AI in Healthcare
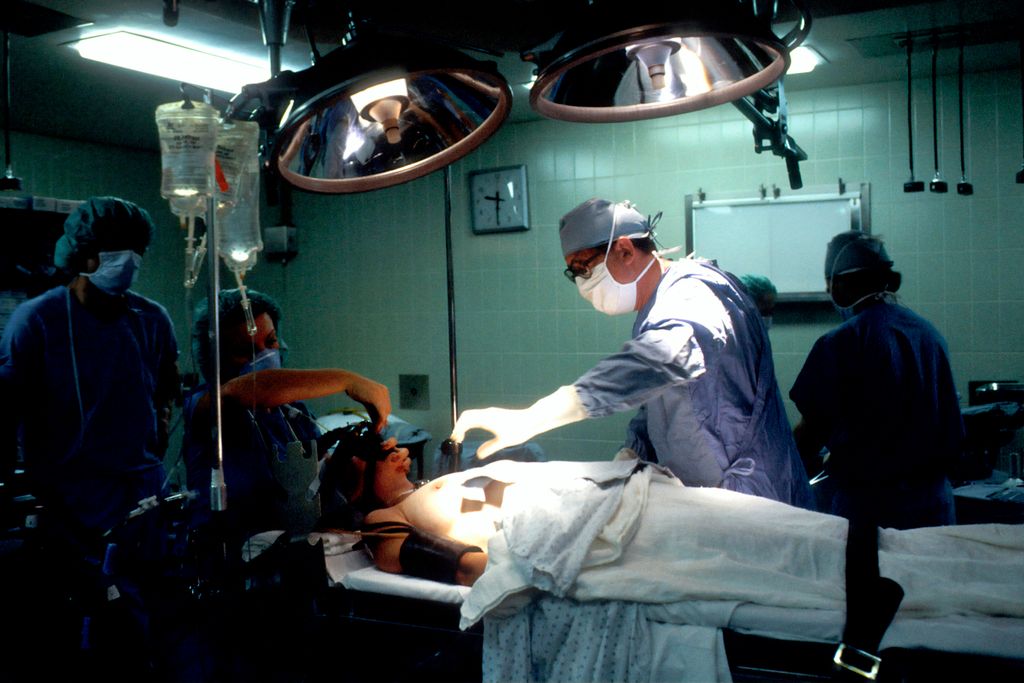
Artificial Intelligence (AI) has made significant strides in revolutionizing the healthcare industry. From regulatory frameworks to the impact on medical professionals, AI continues to shape the way healthcare is delivered and managed. This article delves into the evolution of AI in healthcare, the role of machine learning, and the future prospects of AI in medical research.
Key Takeaways
- Regulatory frameworks play a crucial role in fostering AI innovation in healthcare while addressing potential risks.
- Challenges in AI implementation include data privacy concerns and the need for robust infrastructure and training.
- AI has the potential to significantly impact the medical profession by augmenting decision-making processes and improving patient outcomes.
- Machine learning applications in healthcare are enabling the identification of biomarkers for disorders, enhancing health care delivery, and developing risk prediction models.
- The future of AI in medical research involves establishing networks of AI assurance laboratories, integrating AI in clinical sciences, and addressing ethical considerations in AI adoption.
