AI in Finance: Transforming Banking Services
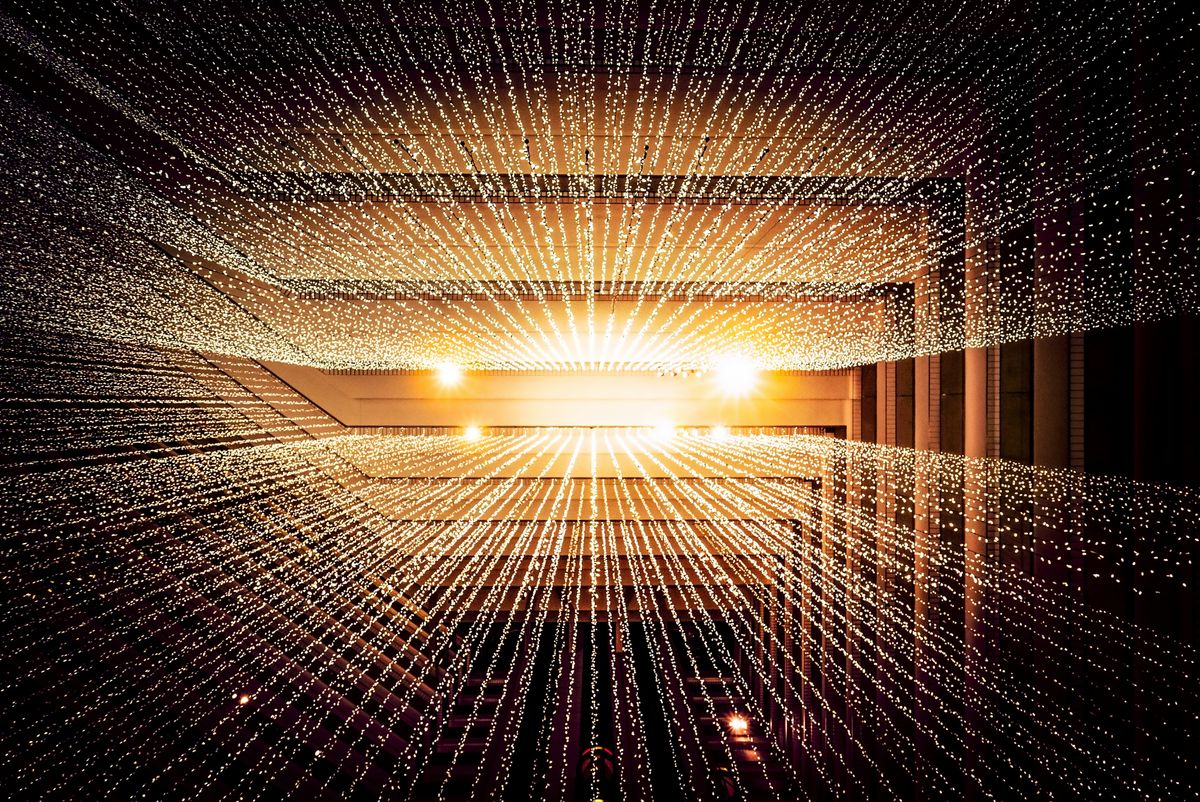
In an era where technology intertwines intimately with daily operations, the financial sector stands on the cusp of a revolution, primarily fueled by Artificial Intelligence (AI). Innovations such as predictive analytics, algorithmic trading, and generative AI are reshaping the landscape of banking services, from customer interactions to risk management. As AI continues to evolve, it promises to further streamline banking operations, enhance customer experiences, and revolutionize financial trading and compliance processes.
Key Takeaways
- AI-driven personalization and service automation are enhancing customer experiences and operational efficiency.
- Predictive analytics and AI are pivotal in transforming risk assessment, fraud detection, and regulatory compliance.
- Algorithmic trading and AI-powered investment strategies are significantly impacting financial markets and asset management.
- Generative AI is catalyzing innovation in banking, leading to tailored products and transformative business models.
- The convergence of AI with embedded finance and BaaS is creating seamless financial experiences beyond traditional banking interfaces.
