AI in Finance
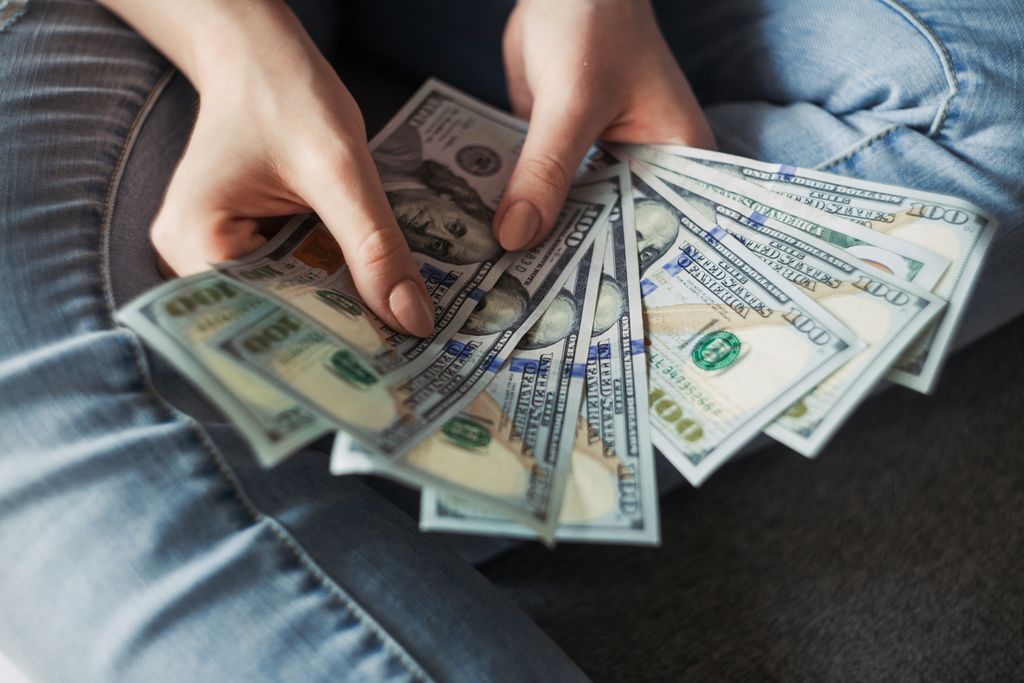
AI is revolutionizing the finance industry with its capabilities to enhance financial analysis, banking operations, and investing strategies. As AI continues to evolve, it is crucial to understand its role and potential in shaping the future of finance. This article explores the key aspects of AI in finance and dispels common misconceptions surrounding its implementation and impact on the industry.
Key Takeaways
- AI plays a significant role in financial analysis by integrating human expertise with advanced algorithms and technologies.
- Challenges in data processing highlight the importance of human oversight in AI-driven financial tasks.
- The future potential of AI in credit scoring lies in its ability to automate productive tasks and drive technological advancements in banking operations.
- Misconceptions about AI in finance, such as the myth of a singular algorithm, emphasize the importance of dynamic ML models and the human element in AI-driven investing.
- AI implementation in banking operations is reshaping the industry by automating productive tasks and paving the way for future innovations in technology and customer services.
