Empowering Visual Cognition: Advances in Computer Vision Applications
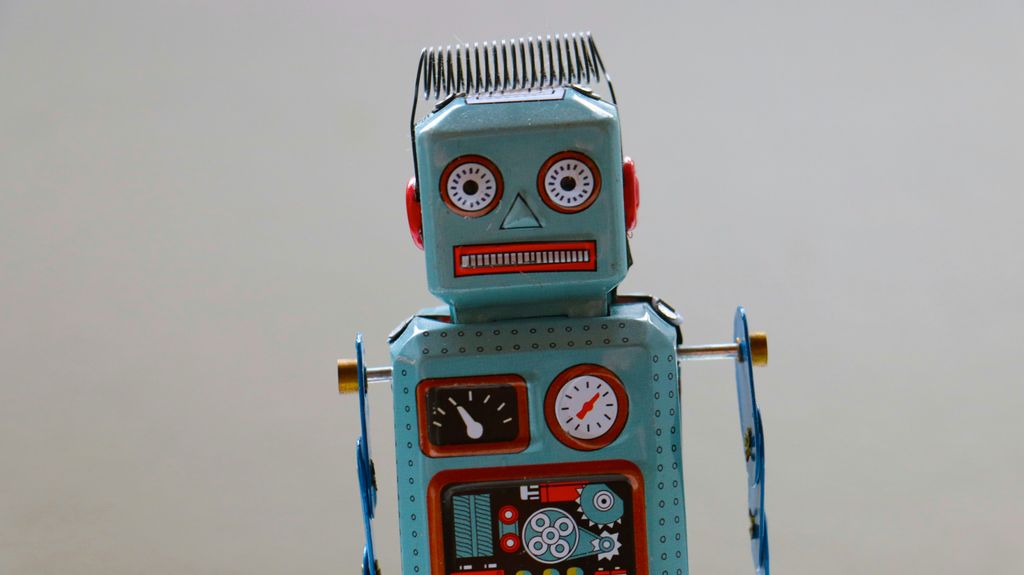
Computer vision is a branch of artificial intelligence that enables machines to interpret and understand visual information from the real world. Recent advancements in computer vision have led to improved object recognition, image segmentation, and scene understanding capabilities. This article explores the advancements, applications, and benefits of computer vision technology in various industries such as healthcare, manufacturing, and autonomous vehicles.
Key Takeaways
- Computer vision technology enables machines to interpret and understand visual information from the real world
- Recent advancements in computer vision have improved object recognition, image segmentation, and scene understanding capabilities
- Applications of computer vision include healthcare innovations, manufacturing automation, and autonomous vehicles development
- Benefits of computer vision include enhanced productivity and efficiency, innovative opportunities in AR and medical imaging, and safety and security enhancements
- Computer vision drives insights and decision-making based on visual data in diverse industries
Advancements in Computer Vision
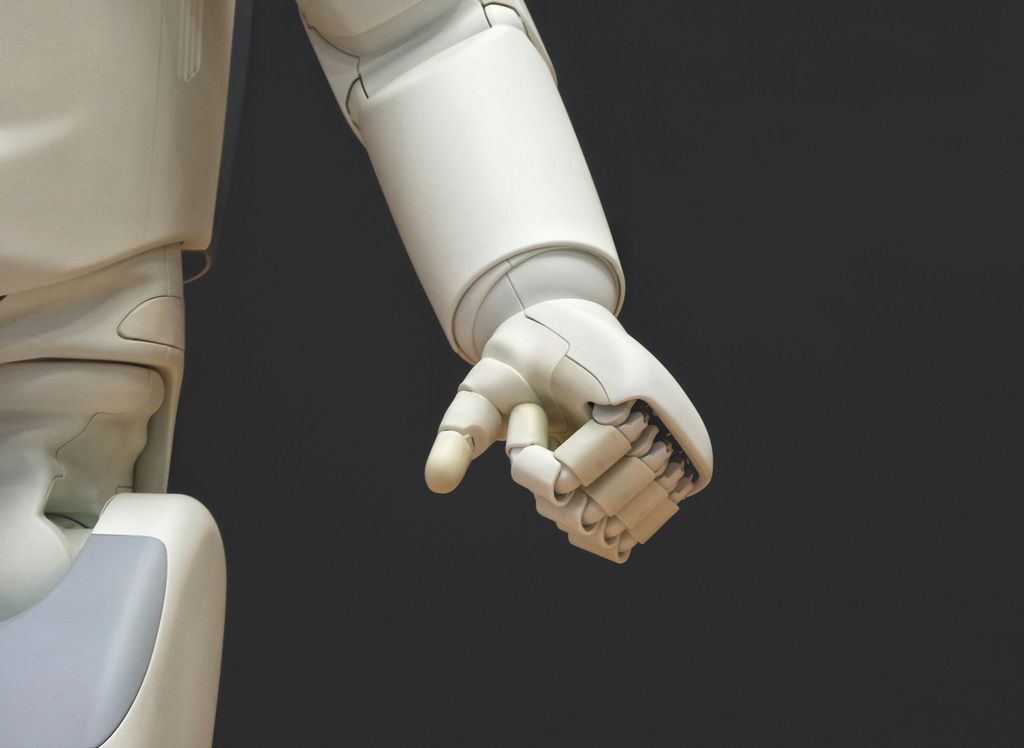
Object Recognition Enhancements
Recent advancements in computer vision have significantly improved object recognition capabilities. Object recognition techniques vary widely, from traditional methods like template matching to advanced deep learning algorithms, such as Convolutional Neural Networks (CNNs). These enhancements are pivotal for the development of more sophisticated and accurate computer vision systems.
The integration of state-of-the-art (SOTA) object detection and Optical Character Recognition (OCR) models has led to remarkable improvements in detection accuracy. This has been instrumental in refining multimodal learning language models (MLLMs) with integrated vision detection capabilities, resulting in more nuanced image understanding.
The success of object recognition models is crucial for the reliability and applicability of computer vision systems across various industries.