The Advent of AI in Customer Service and Personalization
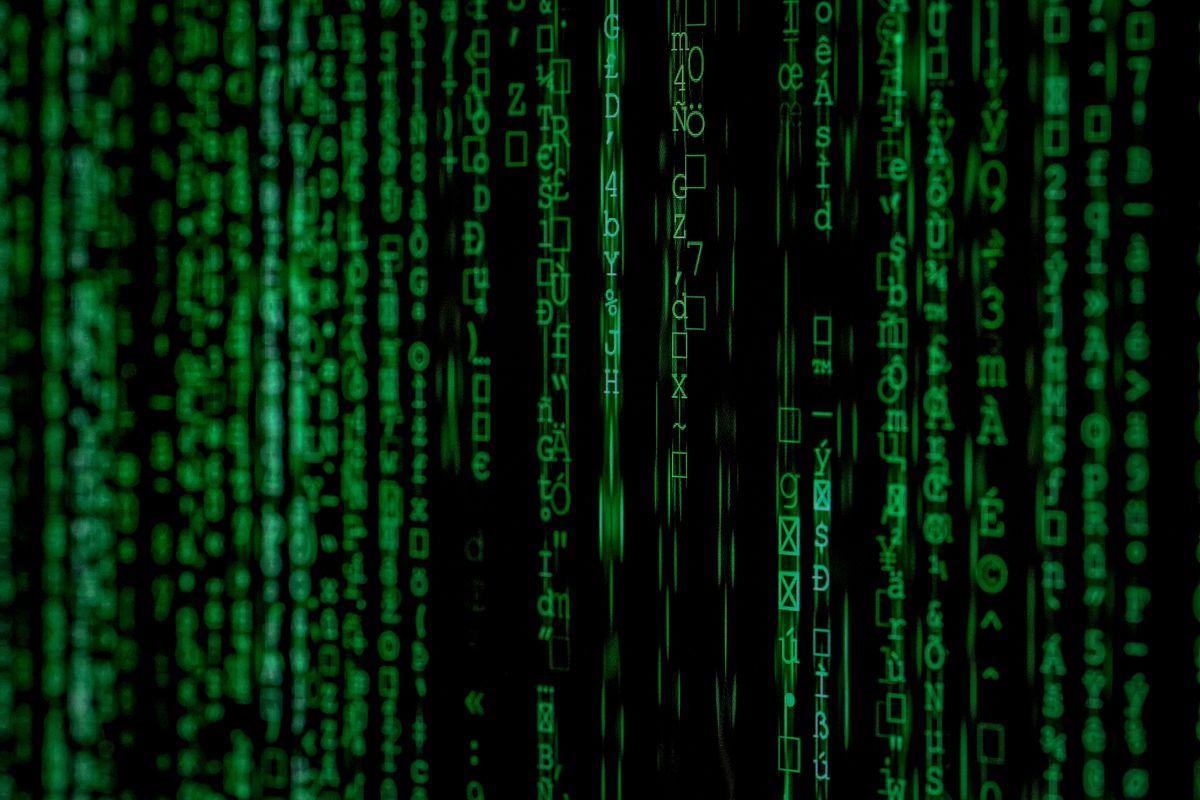
Enhancing Customer Interactions with Personalized Experiences
The integration of AI in banking has led to a significant shift in how financial institutions interact with their customers. AI-driven personalized financial recommendations and chatbot assistance are at the forefront of this transformation. By analyzing vast amounts of customer data, such as transaction history and spending patterns, AI can deliver hyper-personalized experiences that cater to individual financial goals and needs.
- Personalized Customer Experiences
- Increased Productivity and Efficiency
- Advanced Customer Interactions
These personalized experiences are not just about convenience; they represent a strategic move towards deepening customer relationships and fostering loyalty. The efficiency gained through AI not only enhances customer satisfaction but also contributes to cost reduction for the banks.
The use of AI in customer service is a game-changer, enabling banks to offer a level of personalization that was previously unattainable.
Streamlining Service Automation for Efficiency
The integration of AI in the banking sector has led to significant advancements in service automation, enhancing both cost optimization and process efficiency. By automating routine tasks, banks can reduce operational costs, a move that is particularly beneficial given the high proportion of bank workforces susceptible to automation. This not only trims expenses but also allows employees to focus on more complex and value-adding activities.
In the realm of customer support, AI-driven solutions streamline interactions by automating report generation and performing sentiment analysis on unstructured text data. These capabilities ensure that customers receive personalized financial advice, tailored to their interactions and preferences, without undue delay. The efficiency gained through these automated processes is a testament to AI's transformative power in the banking sector.
The swift and informed decisions made possible by AI's data processing and analysis capabilities are reshaping the landscape of banking, leading to improved customer engagement and satisfaction.
- Cost optimization
- Process efficiency
- Automated customer support
- Personalized financial advice
Utilizing Predictive Analytics for Customer Behavior Insights
The integration of predictive analytics into retail banking is reshaping how financial institutions understand and engage with their customers. By analyzing vast amounts of data, AI systems can identify historical patterns and predict future trends in customer behavior, enabling banks to tailor their services to meet evolving expectations.
