AI in Manufacturing
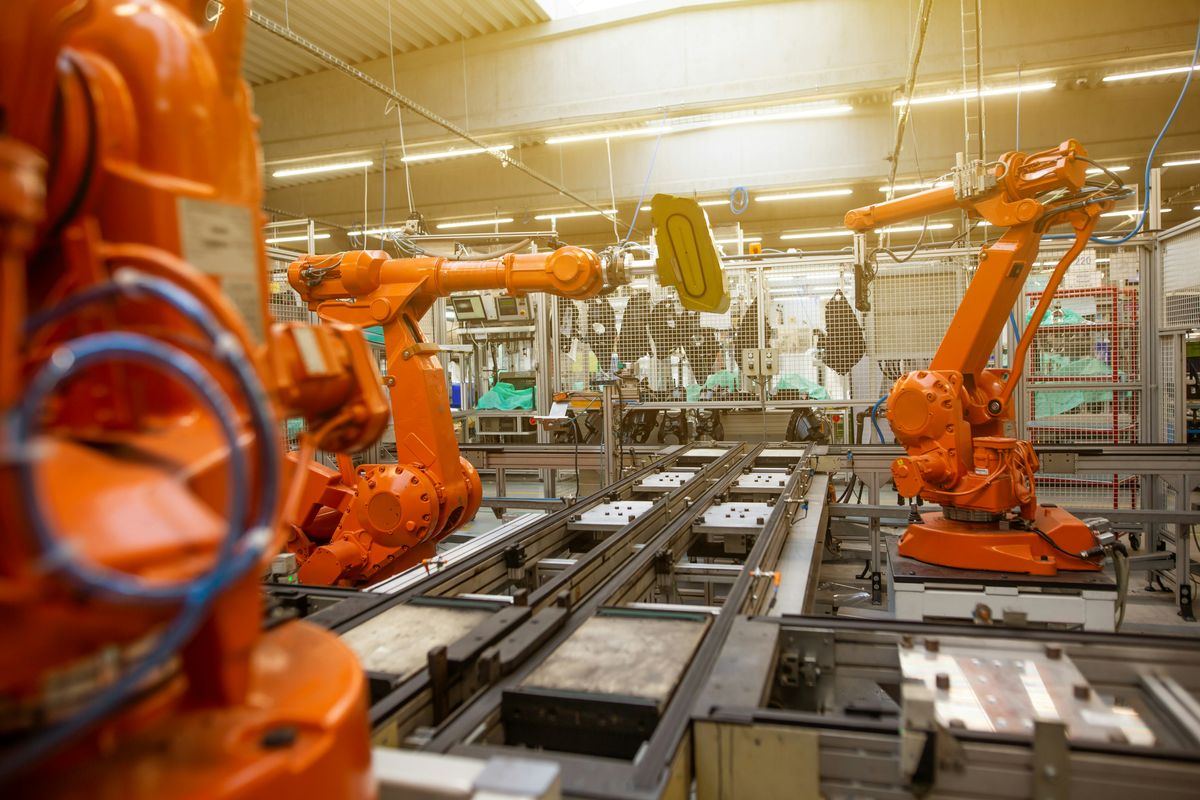
Artificial Intelligence (AI) is revolutionizing the manufacturing industry by offering numerous benefits and applications. However, implementing AI in manufacturing also comes with its own set of challenges. This article explores the benefits, challenges, and applications of AI in the manufacturing sector.
Key Takeaways
- AI enhances efficiency and productivity in manufacturing processes.
- Quality improvement is achieved through AI-driven insights and automation.
- Predictive maintenance helps prevent costly downtime and equipment failures.
- Data integration is a key challenge in implementing AI in manufacturing.
- Workforce training is essential to harness the full potential of AI in manufacturing.
