization or upgrading of systems
- Testing and validation of AI integration
The goal is to create a symbiotic relationship between AI and existing systems, where each enhances the other's capabilities, leading to greater overall efficiency.
For those seeking guidance on this complex process, resources such as the Appinventiv's "AI in Business: A Comprehensive Integration Guide" can be invaluable. This guide provides insights on optimizing operations and achieving success through strategic AI implementation.
Skill Gap in Workforce
The integration of AI into energy efficiency initiatives is not without its challenges, particularly when it comes to the skill gap in the workforce. Adopting AI technologies requires a certain level of expertise that is not always readily available within existing teams. This gap can slow down implementation and reduce the effectiveness of AI solutions.
To address this issue, companies may need to consider several strategies:
- Investing in training and development programs for current employees
- Recruiting new talent with the necessary AI skills
- Partnering with educational institutions to prepare future workers
It is crucial for organizations to proactively tackle the skill gap to fully harness the potential of AI in enhancing energy efficiency.
The AI revolution reshapes economies and creates new markets, but it also demands a workforce that is adept at navigating the complexities of advanced technologies. Without the right skills, the benefits of AI, such as advancements in predictive maintenance, may not be fully realized.
Measuring the Impact of AI on Energy Efficiency
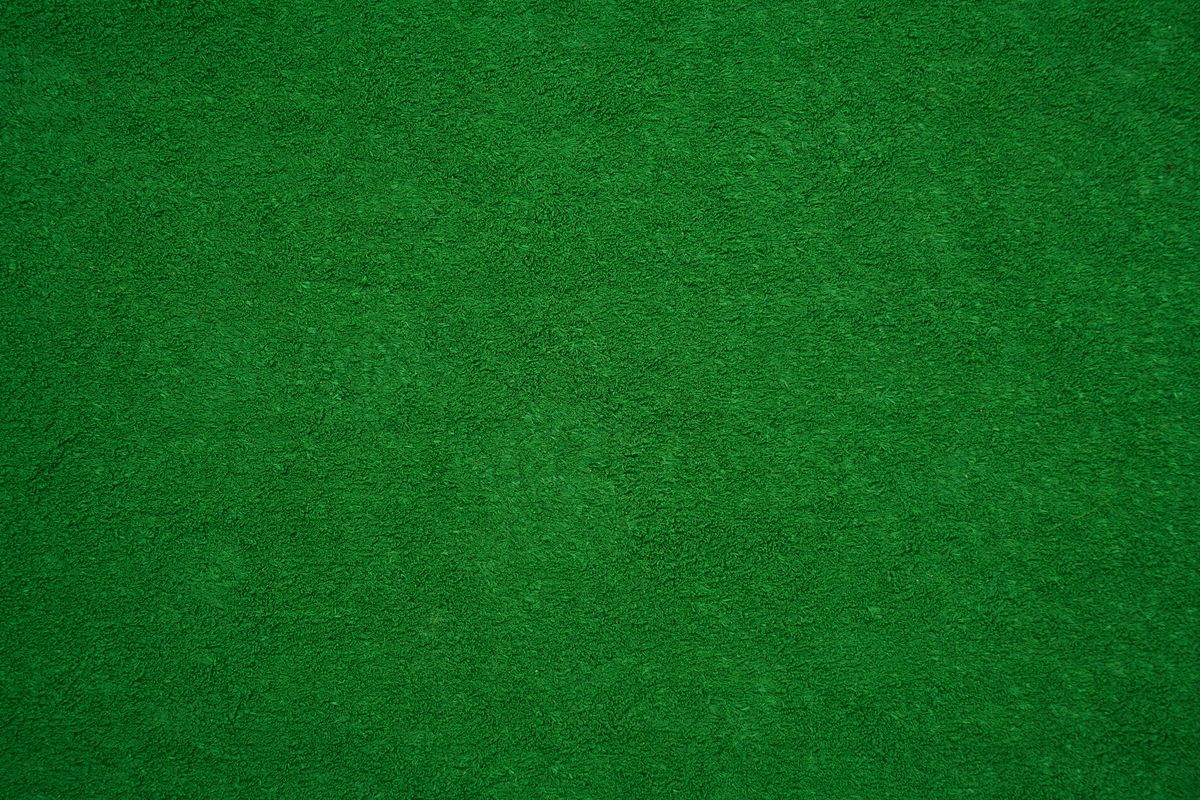
Quantifying Energy Savings
The adoption of AI in energy management systems allows for precise tracking and analysis of energy usage patterns. By leveraging data analytics, AI can identify inefficiencies and suggest actionable insights to reduce energy consumption. Accurate quantification of energy savings is crucial for validating the effectiveness of AI interventions.