- Data-driven decision-making
- Streamline customer support
- Automate report generation
- Perform sentiment analysis
- Generate personalized financial advice
This approach not only enhances the customer experience but also drives operational efficiency. For instance, predictive analytics can be used to streamline customer support by anticipating issues and automating report generation, thus reducing response times and improving service quality.
Predictive analytics is pivotal in transforming banks' ability to respond more effectively to customer needs, focusing on key areas such as geospatial analytics, product analytics, and fraud prevention.
Revolutionizing Risk Management and Fraud Detection
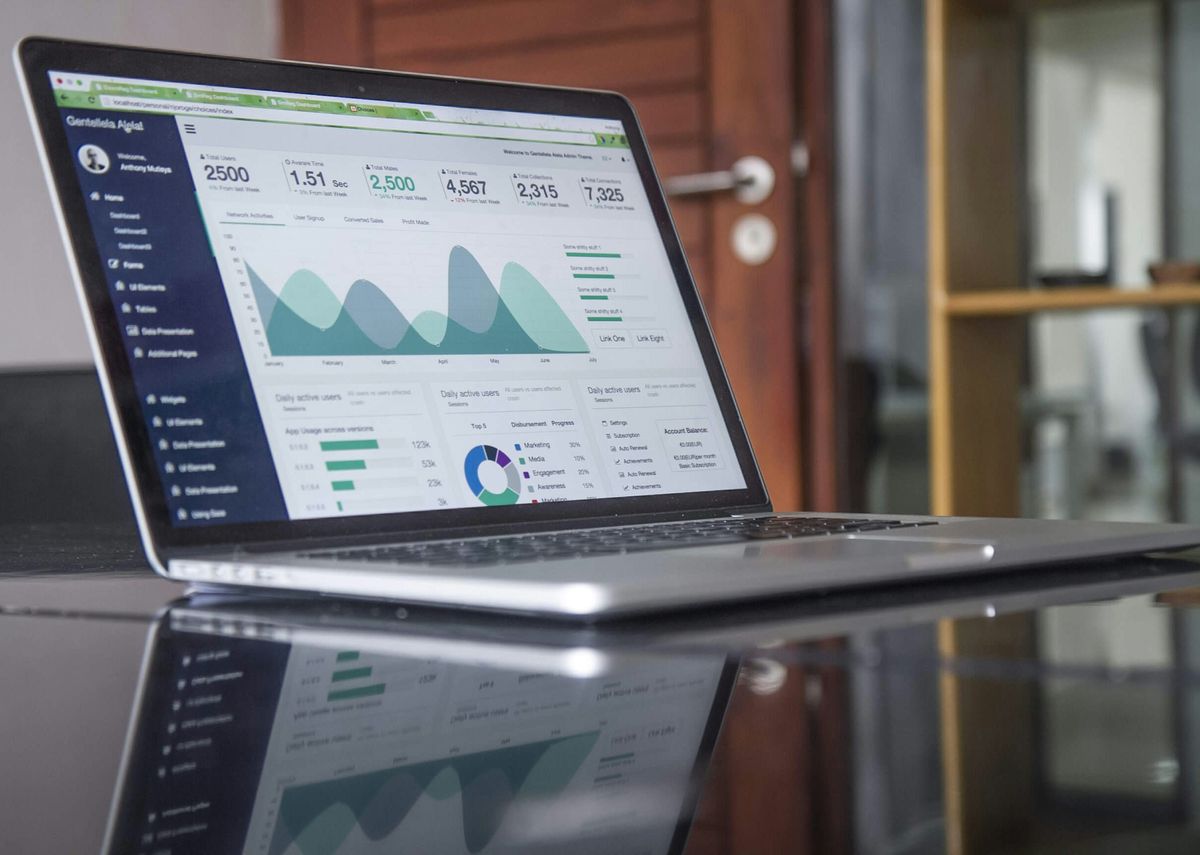
Improving Credit Scoring and Risk Assessment with AI
The integration of AI into credit scoring and risk assessment has marked a significant leap forward for financial institutions. AI-driven models offer a more nuanced understanding of credit risk, enabling banks to make more informed lending decisions. These models analyze a vast array of data points, from transaction histories to social media activity, to assess a borrower's creditworthiness with greater precision.
AI is employed in banks' risk management by utilizing machine learning algorithms to analyze extensive financial data. This not only enhances fraud detection but also streamlines the credit scoring process. By automating the evaluation of risk factors, AI systems can quickly identify potential red flags that might be missed by traditional methods.