Benefits of AI in Manufacturing
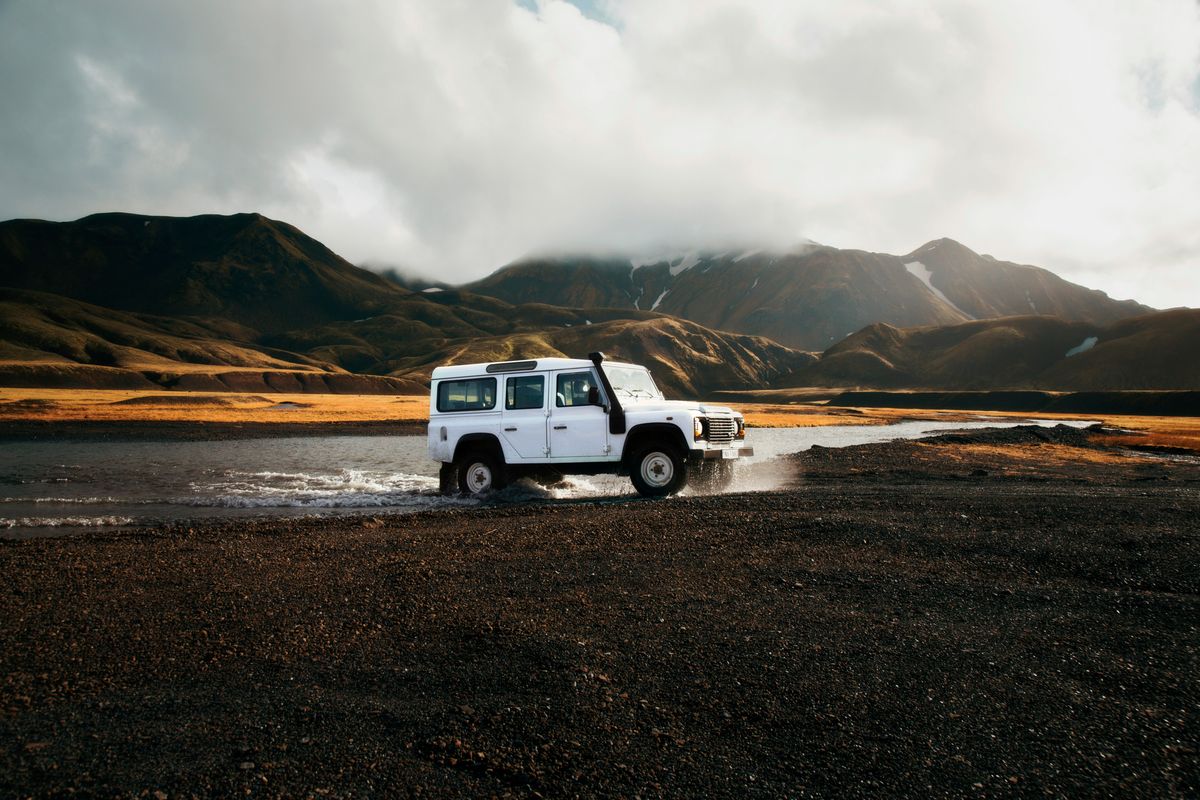
Increased Efficiency
The integration of artificial intelligence (AI) in manufacturing has led to significant strides in operational efficiency. AI-driven systems are adept at streamlining complex processes, reducing downtime, and enhancing productivity. For instance, AI can optimize machine use, leading to faster production times and lower costs.
- AI algorithms can analyze production workflows to identify bottlenecks.
- Real-time data processing allows for immediate adjustments to the production line.
- Energy consumption is minimized by optimizing equipment schedules.
By leveraging AI, manufacturers are able to achieve a more efficient use of resources, which is crucial in today's competitive market.
Moreover, AI contributes to sustainability efforts by monitoring and managing energy consumption. This not only reduces operational expenses but also supports environmental initiatives. The use of AI to analyze energy usage patterns and suggest optimizations is a testament to its role in promoting efficiency and sustainability.
Quality Improvement
The integration of AI in manufacturing has led to significant advancements in quality control. Smart image recognition systems have been pivotal in enhancing product quality, ensuring that defects are identified and rectified promptly. This not only improves the final product but also reduces waste and increases customer satisfaction.
Quality enhancement through AI is not just about defect detection; it's about building a culture of continuous improvement. By analyzing vast amounts of data, AI algorithms can suggest modifications to the manufacturing process that lead to better outcomes. For instance, AI can help in fine-tuning machine parameters for optimal performance, resulting in products that consistently meet high standards.
The use of AI-driven quality control systems can lead to a productivity increase of up to 50%. This is a testament to the transformative power of AI in the manufacturing sector.
The table below illustrates the impact of AI on quality improvement in a manufacturing setting:
Aspect | Before AI | After AI Implementation |
---|---|---|
Defect Rate | High | Significantly Reduced |
Inspection Time | Lengthy | Greatly Reduced |
Process Adjustments | Manual | Automated |
Customer Returns | Frequent | Drastically Lowered |
Predictive Maintenance
Predictive maintenance harnesses the power of AI to forecast equipment failures before they occur, significantly reducing downtime and maintenance costs. By analyzing data from sensors and machinery, AI algorithms can detect patterns and anomalies that may indicate a potential breakdown.