The Role of AI in Financial Analysis
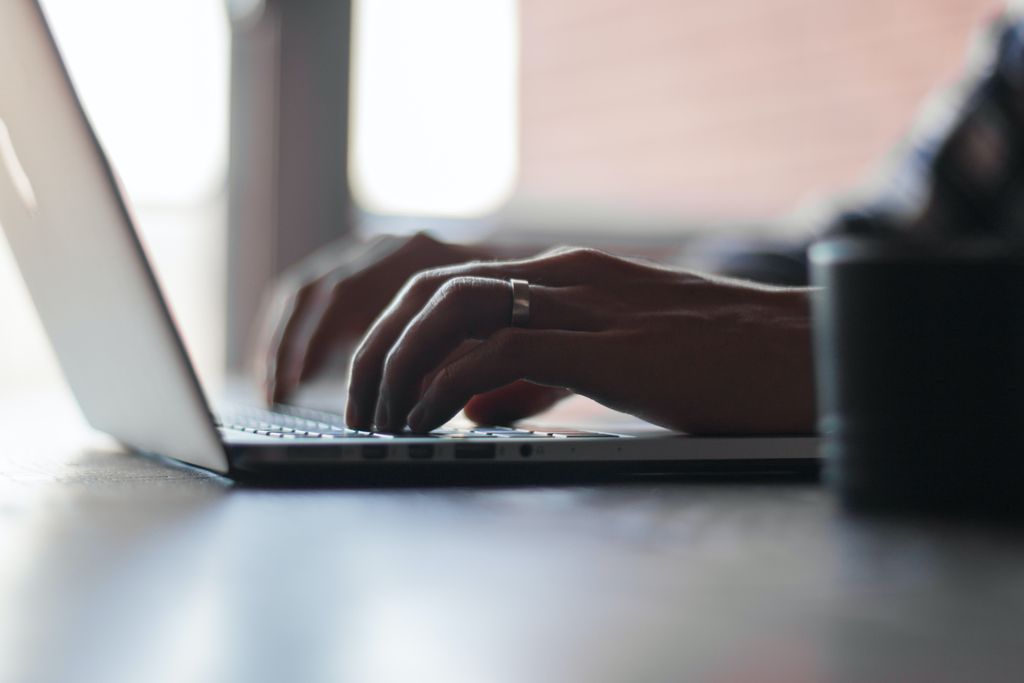
Integrating Human Expertise
The advent of AI in financial services has not diminished the value of human intelligence, which is crucial for learning, adapting, and applying knowledge in the sector. AI represents a paradigm-altering moment in history, reshaping how managers navigate the intricacies of investing. However, the idea that AI surpasses human capability is an oversimplification of the dynamic relationship between technology and human expertise.
Rather than seeing AI as a competitor, it should be viewed as a complementary force that, when combined with human insight, can address challenges more effectively. This synergy is not about claiming superiority but about fostering collaboration to leverage the strengths of both domains. By integrating the domain expertise of financial professionals with AI's rapid pattern recognition and emotion-free decision-making, a more robust approach to investing emerges.
Dedication to continuous innovation is essential in this rapidly evolving landscape. What is considered cutting-edge today may become obsolete tomorrow, underscoring the importance of adapting to technological advancements.
The following points highlight the benefits of integrating human expertise with AI in financial analysis:
- Enhanced decision-making through combined human intuition and AI analytics
- Improved risk assessment by leveraging human experience with AI's predictive capabilities
- Greater efficiency in identifying market opportunities by merging human strategic thinking with AI's data processing speed
Challenges in Data Processing
The integration of AI in finance is not without its hurdles, particularly when it comes to data processing. Financial data is inherently non-stationary, often messy, inconsistent, and noisy. It's a challenge to ensure that the data fed into AI systems is of high quality and reliability. Bold predictions and nuanced insights depend on the accuracy of this data.
Data science tools are essential in processing historical and present data to make future predictions. However, the expectations for immediate breakthroughs in AI technologies may be overrated, indicating that significant changes may not be imminent. Despite this, AI has demonstrated its effectiveness in areas such as electronic document management, where it enhances performance and accuracy.
The collaboration between domain experts and AI is crucial. Financial analysts and data scientists work together to preprocess data, addressing issues such as inaccuracies, missing values, and inconsistencies. This partnership is vital for the effective use of machine learning models in finance.
The table below outlines the key challenges in data processing for AI in finance:
Challenge | Description |
---|---|
Data Quality | Ensuring accuracy, completeness, and consistency. |
Data Standardization | Harmonizing various data formats and sources. |
Real-time Processing | Managing and analyzing data in a timely manner. |
Scalability | Handling the increasing volume of data. |
Addressing these challenges is essential for AI to reach its full potential in financial analysis and decision-making.
Future Potential in Credit Scoring
The integration of AI in credit scoring is a testament to the technology's transformative power in finance. AI is reshaping the way financial institutions assess creditworthiness, offering a more nuanced approach than traditional methods. By analyzing vast amounts of data, AI models can predict payment behavior with greater accuracy, aiding banks in making well-informed decisions.
Credit scoring models powered by AI not only enhance decision-making but also expand the customer base by efficiently processing diverse information sets. This leads to a reduction in risks and an increase in potential customers. The future of AI in credit scoring is bright, with the promise of further innovations that will refine these processes even more.