Further research is directed towards overcoming the limitations of closed-set detection models, which are efficient but constrained by their scope, and open-set models, which offer a broader range but can be prone to inaccuracies. The pursuit of robustness against detection errors and the exploration of architectural modifications present exciting opportunities for future enhancements.
Image Segmentation Improvements
Recent advancements in image segmentation have been pivotal in enhancing computer vision capabilities. Instance segmentation, a task that involves identifying and delineating individual objects within an image, has seen significant improvements. This process not only detects objects but also assigns a unique label to each, allowing for more granular analysis and application.
Innovations such as the Rssformer have introduced foreground saliency enhancement, which is crucial for tasks like remote sensing land-cover segmentation. By focusing on the salient parts of an image, these models achieve greater precision in distinguishing between different regions.
The diffusion process in image segmentation has revealed how models predict visual concepts at each denoising step, leading to more accurate and detailed image recovery.
Furthermore, the development of methods like Self Correspondence Distillation for weakly-supervised semantic segmentation indicates a shift towards more efficient training processes. These methods leverage the inherent structure of data to reduce the need for extensive labeled datasets, which are often costly and time-consuming to produce.
Scene Understanding Capabilities
The realm of computer vision has seen significant strides in scene understanding capabilities, enabling machines to interpret complex visual data with greater accuracy. Scene understanding goes beyond mere object recognition; it involves comprehending the context and relationships within a visual scene. This advancement is pivotal for applications where contextual awareness is crucial, such as in autonomous driving or advanced surveillance systems.
Scene understanding is facilitated by sophisticated algorithms that analyze various elements of a scene, including the layout, the interactions between objects, and the overall semantics. These algorithms leverage deep learning techniques to provide a comprehensive interpretation of the visual data.
The integration of scene understanding in computer vision systems has led to a transformative shift in how machines perceive and interact with their environment.