The benefits of AI in this domain are manifold:
- Improved accuracy in credit scoring
- Faster risk assessment processes
- Enhanced ability to predict default risks
AI's predictive capabilities are not just about avoiding risks; they're also about seizing opportunities. By accurately assessing the likelihood of repayment, banks can offer more competitive rates to reliable borrowers, fostering a healthier credit environment.
Advancements in Real-Time Fraud Detection Systems
The integration of generative AI (Gen AI) into real-time fraud detection systems marks a significant leap forward in the financial industry's ability to combat fraudulent activities. Mastercard's new generative AI model exemplifies this advancement, offering banks a substantial increase in fraud detection rates. By analyzing the vast amount of transaction data, these AI models can discern intricate patterns and anomalies that may indicate fraudulent behavior.
- Gen AI techniques, such as Generative Adversarial Networks (GANs), are instrumental in creating synthetic fraudulent transactions. This enhances the training of fraud detection models, leading to:
- Improved robustness
- Greater accuracy
Generative AI models are not only adept at identifying potential risks but also in simulating various economic scenarios to assess and mitigate different types of risks, including credit, market, and operational risks.
Furthermore, the predictive capabilities of Gen AI allow for a more proactive approach to fraud detection, identifying risks before they materialize. This proactive stance is crucial in preserving the profitability and integrity of financial institutions.
Leveraging AI for Regulatory Compliance and Reporting
The integration of generative AI into regulatory compliance processes marks a significant advancement in the banking sector. Banks are now equipped to monitor regulations in real-time, ensuring that they remain compliant amidst the ever-changing landscape of financial regulations. This not only streamlines the compliance workflow but also reduces the burden of manual oversight.
For instance, Citigroup's use of generative AI to analyze extensive regulatory documents exemplifies the practical benefits of AI in compliance. By automating the analysis of 1,089 pages of new capital rules, the bank's risk and compliance team could swiftly adapt to regulatory changes, setting a precedent for other financial institutions.
Moreover, generative AI aids in customer due diligence, a critical component of KYC compliance regulations. The technology's ability to process vast amounts of data can significantly expedite customer onboarding, minimize false positives, and improve risk assessments, all while adhering to AML and KYC standards.
The proactive use of AI in compliance not only mitigates risks but also empowers banks to navigate the complexities of regulatory environments with greater agility and precision.
Optimizing Financial Trading with AI
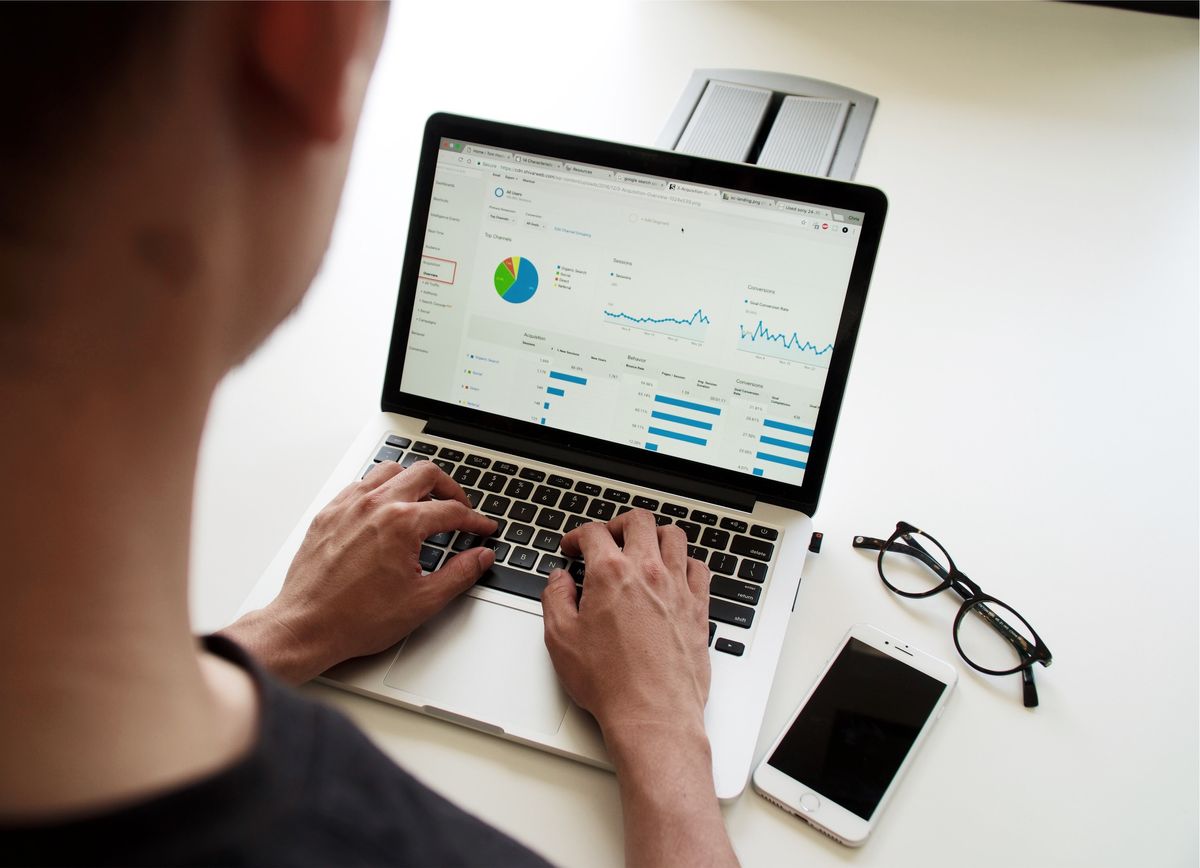
The Impact of Algorithmic Trading on Market Dynamics
Algorithmic trading, powered by AI, has significantly altered the landscape of financial markets. Real-time trading algorithms have introduced a level of speed and efficiency previously unattainable, enabling trades to be executed in milliseconds. This rapid pace has enhanced market liquidity and facilitated the entry of new market participants.