The implementation of predictive maintenance can lead to substantial cost savings and increased machine longevity. It's not just about preventing failure; it's about optimizing the maintenance schedule to ensure that machines are serviced only when necessary, avoiding both over-maintenance and under-maintenance.
Predictive analytics play a crucial role in this process, enabling manufacturers to make informed decisions based on data-driven insights. Here's how AI-driven predictive maintenance can benefit a manufacturing setup:
- Early Fault Detection: Identifying issues before they escalate can prevent costly repairs and production halts.
- Maintenance Scheduling: AI can predict the optimal time for maintenance, ensuring machines are serviced without interrupting production.
- Resource Optimization: By predicting maintenance needs, manufacturers can better allocate resources and plan for parts inventory.
With predictive maintenance, manufacturers are empowered to transition from a reactive to a proactive maintenance strategy, enhancing overall operational efficiency.
Challenges of Implementing AI in Manufacturing
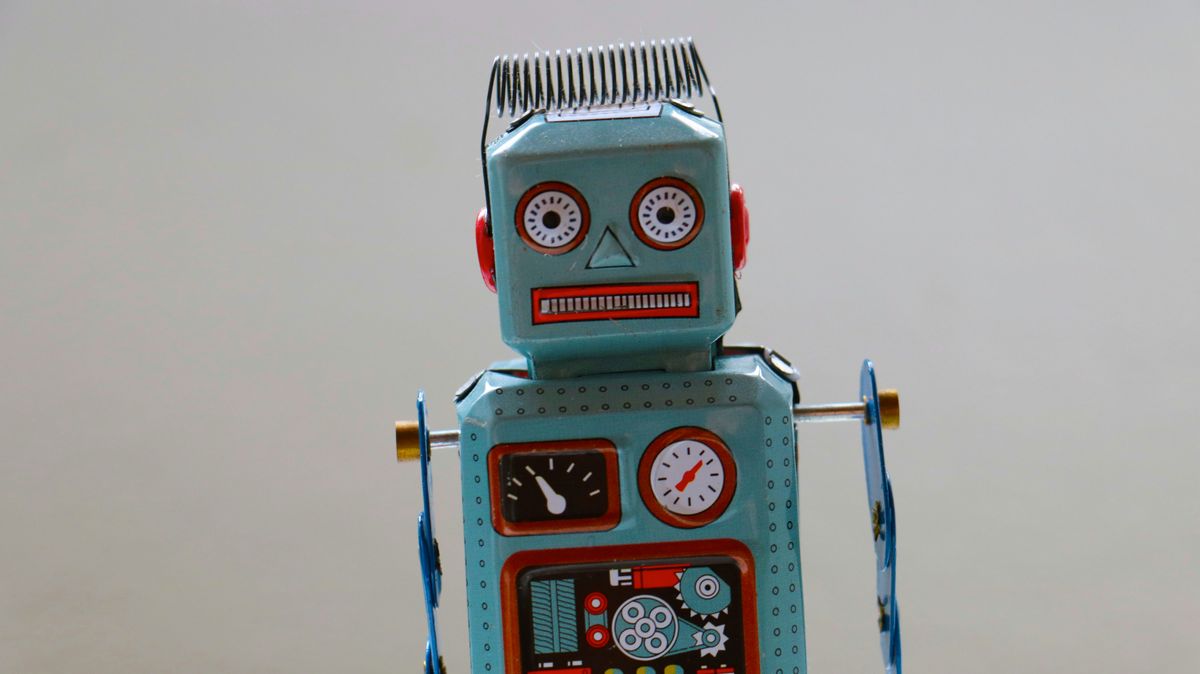
Data Integration
The integration of data is a pivotal challenge when implementing AI in manufacturing. Seamless data integration is crucial for AI systems to function effectively, as they rely on large volumes of data from various sources. Manufacturers often grapple with the heterogeneity of data formats and the need for real-time data processing.
- Ensuring compatibility between different data systems
- Establishing a centralized data repository
- Developing standardized data formats
- Implementing real-time data capture and analysis
Effective data integration facilitates a unified view of operations, which is essential for informed decision-making and strategic planning. Without it, the potential of AI to revolutionize manufacturing processes cannot be fully realized.
Manufacturers must prioritize data integration to harness the full capabilities of AI and maintain a competitive edge in the industry. It is not just about collecting data, but also about transforming it into actionable insights that drive innovation and efficiency.
Workforce Training
The integration of AI into manufacturing processes necessitates a skilled workforce capable of operating and maintaining these advanced systems. Workforce training is essential to bridge the gap between traditional manufacturing skills and the demands of modern AI-driven environments. Training programs must evolve to include not only the technical aspects of AI but also the ability to interact with and manage these intelligent systems.