The following list highlights key components that contribute to enhanced scene understanding capabilities:
- Advanced object detection and recognition
- Contextual relationship modeling
- Semantic segmentation and classification
- Integration of temporal dynamics for scene evolution
- Use of attention mechanisms to focus on relevant scene elements
Applications of Computer Vision
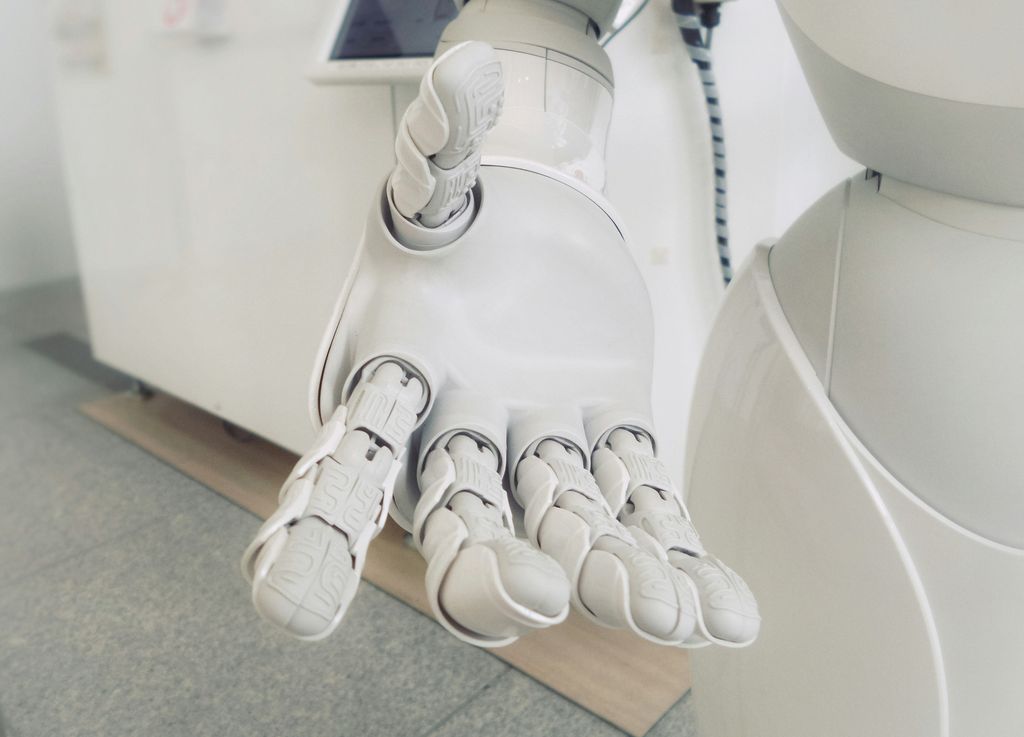
Healthcare Innovations
The integration of computer vision in healthcare is revolutionizing the way medical professionals diagnose and treat diseases. Advanced imaging techniques, powered by computer vision, are enabling earlier detection of conditions and more precise interventions. For instance, in radiology, algorithms can now identify patterns in imaging data that may be imperceptible to the human eye.
Telemedicine has also benefited greatly from computer vision advancements. Remote consultations and diagnostics are becoming more accurate and accessible, allowing for better patient care regardless of location. This is particularly important in areas with limited access to medical facilities.
The synergy between computer vision and healthcare is creating a new paradigm in patient care, where technology enhances the capabilities of medical professionals and improves patient outcomes.
Here are some key areas where computer vision is making an impact in healthcare:
- Automated analysis of medical images for faster diagnosis
- Precision surgery with image-guided robotic systems
- Monitoring patient movements and vitals through non-invasive sensors
- Enhancing prosthetics with visual feedback capabilities
The potential of computer vision in healthcare continues to grow as researchers and practitioners collaborate to develop innovative applications that push the boundaries of what's possible in medicine.
Manufacturing Automation
The integration of Artificial Intelligence (AI) into manufacturing is revolutionizing the industry, enabling unprecedented levels of automation and efficiency. AI-driven systems are now capable of performing complex tasks, from quality assurance (QA) and defect detection to preventative maintenance, leveraging sensor data to predict and mitigate potential issues before they arise.
- Quality Assurance and Defect Detection
- Preventative Maintenance
- Material Manipulation
- Supply Chain Optimization
The next generation of AI in manufacturing aims to fully integrate into the entire production process, enhancing the manipulation of materials and streamlining the supply chain.