Algorithmic trading also brings a layer of sophisticated risk management, allowing for more precise control over trading strategies. However, the responsible deployment of these technologies is crucial to maintain market stability and foster investor confidence. As AI reshapes industries, it's essential to ensure these advancements enrich human endeavors rather than create unwarranted volatility.
The integration of AI in trading strategies has not only improved accuracy but also underscored the importance of transparency and trust in financial decisions. The use of Explainable AI and methods like SHAP in financial engineering is a testament to the industry's commitment to responsible innovation.
While the benefits are clear, it's important to recognize the potential challenges that come with the integration of AI in finance. Ethical considerations and the need for robust regulatory frameworks are paramount to ensure that the evolution of trading algorithms continues to serve the broader interests of the market.
Predictive Analytics in Asset Management
The integration of predictive analytics in asset management has been a game-changer for the finance industry. By harnessing the power of AI, asset managers can now anticipate market movements and adjust their strategies accordingly. Predictive analytics tools sift through vast datasets, identifying patterns and signals that are imperceptible to the human eye, thus offering a competitive edge in investment decision-making.
- Identification of potential investment opportunities
- Risk mitigation through early detection of market shifts
- Enhanced portfolio diversification based on predictive insights
Predictive analytics in asset management not only sharpens the accuracy of forecasts but also democratizes access to sophisticated investment strategies, previously available to only a select few with deep market knowledge.
The future of asset management is undeniably intertwined with the advancement of predictive analytics, which continues to evolve and refine the precision of financial predictions. As we move forward, the role of AI in asset management will become even more pronounced, leading to more robust and resilient investment portfolios.
AI-Driven Investment Strategies and Portfolio Management
The integration of AI into investment strategies and portfolio management marks a significant shift in the financial industry. AI-driven tools are enhancing the precision of investment recommendations, leveraging vast amounts of market data to inform decisions. These tools utilize trend analysis and predictive models to identify potential investment opportunities and optimize portfolio allocations.
Generative AI, in particular, is showing promise in its ability to propose and evaluate new trading strategies. By simulating different market scenarios, it aids in uncovering profitable ventures while aiming to minimize risks. The financial sector is still in the early stages of adopting generative AI for these purposes, but the potential for transformative impact is clear.
The use of AI in finance is not just about automation; it's about enabling smarter, data-driven decisions that can lead to better financial outcomes for both institutions and their clients.
While the future of AI in finance is bright, it is important to approach its integration with caution, ensuring that ethical considerations and risk management are at the forefront of its application.