Evolution of AI in Healthcare
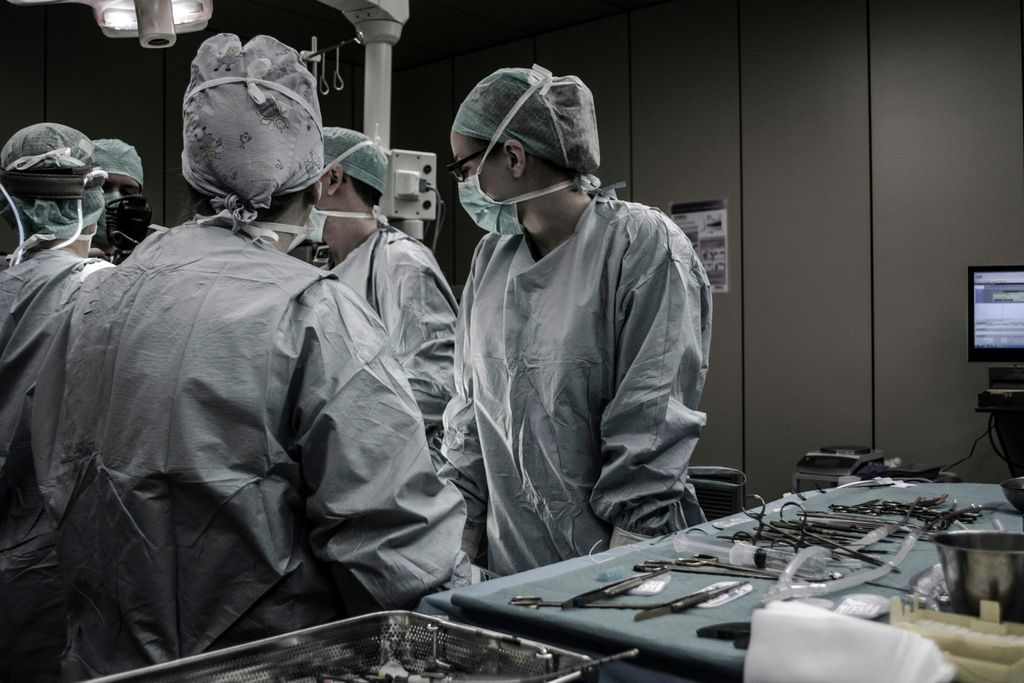
Regulatory Frameworks for AI Innovation
The evolution of AI in healthcare has necessitated the development of robust regulatory frameworks to ensure safety, efficacy, and ethical standards. Regulatory agencies are tasked with balancing the rapid pace of AI innovation with the need for comprehensive oversight. One such framework is the World Health Organization's (WHO) 6 Principles for an AI Regulatory Framework for Medical Product Development, which includes Documentation and Transparency, Risk Management, and Validation among its guidelines.
The challenge lies in creating regulations that are both stringent enough to protect patients and flexible enough to encourage innovation.
To address this, policymakers are exploring various strategies:
- Establishing clear guidelines for the evaluation and development of AI applications in healthcare.
- Prioritizing patient outcomes in the regulatory process.
- Considering the implications of AI on health policy and care quality.
These efforts aim to create an environment where AI can thrive while maintaining the highest standards of patient care.
Challenges in AI Implementation
The integration of AI into healthcare systems presents a myriad of challenges. Accountability remains a significant concern, particularly when AI is involved in treatment decisions. The question of who is responsible when an AI system's recommendation leads to a negative outcome is still unresolved. Additionally, the deployment of generative AI in healthcare is hindered by data-focused strategies that may not fully address the complexities of medical applications.
- Ensuring AI systems are transparent and explainable
- Maintaining data privacy and security
- Overcoming data bias and ensuring equitable AI performance
- Addressing the legal and ethical implications of AI decisions
The effective regulation of AI must balance the need for innovation with the safeguarding of patient welfare and professional standards. This requires a collaborative effort between policymakers, healthcare providers, and AI developers.
The evolution of AI in healthcare demands continuous dialogue and adaptation. As AI systems become more sophisticated, the medical profession must evolve to embrace these changes while preserving the core values of patient care.
Impact on Medical Profession
The integration of artificial intelligence (AI) into healthcare has brought about transformative changes in the medical profession. AI technologies have enhanced the ability of healthcare providers to diagnose and treat diseases, leading to improved patient outcomes. One significant benefit is the increased access to care, as AI can handle a larger volume of patient data and assist in managing more patients effectively.
AI's role in healthcare extends beyond direct patient care. It also influences medical education and training, equipping new generations of medical professionals with the skills to leverage AI tools.
The adoption of AI in healthcare has necessitated a shift in the skill set required for medical professionals. There is a growing need for expertise in data analysis, interpretation of machine learning outputs, and an understanding of AI-driven decision-making processes. This evolution is reflected in various aspects of the medical field, including:
- Medical education and training
- Clinical decision support systems
- Patient information management
- Health policy and regulatory frameworks
As AI continues to advance, it is crucial to address the challenges it poses, such as ensuring the ethical use of AI, maintaining patient privacy, and preventing biases in AI algorithms.