The potential of AI in credit scoring extends beyond mere efficiency; it signifies a shift towards more inclusive financial practices.
While the technology is still evolving, the trajectory suggests a significant impact on the financial landscape. The table below outlines the anticipated advancements in AI-driven credit scoring:
Advancement | Description |
---|---|
Enhanced Predictive Analytics | Improved models for assessing payment behavior |
Inclusive Credit Access | Broader evaluation criteria to serve underrepresented groups |
Real-time Decision-making | Faster credit approvals using AI algorithms |
As we look to the future, it is clear that AI will continue to play a pivotal role in the evolution of credit scoring, loan approval, and financial inclusivity.
AI Implementation in Banking Operations
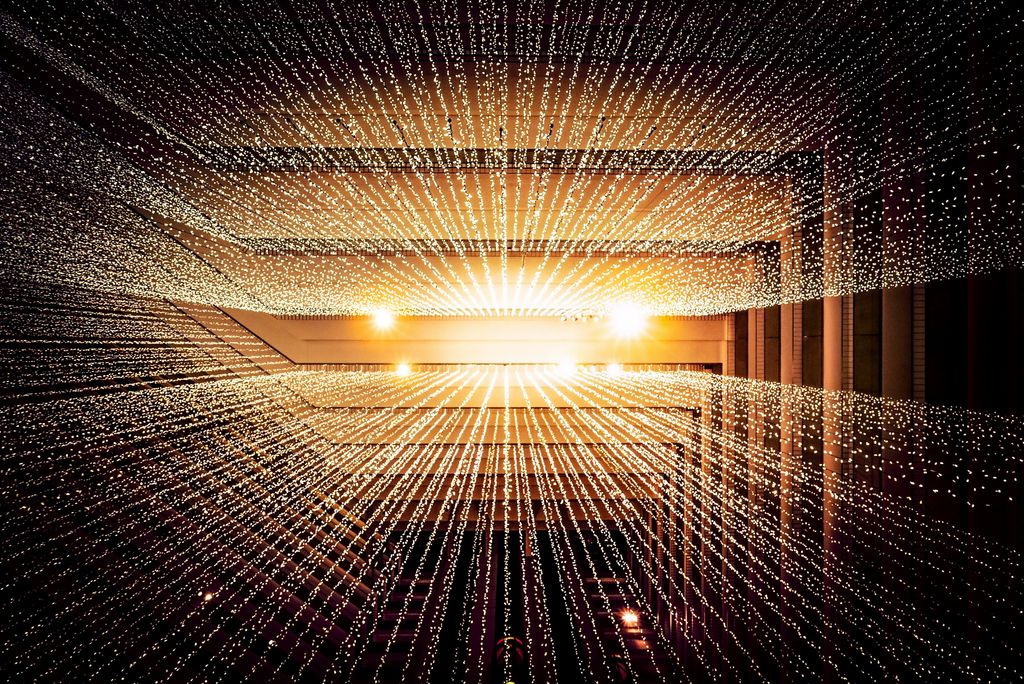
Automating Productive Tasks
The integration of AI in banking operations has led to the automation of numerous productive tasks, enhancing efficiency and customer experience. AI for corporate banking automates tasks, such as data analysis and customer service, freeing up human employees for more complex problem-solving roles. For instance, chatbots powered by AI have revolutionized customer service, providing quick responses to inquiries and reducing the need for extensive human intervention.
Chatbots and automated systems not only handle customer queries but also play a crucial role in fraud detection and market trend prediction. The table below illustrates the impact of AI on various banking operations:
Operation | Impact of AI |
---|---|
Customer Service | Improved response times |
Fraud Detection | Enhanced accuracy |
Market Analysis | Predictive insights |
The ongoing replacement of human personnel with AI agents, particularly in call centers, is a significant shift. This transformation aims to reduce costs and improve service quality, although it is met with challenges such as the need for more humanlike interactions.
While some operational tasks have already reached optimal solutions and may not benefit from AI, others are ripe for innovation. The technology is still evolving, and significant advancements are expected in the near future, particularly in areas like credit scoring.