The adoption of AI in manufacturing also addresses labor shortages by automating tasks that traditionally required human intervention. This shift not only improves productivity but also creates new job opportunities that blend manufacturing with IT skills. As AI systems become more situationally aware, they offer the flexibility to handle multiple tasks and adapt to various designs and materials, challenging the traditional notion of single-purpose machinery.
Autonomous Vehicles Development
The development of autonomous vehicles is a testament to the remarkable strides made in computer vision. These vehicles, equipped with AI, utilize a complex array of sensors and advanced algorithms to navigate and respond to dynamic road conditions. Not only do they promise to revolutionize transportation by reducing accidents and improving traffic flow, but they also aim to decrease carbon emissions significantly.
Beyond the realm of personal cars, this technology is expanding into public transportation and freight, with autonomous buses and trucks becoming increasingly prevalent. As AI continues to integrate with transportation infrastructure, we can anticipate more efficient and safer transit systems. This could potentially transform urban landscapes by minimizing the need for parking spaces and altering commuting patterns.
Despite the enthusiasm, the adoption timeline for autonomous vehicles has experienced delays. According to a report by McKinsey, the stages of autonomous-vehicle development indicate that the timeline has slipped by two to three years on average across all autonomy levels.
The autonomous vehicle industry is moving forward, albeit at a cautious pace, ensuring that safety and reliability remain at the forefront of this technological evolution.
Benefits of Computer Vision
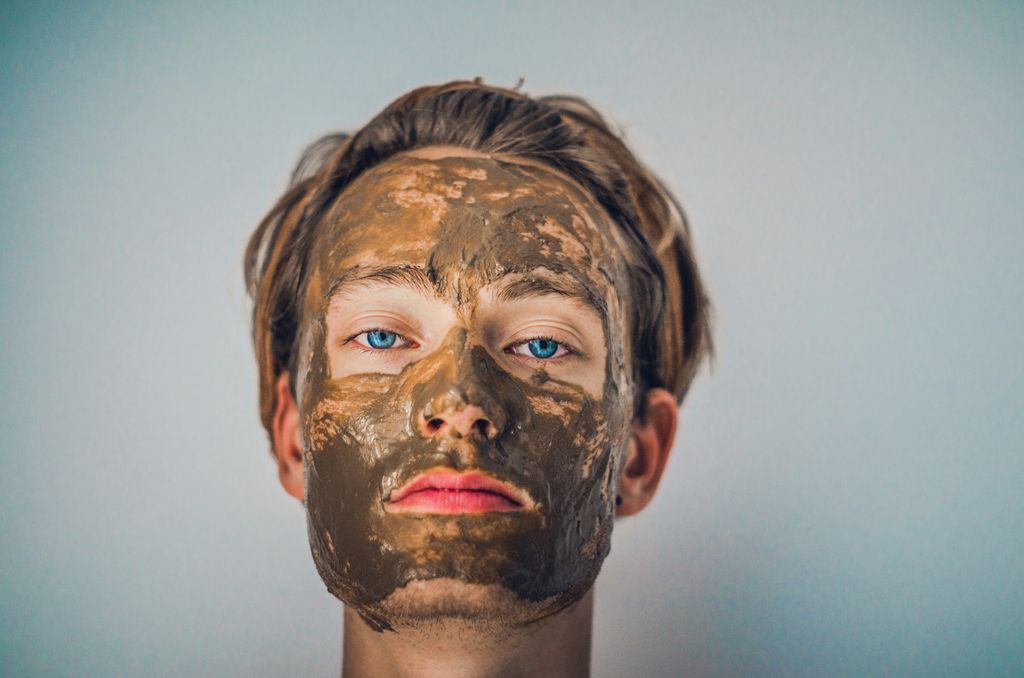
Enhanced Productivity and Efficiency
The integration of computer vision into various sectors has led to a significant boost in productivity and efficiency. By automating routine and repetitive tasks, computer vision allows employees to focus on more complex and strategic work. This shift not only optimizes the workflow but also enhances the quality of outputs.