Generative AI: The Future of Banking Innovation
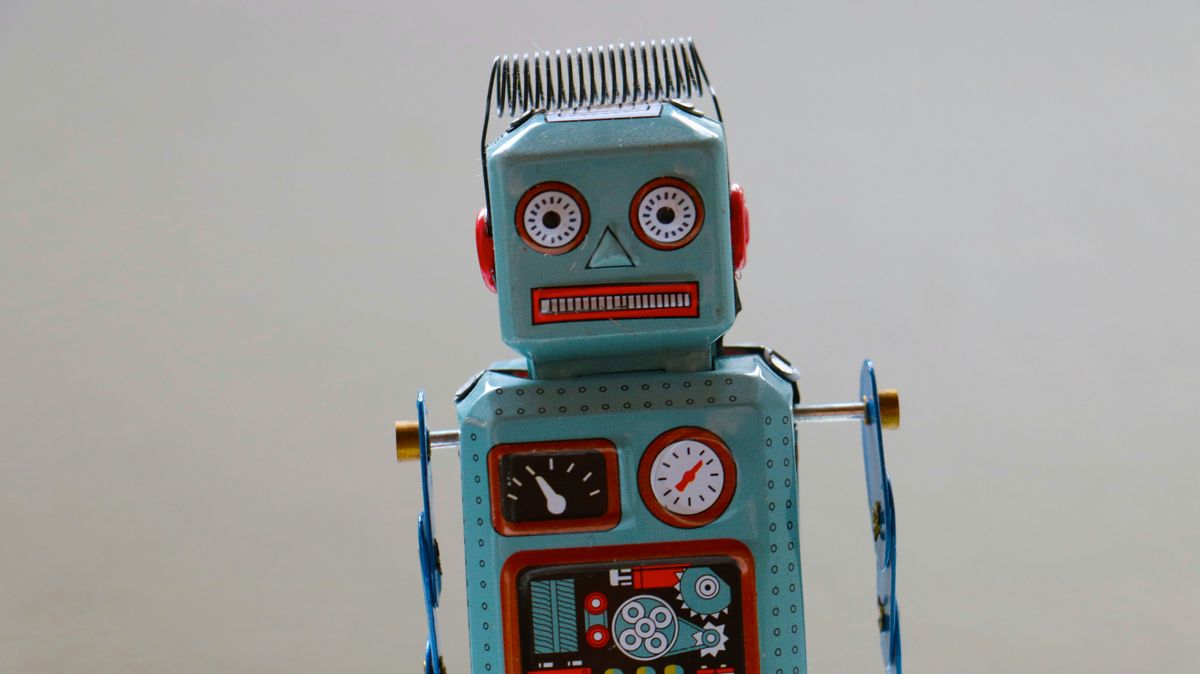
Tailoring Banking Products with Generative AI
The integration of generative AI into the banking sector marks a significant shift towards more adaptive and intuitive systems. Banks are now able to offer highly personalized products and services by leveraging AI's capability to analyze extensive datasets and understand market trends and customer preferences. This not only meets the evolving demands of customers but also provides banks with a distinct competitive edge.
Generative AI's potential extends to various applications within the banking industry, from enhancing customer service to innovating back-office operations. It enables the creation of new banking models that are more efficient and customer-centric. For instance, generative AI can be used to:
- Streamline customer support through natural language processing
- Automate the generation of reports
- Perform sentiment analysis on unstructured data
- Develop tailored financial products based on individual customer data
The convergence of generative AI with banking is transforming traditional services into differentiated, secure, and efficient offerings. It's not just an evolution of technology but a redefinition of banking itself.
As we witness the rise of generative AI in the banking industry, it's clear that its impact is profound, touching upon all aspects of banking operations and decision-making. In a data-rich environment where customer interaction is key, generative AI stands as a pivotal element in shaping the future of banking.
Gen AI as a Catalyst for Banking Business Model Transformation
The integration of generative AI (Gen AI) within the banking sector marks a pivotal shift, not merely in operational efficiency but as a driving force for innovative banking business models. By harnessing Gen AI, banks can rapidly analyze extensive datasets, enabling them to stay ahead of market trends and align with customer preferences, thus securing a competitive edge.
Gen AI’s role extends beyond cost savings, fostering innovation and tailoring products to meet the dynamic needs of customers.
Some key applications of Gen AI in banking include:
- Fraud detection
- Customer service automation
- Credit score calculations and risk assessment
- Algorithmic trading
- Market trend and customer behavior prediction
Each innovation in FinTech has incrementally transformed banking functions, but Gen AI is reshaping the entire industry. It redefines banking operations and decision-making in a data-rich environment where customer interaction and a substantial workforce are crucial.
Real-life Examples of Generative AI Applications in Banking
The integration of Generative AI (Gen AI) in banking is not just a theoretical concept; it's a burgeoning reality with several institutions leading the charge. Banks such as Swift, ABN Amro, and ING Bank are trailblazers, experimenting with Gen AI to enhance their operations and customer service.
Gen AI's potential extends beyond routine task automation, serving as a catalyst for innovation and tailored banking solutions.