Technological Advancements
The landscape of banking operations is continuously reshaped by technological advancements in artificial intelligence (AI). These innovations are not just about replacing human effort but enhancing it, leading to more efficient and accurate processes. For instance, AI has significantly improved electronic document management, offering better performance and precision in handling data.
One area that stands to benefit greatly from AI is credit scoring. By analyzing vast amounts of data, AI can provide more nuanced and accurate assessments of creditworthiness, which is crucial for financial decision-making. Here's a brief look at the current state of AI in banking operations:
- Electronic document management
- Data processing and analysis
- Credit scoring enhancements
- International performance through translation
Despite the promise of AI, it's important to manage expectations. While some sectors may not see groundbreaking changes immediately, the trajectory is set for substantial progress in the near future. The key is continuous innovation, as what is cutting edge today may become obsolete tomorrow.
Future Innovations
The landscape of banking and finance is rapidly transforming, driven by the relentless pace of technological innovation. The future of AI in banking is not just about incremental changes, but about reimagining possibilities. The research and development (R&D) efforts of today lay the groundwork for tomorrow's breakthroughs, which may soon surpass our wildest expectations.
The synergy between AI and financial technology (FinTech) companies is crafting enhanced banking solutions, signaling a shift towards more collaborative and integrated financial services.
Looking ahead, we can anticipate AI to play a pivotal role in areas such as credit scoring, where it offers more nuanced insights than traditional methods. This is not just about the technology itself, but about how it empowers banks to make fully informed decisions. The key takeaway for industry leaders is clear: innovate relentlessly and embrace change proactively to stay ahead in the game.
The Misconceptions of AI in Finance
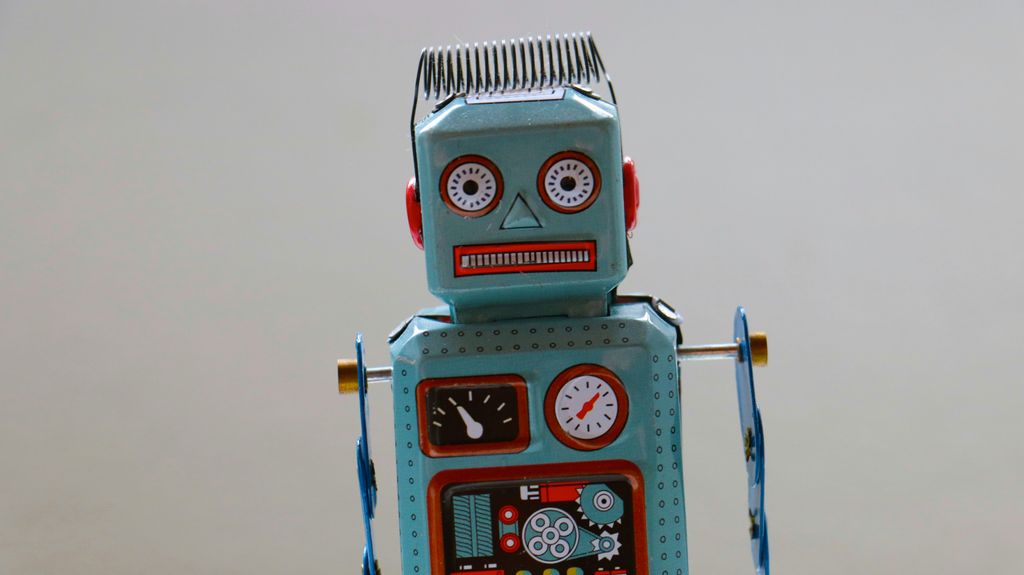
The Myth of a Singular Algorithm
One of the most pervasive misconceptions about AI in the realm of finance is the belief in a single, all-powerful algorithm capable of unraveling the complexities of the market. In reality, the financial landscape is characterized by deep, repeatable patterns that no single algorithm can master. Instead, a dynamic ecosystem of machine learning models is employed to navigate the intricacies of investing.
Investing with AI isn't just about data and algorithms; it's deeply intertwined with human expertise in financial analysis, economics, and geopolitics.
For instance, our approach utilizes 26 distinct models, referred to as "virtual analysts," each fine-tuned to specific objectives and time horizons. These models engage in a symbiotic process of competition and cooperation, ultimately leading to stock recommendations where the most convincing ideas receive the greatest emphasis. This method underscores the fact that human-plus-machine is more effective than either working in isolation.