Machine Learning in Health Care
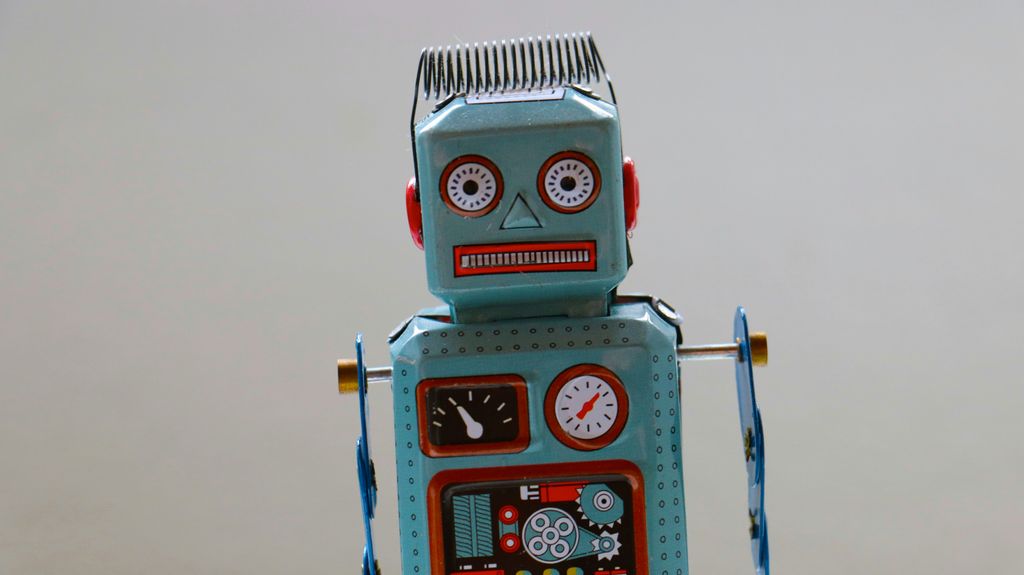
Identifying Biomarkers for Disorders
The quest to identify biomarkers for various disorders is one of the most promising areas where AI is making significant strides. By analyzing vast datasets, AI algorithms can detect patterns that may elude human researchers. For instance, a recent study published in JAMA Psychiatry highlighted the potential of machine learning in identifying a multivariate biomarker for major depressive disorder. This advancement could lead to more accurate diagnoses and personalized treatment plans.
Machine learning's ability to process and learn from large volumes of data is particularly useful in the field of neuroimaging, where subtle changes in brain structure or function may indicate the onset of a disorder. The use of AI in this domain not only accelerates the discovery of biomarkers but also enhances the precision of medical imaging techniques.
The integration of AI into the identification of biomarkers represents a transformative shift in the approach to diagnosing and treating mental health conditions.
While the potential is vast, it is important to recognize the challenges that come with the implementation of AI in healthcare. Ensuring the accuracy and reliability of AI-derived biomarkers is paramount, as is the ethical use of patient data. The following list outlines key considerations in the ongoing development of AI for identifying biomarkers:
- Ensuring data privacy and security
- Validating AI algorithms against clinical outcomes
- Addressing potential biases in AI models
- Fostering interdisciplinary collaboration between AI experts and healthcare professionals
Improving Health Care Delivery
The integration of AI into health care delivery is transforming the landscape of patient care. AI-driven systems are enhancing the efficiency and accuracy of diagnoses, leading to more personalized treatment plans. For instance, AI algorithms can analyze vast amounts of data to identify patterns that may be invisible to the human eye, aiding in the early detection of diseases.
Telemedicine has emerged as a key area where AI is making significant strides. By leveraging AI, telemedicine platforms can offer more accurate and timely medical advice, reducing the need for in-person visits and thus alleviating the strain on healthcare facilities.
- Streamlined administrative processes
- Enhanced patient engagement
- Improved resource allocation
The potential of AI to revolutionize health care delivery is immense, offering a pathway to more accessible and higher-quality care for all.