Workforce training programs can be categorized into several key areas:
- Understanding AI and machine learning basics
- Operating AI-powered machinery
- Troubleshooting and maintenance of AI systems
- Safety protocols in an AI-enhanced workplace
Embracing AI in manufacturing requires a commitment to continuous learning and adaptation. Employees must be equipped with the knowledge and tools to work alongside AI, ensuring a seamless integration into the manufacturing ecosystem.
The challenge lies not only in developing effective training modules but also in delivering them in a manner that is accessible and engaging for the workforce. Companies like nFlux are leading the way with innovative on-the-job training technology, such as their Advanced Co-pilot, designed to unlock the potential of the workforce and enhance the efficiency of training programs.
Security Concerns
While AI can revolutionize manufacturing, it also introduces new security risks that must be meticulously managed. Limited testing of AI models can lead to unexpected behaviors once deployed in production environments. This unpredictability not only affects user experience but also opens up the system to potential vulnerabilities.
Cybersecurity measures are essential to safeguard sensitive data and intellectual property. Manufacturers must establish robust protocols to detect and respond to threats promptly. A proactive approach to security can prevent disruptions that may arise from cyber attacks.
It is crucial for manufacturers to stay informed about the latest security risks and to continuously update their defense mechanisms.
To effectively address these concerns, a comprehensive security strategy should include:
- Regular security audits and updates
- Employee training on security best practices
- Implementation of access controls and encryption
- Collaboration with security experts to stay ahead of emerging threats
Applications of AI in Manufacturing
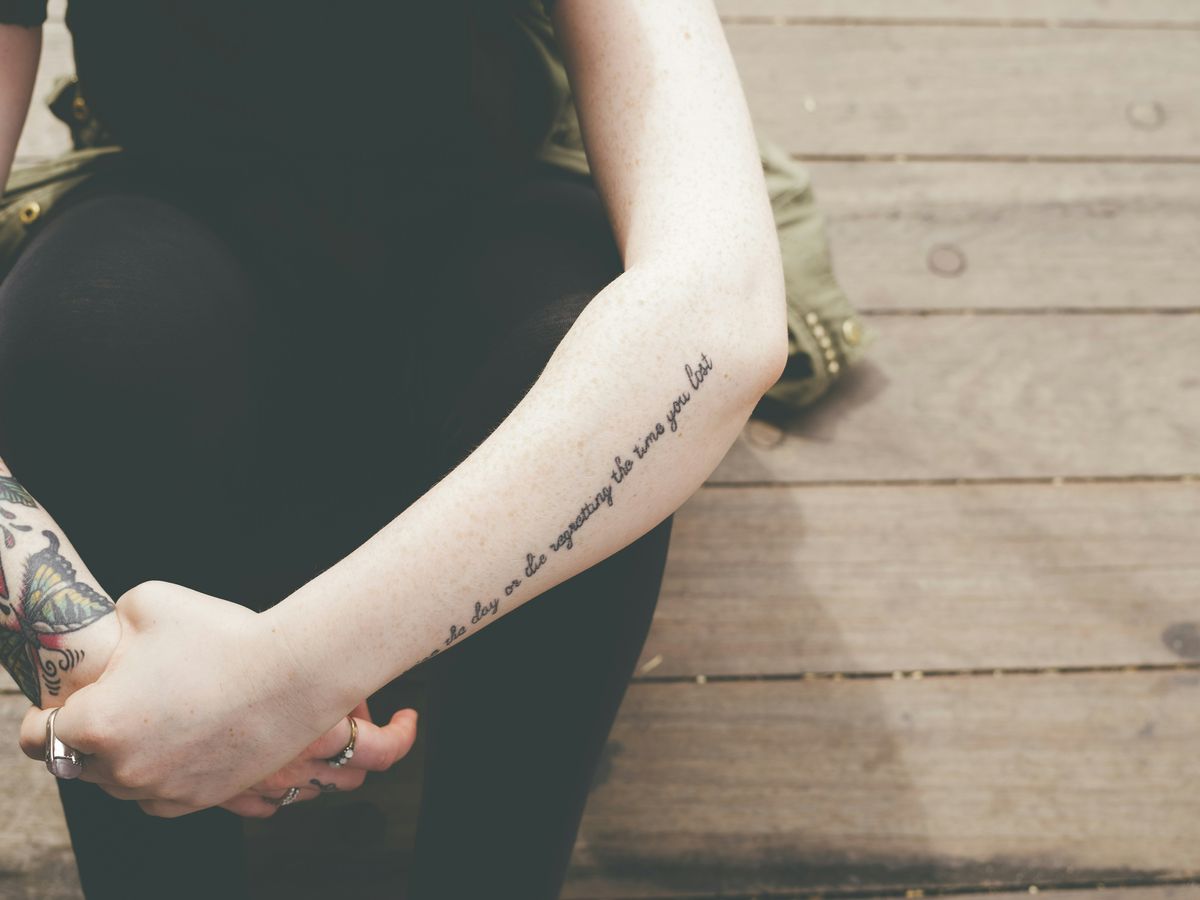
Robotic Process Automation
The advent of Robotic Process Automation (RPA) has revolutionized the way manufacturers handle routine tasks. By deploying RPA, companies can automate processes that were once time-consuming and prone to human error. This includes tasks such as inventory management, quality control, and assembly line operations.