For instance, Fujitsu and Hokuhoku Financial Group's joint trials represent a significant stride towards realizing Gen AI's capabilities in banking. They are exploring use cases like generating responses to internal inquiries, creating and checking business documents, and even building programs. This practical application of Gen AI is indicative of the technology's versatility and its ability to streamline complex banking processes.
Moreover, the technology's prowess in analyzing vast datasets allows banks to rapidly adapt to market trends and customer preferences, securing a competitive edge. Here are some key applications of Gen AI in banking:
- Automating routine tasks and customer inquiries
- Generating and verifying business documents
- Tailoring products and services based on data analysis
- Synthesizing data for training other AI models
The evolution of banking, from physical establishments to a digital realm, has been significantly accelerated by Gen AI, marking a new era of banking innovation.
Embedded Finance and AI: A New Paradigm
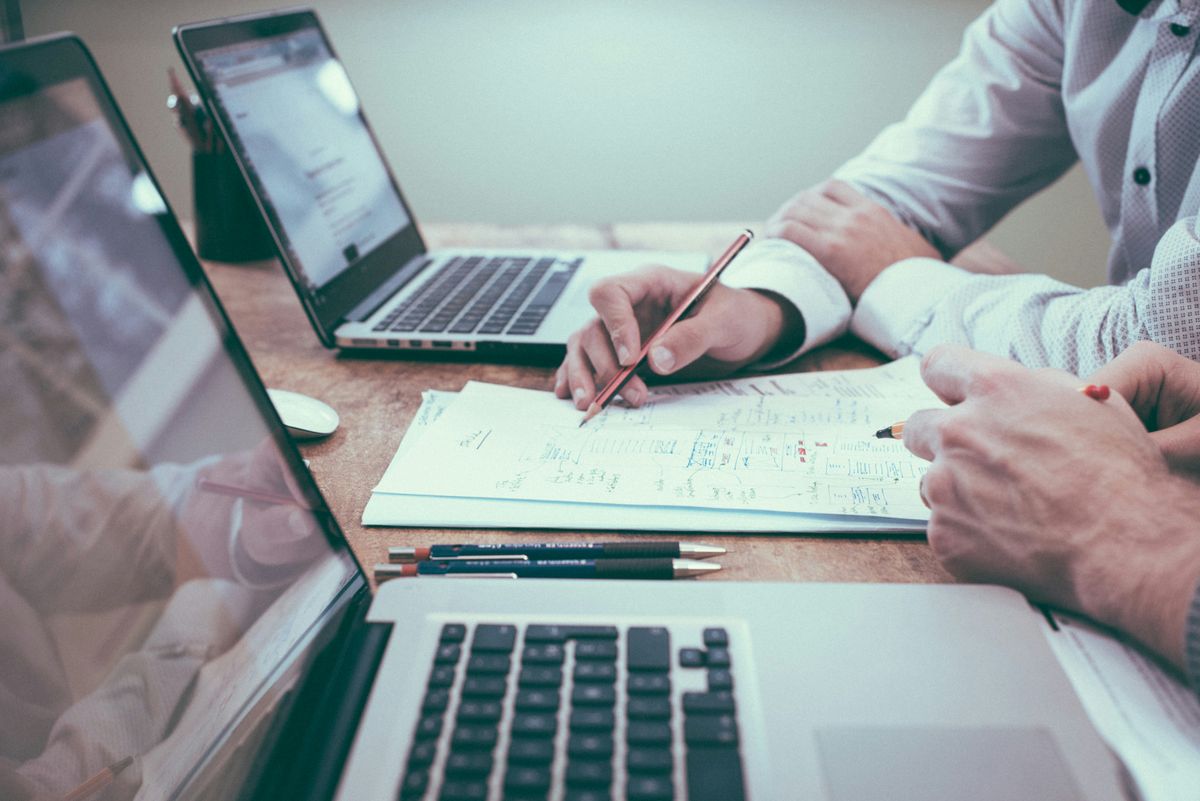
The Role of AI in Embedded Finance Solutions
Artificial intelligence (AI) is transforming the landscape of embedded finance, enabling seamless integration of financial services into non-banking platforms. AI-driven embedded finance solutions are not just about convenience; they represent a paradigm shift in how consumers access and use financial products.
Embedded finance, facilitated by AI, allows for sophisticated risk assessment and credit scoring, enhancing the trustworthiness of lending processes. This integration is particularly impactful in the lending sector, where AI's potential is recognized for its ability to support lenders in improving customer experience and making lending processes more reliable.