The adoption of AI in health care also extends to the management of health systems. Predictive analytics are being used to forecast patient admissions, helping hospitals manage their workforce and resources more effectively. Moreover, AI applications in medical coding and auditing are setting new standards for billing accuracy and compliance.
Risk Prediction Models
The advent of machine learning (ML) has revolutionized the field of risk prediction in healthcare. Predictive models are now integral tools for forecasting various health outcomes and guiding clinical decision-making. For instance, a model developed at UC San Diego has shown a significant reduction in mortality by predicting sepsis risk in emergency departments.
One of the key applications of these models is in the realm of hospital mortality. They are designed to identify patients at high risk and facilitate timely interventions. However, these models require hospital-level validation and recalibration to effectively guide mortality reduction interventions.
The potential of ML in improving patient outcomes is immense, yet it hinges on the continuous refinement and validation of the predictive algorithms.
Risk prediction models have also been employed to track disease progression, such as Alzheimer's, and to predict treatment responses in conditions like acute decompensated heart failure. The STOP-RVF Score, for example, is a multicenter risk model that predicts right ventricular failure after mechanical circulatory support.
Here are some notable risk prediction models and their applications:
- UC San Diego AI for Sepsis Risk
- Machine Learning Tool for Heart Failure Treatment Response
- Predictive Model for Alzheimer's Disease Progression
- STOP-RVF Score for Right Ventricular Failure
Future of AI in Medical Research
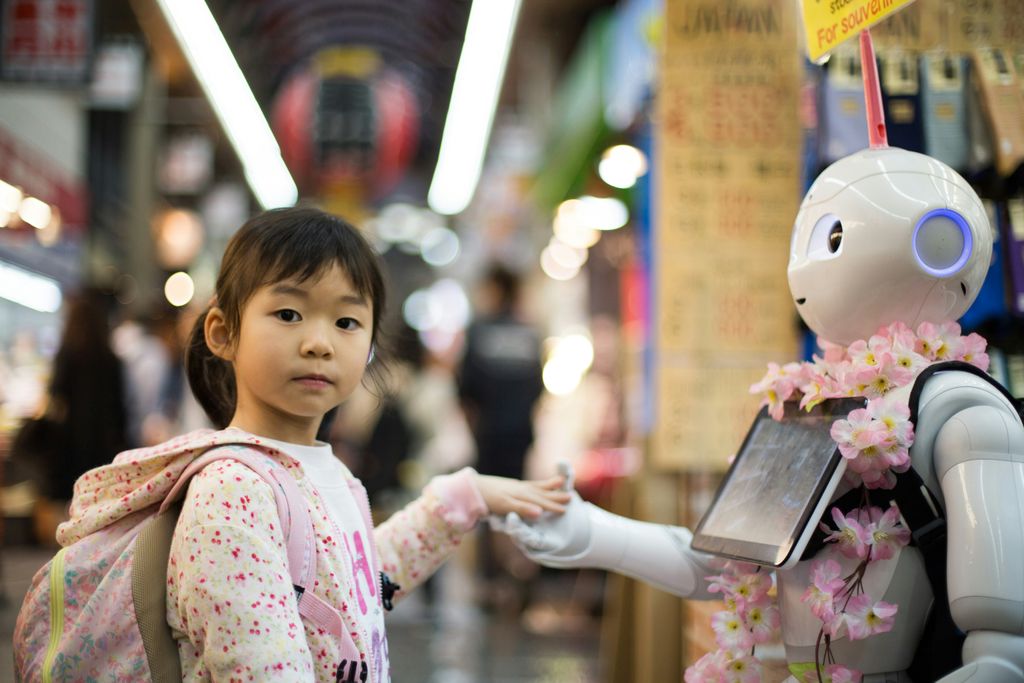
Network of AI Assurance Laboratories
The establishment of a nationwide network of AI assurance laboratories is a pivotal step in the evolution of AI in healthcare. These laboratories, envisioned as a collaborative effort between public and private sectors, are designed to rigorously evaluate and develop AI technologies for health care applications. The JAMA Review from January 16, 2024, emphasizes the importance of such a network in ensuring the quality and safety of AI systems before they are deployed in clinical settings.