Domain expertise remains crucial in AI-driven investing. The integration of human knowledge in areas such as fundamental equity analysis and computer science enhances the performance of AI systems. This collaborative synergy ensures that AI in finance is not just a technological tool, but a partnership that leverages the best of both worlds.
The Reality of Dynamic ML Models
The application of AI in finance is often misunderstood as a search for the one algorithm that can unlock all the secrets of the market. However, the reality is far more complex and dynamic. Machine learning (ML) models in finance are not static; they are continuously refined and improved upon. These models are designed to adapt to new data, learning from the market's ever-changing landscape to make more accurate predictions over time.
In the realm of investing, a dynamic ensemble of ML models has proven to be most effective. For instance, one approach involves using multiple models, each with its own specialty in analyzing different aspects of financial data. This method allows for a more comprehensive analysis, as each model brings a unique perspective to the table. Consider the following:
- Model A: Focuses on macroeconomic indicators
- Model B: Analyzes company-specific fundamentals
- Model C: Examines market sentiment and technical indicators
Through a collaborative effort, these models can provide a nuanced understanding of the market, leading to more informed investment decisions. It's a process of competition and cooperation, where the most convincing insights gain prominence.
The synergy between human expertise and machine intelligence is what truly enhances the decision-making process in financial analysis. It's not about replacing one with the other, but rather about leveraging the strengths of both to achieve superior results.
The Human Element in AI-Driven Investing
While AI unlocks formidable analytical capabilities, the human capacity for judgment, ethics, and values remains irreplaceable. The synergy between human expertise and AI's computational power is not just beneficial; it's essential for navigating the complexities of financial markets. Domain expertise, encompassing knowledge of financial analysis, economics, and geopolitics, is crucial in shaping the AI models that drive investment strategies.
The integration of AI in finance is not about replacing human decision-making but enhancing it. AI tools and models are designed to augment the capabilities of financial professionals, not to supplant them.
For instance, in the realm of stock recommendations, a dynamic ensemble of machine learning models, each tuned to different objectives, competes and cooperates to surface the best investment opportunities. However, it is the human touch that adds a layer of strategic oversight, ensuring that the highest-conviction ideas align with broader economic and ethical considerations.
- Human expertise is vital for feature engineering and model tuning.
- A dynamic ecosystem of machine learning models captures financial complexities.
- The collaboration between humans and AI leads to more effective problem-solving.
In conclusion, the human element in AI-driven finance is not just a complementary aspect; it is a cornerstone that ensures AI applications are grounded in real-world context and human values.
Conclusion
In conclusion, AI is revolutionizing the finance industry by reshaping financial forecasts, improving credit scoring, and enhancing investment strategies. While AI offers immense potential for automation and efficiency, it is crucial to remember that human expertise and domain knowledge play a vital role in the successful implementation of AI in finance. As technology continues to advance, the collaboration between AI algorithms and financial experts will drive innovation and create new opportunities in the financial sector.
Frequently Asked Questions
How is AI reshaping financial forecasts and disclosure?
AI is reshaping financial forecasts and disclosure by making language more important than ever, emphasizing the need for accurate and insightful communication in financial analysis.
What is the role of human expertise in AI-driven investing?
Human expertise is essential in AI-driven investing as it provides domain knowledge in financial analysis, economics, and geopolitics, which informs the investment process and enhances data curation and feature design.
Is there a singular algorithm that dominates AI in finance?
Contrary to the misconception, there is no singular algorithm that dominates AI in finance. Instead, a dynamic ecosystem of machine learning models is utilized to capture the complexities of financial investing effectively.
How does AI contribute to credit scoring in banking operations?
AI contributes to credit scoring in banking operations by effectively determining the creditworthiness of individuals through specialized models that offer nuanced insights and assist in decision-making based on comprehensive information.
What are the challenges in implementing AI in financial analysis?
Challenges in implementing AI in financial analysis include the need for accurate data processing, addressing data quality issues, and ensuring effective collaboration between financial experts and computer science professionals.
What is the future potential of AI in credit scoring?
The future potential of AI in credit scoring lies in mitigating risks, increasing customer base, and enhancing decision-making processes by processing more information and providing detailed insights for credit assessment.