- Identity management
- Content delivery
- KYC compliance
- Fraud detection
- Personalization
The synergy between AI and embedded finance is creating new opportunities for both financial and non-financial platforms to address challenges more effectively and offer tailored financial services.
While the benefits are substantial, including reduced technology costs and strengthened security, the deployment of AI must be approached with caution. The absence of emotional intelligence and the reliance on historical data necessitate a responsible use of AI to avoid perpetuating biases and ensure its ethical application.
Banking-as-a-Service (BaaS) and the Integration of AI
Banking-as-a-Service (BaaS) represents a foundational shift in the financial services industry, allowing non-banking platforms to offer banking services by integrating with banks' systems through APIs. This model has been further enhanced by the integration of Artificial Intelligence (AI), which has enabled a more seamless and personalized user experience.
AI's role in BaaS is multifaceted, ranging from improving operational efficiency to providing personalized customer experiences. For instance, AI-driven data can be leveraged to refine customer scoring models, enhance fraud detection, and tailor banking services to individual needs.
The integration of AI with BaaS has led to the development of innovative solutions that cater to the modern consumer's expectations of banking services without relying on traditional banking interfaces. Here are some key benefits of AI in BaaS:
- Personalized customer experiences through advanced data analysis
- Increased productivity and efficiency in banking operations
- Enhanced security and compliance through automated systems
The synergy between BaaS and AI is creating new opportunities for tech companies and financial institutions to collaborate and innovate, offering advanced financial services that were once the sole domain of traditional banks.
The Convergence of AI and Non-Banking Interfaces
The integration of AI into non-banking interfaces marks a significant shift in the financial landscape. AI-driven strategies are now extending beyond traditional banking platforms, enabling a seamless financial experience for users even without direct interaction with banks. This convergence allows for a more intuitive and personalized approach to financial services, catering to the modern consumer's expectation of convenience and efficiency.
The following points illustrate the transformative impact of AI on non-banking interfaces:
- Identity Management: AI enhances the security and accuracy of identity verification processes.
- Content Personalization: Tailored financial advice and product offerings are made possible through AI's deep learning capabilities.
- KYC and Fraud Detection: AI's predictive analytics improve the detection of fraudulent activities and streamline Know Your Customer (KYC) procedures.
- Embedded Analytics: Financial insights are integrated into non-financial platforms, enriching the user experience.
The synergy between AI and non-banking platforms is not just about technological integration; it's about redefining the very essence of customer engagement in finance.
As AI continues to evolve, its role in non-banking interfaces is expected to grow, further blurring the lines between financial services and everyday digital interactions. The Ethical Droid's focus on data analysis, customer service, and ethical considerations underscores the importance of AI in enhancing decision-making and support systems within the financial sector.
Conclusion
The integration of AI into the financial sector marks a pivotal shift towards more innovative, efficient, and personalized banking services. As we have explored, AI's applications in finance, from fraud detection to customer service automation and algorithmic trading, are not only enhancing operational efficiency but also redefining customer experiences. The emergence of Generative AI further accelerates this transformation, offering financial institutions the tools to innovate and adapt in a rapidly evolving market. While the journey ahead may present challenges, the potential for AI to revolutionize banking is undeniable, promising a future where financial services are more accessible, secure, and tailored to individual needs.
Frequently Asked Questions
How is AI transforming customer service in banking?
AI is transforming customer service in banking by personalizing interactions with customers, utilizing predictive analytics to understand customer behavior, and automating routine tasks to enhance efficiency and response times.
What role does AI play in risk management and fraud detection?
AI plays a crucial role in risk management and fraud detection by improving credit scoring models, providing real-time fraud detection systems, and aiding in regulatory compliance through advanced data analysis and pattern recognition.
How is AI optimizing financial trading?
AI optimizes financial trading through algorithmic trading that affects market dynamics, predictive analytics for asset management, and AI-driven strategies for investment and portfolio management.
What is Generative AI and how is it innovating banking?
Generative AI (Gen AI) refers to AI systems that generate new content or data. In banking, it's innovating by tailoring banking products, acting as a catalyst for business model transformation, and providing real-life applications such as personalized customer experiences and enhanced decision-making.
What is embedded finance and how does AI enhance it?
Embedded finance integrates financial services within non-financial platforms. AI enhances embedded finance by enabling seamless integration of banking services into various digital interfaces, improving identity management, KYC processes, and personalization.
What are some of the traditional AI systems used in banking?
Traditional AI systems in banking include machine learning models for fraud detection, customer service automation, credit score calculations, risk assessment, and algorithmic trading, all of which help in recognizing patterns and predicting future trends.