The network of AI assurance laboratories will serve as a cornerstone for maintaining high standards in AI healthcare solutions, fostering trust among healthcare professionals and patients alike.
The laboratories will focus on several key areas, including but not limited to:
- AI and Society
- AI for Healthcare and Life Sciences
- Biological and Medical Devices and Systems
- Computational Fabrication and Manufacturing
Each area will contribute to a comprehensive understanding of AI's role and impact in healthcare, ensuring that AI technologies are not only innovative but also reliable and ethical.
AI in Clinical Sciences
The integration of AI into clinical sciences is revolutionizing the way we approach medical research and patient care. AI algorithms are now capable of analyzing complex medical data, such as imaging and genetic information, to assist in diagnosis and treatment planning. This has led to the development of advanced machine-learning models that can predict diseases like pancreatic cancer at earlier stages, potentially improving patient outcomes.
AI in clinical sciences is not just about data analysis; it's about translating these insights into actionable technologies. Research labs and centers are actively engaged in harnessing AI to create innovative healthcare solutions. For instance, the Jameel Clinic and affiliated groups are working on AI applications that range from early disease intervention to enhancing life sciences research.
The potential of AI in clinical sciences is vast, with ongoing research exploring various applications:
- Developing AI-based risk prediction models
- Enhancing diagnostic accuracy through machine learning
- Creating personalized treatment plans
- Improving clinical decision support tools
The promise of AI in clinical sciences lies in its ability to transform vast amounts of data into meaningful insights, which can lead to more informed and effective healthcare interventions.
Ethical Considerations in AI Adoption
The integration of AI into healthcare raises significant ethical questions that must be addressed to maintain trust and integrity in the medical profession. Patient privacy and data security are paramount, as AI systems often require access to sensitive health information. A proactive, multi-layered approach is essential for minimizing security risks and protecting patient data.
Transparency in AI development and deployment is another critical ethical consideration. Patients and healthcare providers must understand how AI tools make decisions and the potential biases these systems may carry. This understanding is crucial for informed consent and for maintaining the human element in healthcare decision-making.
To ensure ethical AI adoption, a set of guiding principles may be established:
- Respect for patient autonomy and informed consent
- Adherence to data protection and privacy standards
- Commitment to transparency and explainability of AI systems
- Continuous monitoring for and mitigation of biases
- Prioritization of patient outcomes over technological advancement
The ethical deployment of AI in healthcare is not just a regulatory requirement but a moral imperative to uphold the dignity and rights of patients.
Conclusion
In conclusion, the integration of artificial intelligence (AI) in healthcare has shown immense potential in revolutionizing the industry. From improving decision-making processes to enhancing patient outcomes, AI has proven to be a valuable tool in advancing healthcare practices. However, it is crucial to address the risks and challenges associated with AI implementation, such as regulatory frameworks and ethical considerations. Moving forward, continued research and collaboration between healthcare professionals and AI experts will be essential in maximizing the benefits of AI while mitigating potential drawbacks. The future of healthcare undoubtedly lies in the innovative applications of AI technology.
Frequently Asked Questions
What is the current regulatory framework for AI innovation in healthcare?
The regulatory framework for AI innovation in healthcare is constantly evolving to accommodate technological advancements while ensuring patient safety and data privacy.
What are some challenges in implementing AI in healthcare?
Challenges in implementing AI in healthcare include data privacy concerns, integration with existing systems, and ensuring the ethical use of AI algorithms.
How does AI impact the medical profession?
AI impacts the medical profession by enhancing diagnostic accuracy, improving treatment outcomes, and streamlining administrative tasks to allow healthcare professionals to focus more on patient care.
How is machine learning used to identify biomarkers for disorders in healthcare?
Machine learning is used to analyze large datasets and identify patterns that can serve as biomarkers for various disorders, aiding in early detection and personalized treatment.
What are some future applications of AI in medical research?
Future applications of AI in medical research include establishing a network of AI assurance laboratories, integrating AI into clinical sciences, and addressing ethical considerations in AI adoption.
What are the potential ethical considerations in adopting AI in healthcare?
Ethical considerations in adopting AI in healthcare include ensuring transparency in AI algorithms, maintaining patient confidentiality, and addressing biases in AI decision-making processes.