The synergy between computer vision and automation is transforming industries by enabling smarter and faster decision-making processes. It facilitates tighter monitoring and control, leading to a more efficient allocation of resources.
In the realm of robotics, for example, computer vision has been instrumental in improving task automation and data processing. The table below illustrates some of the key productivity enhancements attributed to computer vision in robotics:
Application Area | Productivity Enhancement |
---|---|
Quality Control | Increased accuracy |
Data Processing | Faster analysis |
Task Automation | Reduced manual intervention |
As we look to the future, the role of computer vision in driving productivity will only become more pronounced, with advances in AI further expanding its capabilities and applications.
Innovative Opportunities in AR and Medical Imaging
The intersection of computer vision with augmented reality (AR) and medical imaging is a hotbed for innovation. Advances in AR are transforming the medical field, allowing for more precise and interactive visualizations of complex anatomical structures. This not only enhances the training of medical professionals but also improves surgical planning and patient education.
In the realm of medical imaging, computer vision algorithms are being fine-tuned to provide more accurate diagnoses and prognoses. For example, machine learning techniques are improving the detection of anomalies in imaging data, which is crucial for early intervention in diseases.
The synergy between computer vision and AR is paving the way for groundbreaking applications that could revolutionize patient care and medical research.
According to GlobalData, the leading innovators in AR-based medical imaging are setting the pace for the medical devices industry. These advancements are not just technical marvels; they represent a leap forward in patient outcomes and healthcare delivery.
Safety and Security Enhancements
The integration of computer vision into safety and security systems has led to significant advancements in threat detection and response. Real-time insights provided by AI-driven solutions, such as SparkCognition's Visual AI Advisor, are transforming the way public sector agencies and educational institutions approach security. By leveraging existing security cameras, these systems can proactively counter threats, ensuring a higher level of safety.
Visual AI Advisor detects a variety of threats, from the presence of weapons to intrusion and trip hazards, triggering immediate alerts and safety procedures. This technology has been implemented in over 147,000 cameras across 16 countries, demonstrating its global impact and reliability. The following list highlights some of the top applications of computer vision in security:
- Health and safety monitoring
- Zero-incident driving initiatives
- Guarding peaceful community living
The ability to seamlessly integrate AI efficiencies into existing security infrastructures reinforces public safety and situational awareness, making communities safer for everyone.
Conclusion
In conclusion, the advancements in computer vision technology have revolutionized the way machines interpret and understand visual information from the real world. This technology has diverse applications in fields such as healthcare, manufacturing, and autonomous vehicles, leading to improved object recognition, image segmentation, and scene understanding capabilities. The benefits of computer vision are vast, including enhancing productivity, enabling innovation in augmented reality and medical imaging, improving safety and security through surveillance systems, and facilitating accessibility for individuals with visual impairments. As we continue to witness the growth of artificial intelligence and machine learning, the role of computer vision will become increasingly crucial in driving insights and decision-making based on visual data across various industries.
Frequently Asked Questions
What is computer vision and how does it work?
Computer vision is a branch of AI that enables machines to interpret and understand visual information from the real world. It works by processing visual data through algorithms to recognize patterns and make decisions based on the input.
What are some common applications of computer vision technology?
Some common applications of computer vision include healthcare innovations, manufacturing automation, and development of autonomous vehicles.
How does computer vision enhance productivity and efficiency?
Computer vision enhances productivity and efficiency by automating visual inspection and analysis tasks, reducing human error, and speeding up processes.
In what ways can computer vision contribute to safety and security enhancements?
Computer vision contributes to safety and security enhancements through surveillance systems, threat detection, and facilitating accessibility for individuals with visual impairments.
What are the benefits of using computer vision in augmented reality and medical imaging?
Using computer vision in augmented reality and medical imaging enables new opportunities for innovation, improves accuracy in diagnostics, and enhances the overall user experience.
How is reinforcement learning related to computer vision technology?
Reinforcement learning is a machine learning approach that involves training AI agents to make sequential decisions in environments. It can be applied in conjunction with computer vision for tasks requiring decision-making based on visual data.