Energy savings can be measured in various units such as kilowatt-hours (kWh), British Thermal Units (BTU), or in financial terms, reflecting the cost savings. A common approach is to compare pre- and post-implementation energy usage to determine the reduction in consumption. This comparison can be structured as follows:
Period | Energy Usage (kWh) | Cost Savings ($) |
---|---|---|
Pre-AI | 10,000 | 1,200 |
Post-AI | 7,500 | 1,800 |
By focusing on the energy savings achieved, businesses can not only reduce operational costs but also contribute to environmental sustainability. The data-driven nature of AI ensures that these savings are not just one-off benefits but part of a continuous improvement process.
It is essential to consider the broader implications of energy savings, such as the reduction in carbon footprint and the positive impact on corporate social responsibility. These factors, while not easily quantifiable, play a significant role in the long-term strategy of a business.
Assessing Environmental Benefits
The environmental benefits of AI-driven energy efficiency are profound and multifaceted. By reducing energy consumption, AI helps in lowering greenhouse gas emissions, a critical factor in combating climate change. The use of AI enables a more sustainable use of resources, leading to less waste and a smaller carbon footprint.
Environmental impact assessments often involve complex calculations and predictive modeling. AI excels in processing large datasets to provide accurate forecasts of environmental savings. For instance, AI can predict the reduction in emissions resulting from optimized energy use in industrial processes.
The integration of AI in energy systems not only conserves energy but also preserves natural habitats by reducing the need for resource extraction.
To illustrate the environmental impact, consider the following table showing hypothetical data on emission reductions:
Year | CO2 Reduction (tons) | Energy Saved (MWh) |
---|---|---|
2021 | 10,000 | 5,000 |
2022 | 12,500 | 6,200 |
2023 | 15,000 | 7,500 |
This table demonstrates the potential year-over-year improvements in emission reductions and energy savings, highlighting the tangible benefits of AI in environmental conservation.
Evaluating Return on Investment
Evaluating the Return on Investment (ROI) is crucial for businesses to justify the adoption of AI in energy efficiency initiatives. The Key Factors Impacting ROI in Predictive Maintenance include skilled personnel, data quality, technology selection, and implementation strategy. These elements are pivotal for PdM success, balancing costs with benefits.
ROI calculations should account for both direct and indirect financial gains. Direct savings are often quantifiable, such as reduced energy costs or decreased downtime. Indirect benefits, though harder to measure, can include improved equipment lifespan and enhanced operational safety.
To systematically assess ROI, companies can use the following framework:
- Initial Investment: The upfront cost of AI technology and related infrastructure.
- Operational Costs: Ongoing expenses for maintenance, updates, and personnel training.
- Cost Savings: Reductions in energy consumption and maintenance expenses.
- Revenue Impact: Increased production efficiency and potential market advantages.
By analyzing these factors, organizations can determine the financial viability and strategic value of implementing AI for energy efficiency.
Conclusion
In conclusion, the integration of AI solutions in the industry has redefined energy efficiency by optimizing processes, reducing waste, and improving overall performance. With the power of artificial intelligence, businesses can now achieve higher levels of productivity and sustainability. As technology continues to advance, the potential for further innovations in energy efficiency is limitless. It is clear that AI is revolutionizing the way industries approach energy management, paving the way for a more sustainable future.
Frequently Asked Questions
How does AI enhance predictive maintenance in the energy industry?
AI algorithms analyze historical data to predict equipment failures before they occur, enabling proactive maintenance and minimizing downtime.
What are the benefits of optimizing energy consumption using AI solutions?
AI can optimize energy usage by adjusting consumption based on real-time data, leading to cost savings and reduced environmental impact.
How does AI improve resource allocation in the energy sector?
AI algorithms can analyze demand patterns and optimize the allocation of resources such as manpower, equipment, and materials to increase efficiency and reduce waste.
What are the main data security concerns in implementing AI solutions for energy efficiency?
Data privacy, protection against cyber threats, and secure data sharing are key concerns when implementing AI solutions in the energy sector.
How can AI solutions be integrated with existing systems in the energy industry?
AI systems can be integrated through APIs, data connectors, and middleware to ensure seamless communication and data exchange with existing systems.
What measures can be taken to address the skill gap in the workforce for implementing AI solutions in energy efficiency?
Training programs, upskilling initiatives, and partnerships with educational institutions can help bridge the skill gap and prepare the workforce for implementing AI solutions in energy efficiency.