RPA systems are designed to mimic human actions within digital systems, executing a sequence of steps to complete a process. They can work 24/7 without breaks, ensuring a consistent output that is not affected by human fatigue or variability.
The integration of RPA in manufacturing leads to significant cost savings and operational efficiencies.
Here are some of the key benefits of RPA in manufacturing:
- Reduction in operational costs
- Increased production speed
- Enhanced accuracy and consistency
- Improved employee satisfaction by removing repetitive tasks
While the benefits are clear, it's important to approach RPA with a strategic plan to ensure seamless integration with existing systems and processes.
Quality Control Systems
The integration of AI into quality control systems has revolutionized the way manufacturers detect and address defects. AI-driven quality control systems are capable of analyzing vast amounts of data from the manufacturing process, identifying anomalies that may indicate a defect. This allows for real-time quality assurance and a significant reduction in the number of defective products reaching customers.
Machine vision, combined with AI algorithms, is a key component in modern quality control systems. It enables the detection of even the smallest imperfections that might be invisible to the human eye. By implementing these advanced technologies, manufacturers can ensure a higher standard of product quality and consistency.
- Real-time defect detection
- Consistent quality standards
- Reduced waste and returns
The use of AI in quality control not only enhances product quality but also contributes to overall operational efficiency. By catching defects early, manufacturers can avoid costly recalls and maintain customer trust.
In conclusion, AI has become an indispensable tool in the realm of quality control within manufacturing. It provides a level of precision and efficiency that was previously unattainable, setting a new benchmark for quality assurance in the industry.
Supply Chain Optimization
The integration of AI into supply chain management has revolutionized the way manufacturers predict demand, manage inventory, and streamline logistics. AI-driven analytics provide deep insights into consumer behavior, enabling companies to optimize their supply chains for maximum efficiency and cost savings. For instance, AI can forecast product demand with high accuracy, reducing overproduction and waste.
Supply chain optimization involves several key components:
- Demand forecasting
- Inventory management
- Transportation logistics
- Supplier relationship management
By leveraging AI, manufacturers can dynamically adjust their supply chains in response to market changes, ensuring that the right products are available at the right time and place.
The implementation of AI in supply chains also poses challenges, such as the need for high-quality data and the integration of various systems and technologies. However, the benefits often outweigh the difficulties, leading to more resilient and responsive manufacturing operations.
Conclusion
In conclusion, the integration of AI in manufacturing has revolutionized the industry by enhancing efficiency, productivity, and quality. From predictive maintenance to autonomous robots, AI technologies have paved the way for a new era of smart manufacturing. As manufacturers continue to adopt and leverage AI solutions, we can expect to see further advancements and innovations in the field. The future of manufacturing is undoubtedly intertwined with AI, and embracing these technologies is essential for staying competitive in the global market.
Frequently Asked Questions
What are the main benefits of AI in manufacturing?
The main benefits of AI in manufacturing include increased efficiency, quality improvement, and predictive maintenance.
What are the challenges of implementing AI in manufacturing?
Challenges of implementing AI in manufacturing include data integration, workforce training, and security concerns.
How is AI used in robotic process automation in manufacturing?
AI is used in robotic process automation in manufacturing to automate repetitive tasks and improve efficiency.
What role does AI play in quality control systems in manufacturing?
AI plays a crucial role in quality control systems in manufacturing by enabling real-time monitoring and defect detection.
How does AI contribute to supply chain optimization in manufacturing?
AI contributes to supply chain optimization in manufacturing by analyzing data to improve inventory management and streamline logistics.
What measures can be taken to address security concerns related to AI implementation in manufacturing?
To address security concerns related to AI implementation in manufacturing, companies can implement robust cybersecurity protocols and regularly update AI systems.